Handling Class Imbalance in Customer Churn Prediction in Telecom Sector Using Sampling Techniques, Bagging and Boosting Trees
2020 10th International Conference on Computer and Knowledge Engineering (ICCKE)(2020)
摘要
Customer churn is a serious problem in the telecommunications industry and occurs more often. The cost of maintaining existing customers is much lower than attracting new customers, and the literature stated that five times the cost of maintaining existing customers have to be spent on attracting new customers. In this article, we have identified customers who intend to stop using the organization's services. One of the most important problems in predicting customer churn is the imbalanced data, which has been tried to be solved and compared with different methods. The machine learning algorithms used in this paper are Decision Tree, Support Vector Machine, Multi-Layer Perceptron, Random Forest, and Gradient Boosting. Data was balanced by random over-sampling, random under-sampling and SMOTE methods. The methods of over-sampling and under-sampling had appropriate and almost similar results in terms of the area under the receiver character curve (AUC) index, the method of under-sampling has shown the better specificity, and the method over-sampling has shown the better sensitivity. Also, the performance of random forest and gradient boosting algorithms were better than other algorithms.
更多查看译文
关键词
Imbalance Data,Customer Churn,Bagging Trees,Boosting Trees,Classification,Data Balancing
AI 理解论文
溯源树
样例
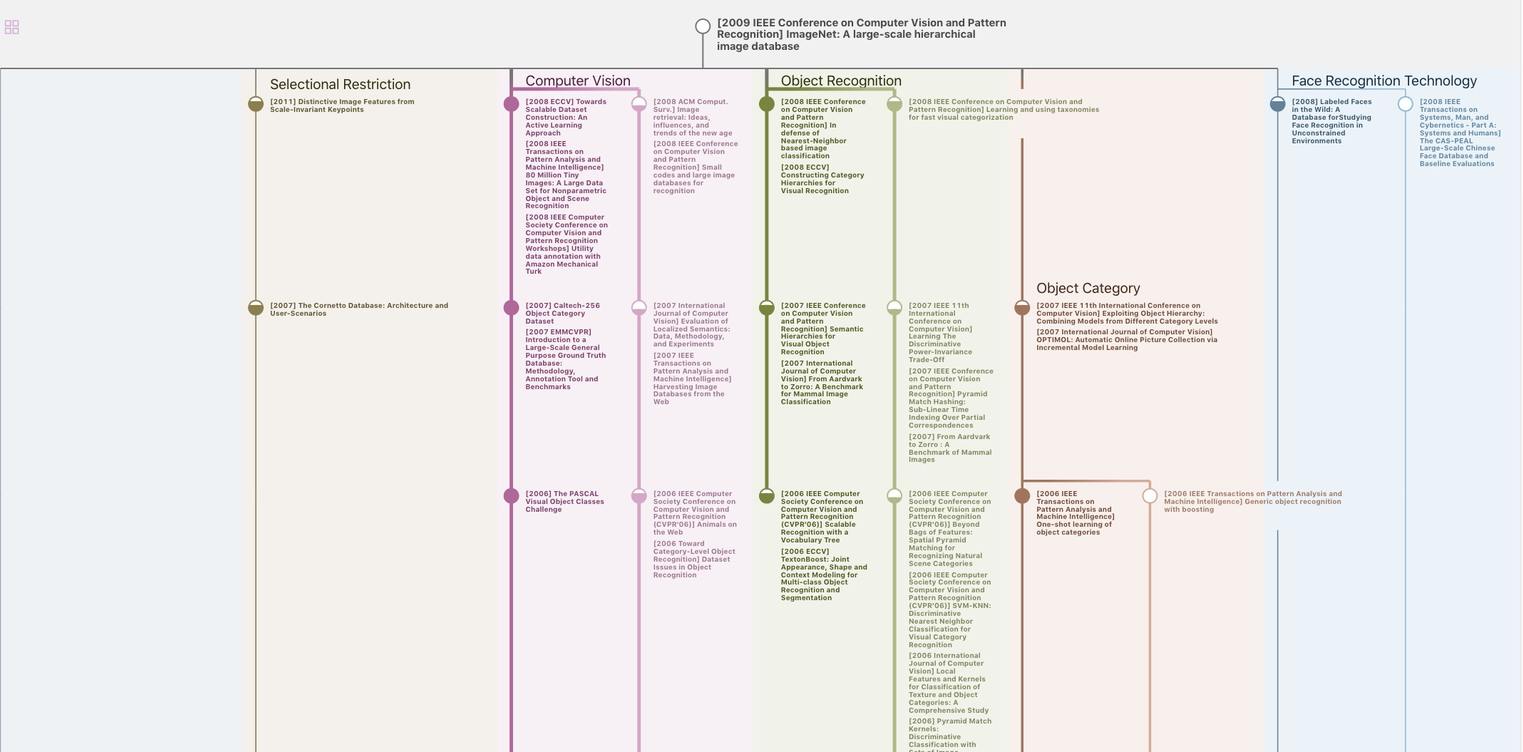
生成溯源树,研究论文发展脉络
Chat Paper
正在生成论文摘要