Semantic Embeddings for Food Search Using Siamese Networks
2020 4TH INTERNATIONAL CONFERENCE ON NATURAL LANGUAGE PROCESSING AND INFORMATION RETRIEVAL, NLPIR 2020(2020)
关键词
Word embeddings,Siamese networks,FastText,Food Search
AI 理解论文
溯源树
样例
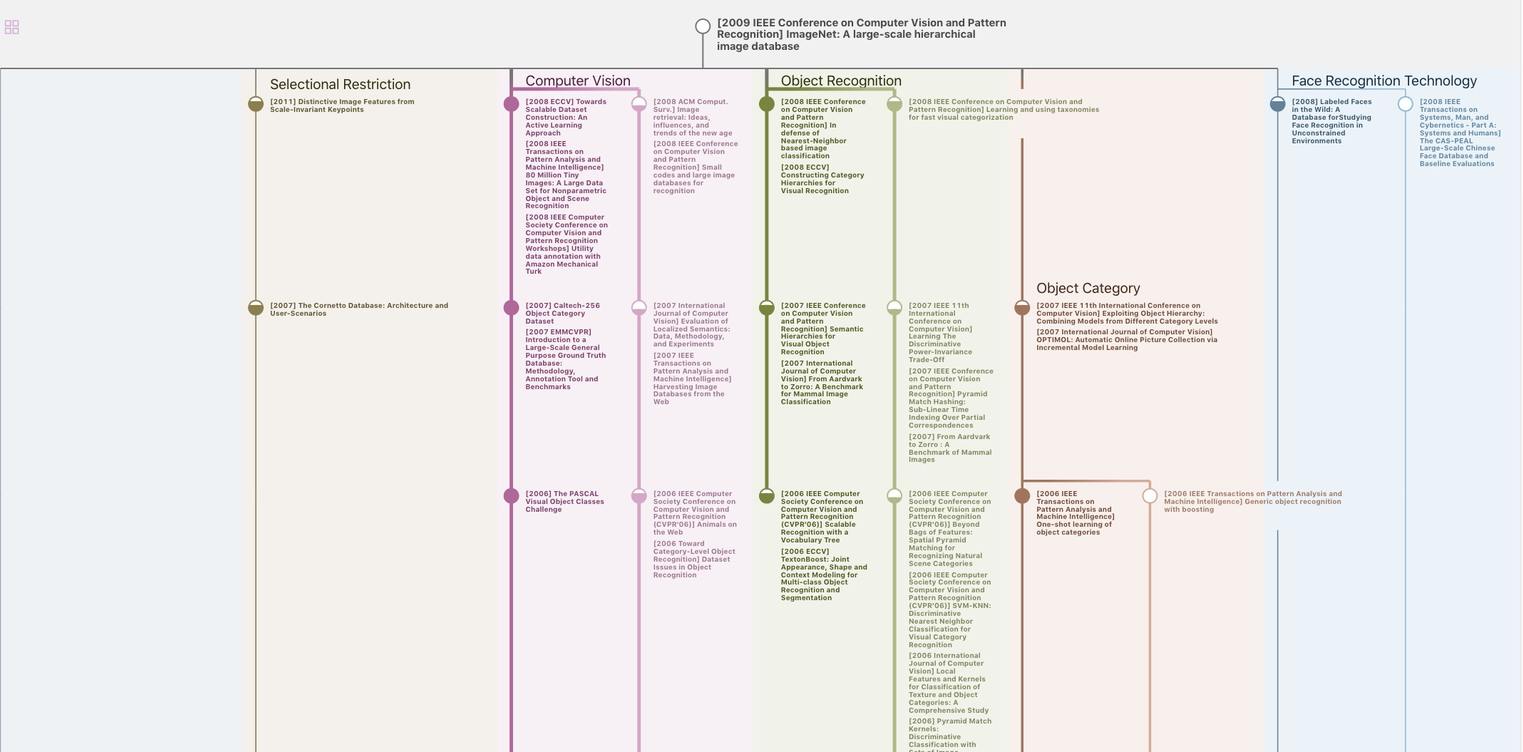
生成溯源树,研究论文发展脉络
Chat Paper
正在生成论文摘要