A Learning-based Load, PV and Energy Storage System Control for Nearly Zero Energy Building
power and energy society general meeting(2020)
摘要
Large volumes of data are generated by advanced meters and sensors in modern buildings. A learning-based control method is proposed in this paper to benefit both power system operation and end-use facilities by utilizing these big data. Different from the other control methods, this paper aims to generate accurate control policies for building loads using deep learning instead of pre-setting by customers. Firstly, the learning models predict solar power supply, fixed loads (FLs), customers’ comfort index requirement, controllable loads (CLs) and the daily driving cycles of EVs of a building based on the history data. Then, the information predicted using the learning models is applied to an optimization algorithm to minimize the deviation between the on-site PV generated energy and the actual building energy consumption by properly scheduling building loads, EVs charging cycles and Energy Storage System (ESS). The optimization algorithm aims to achieve nearly zero energy building (ZEB) and to find a highly efficient and economic management scheduling by considering customers’ comfort demand. The proposed learning strategy is developed and compared by using four different machine learning methods based on the data from Pecan Street project of past three years. The non-linear optimization problem is solved by using CPLEX solver.
更多查看译文
关键词
building energy management, deep neural network (DNN), support vector regression (SVR), gradient boosting repressor (GBR), long short-term memory (LSTM), nearly zero, energy building
AI 理解论文
溯源树
样例
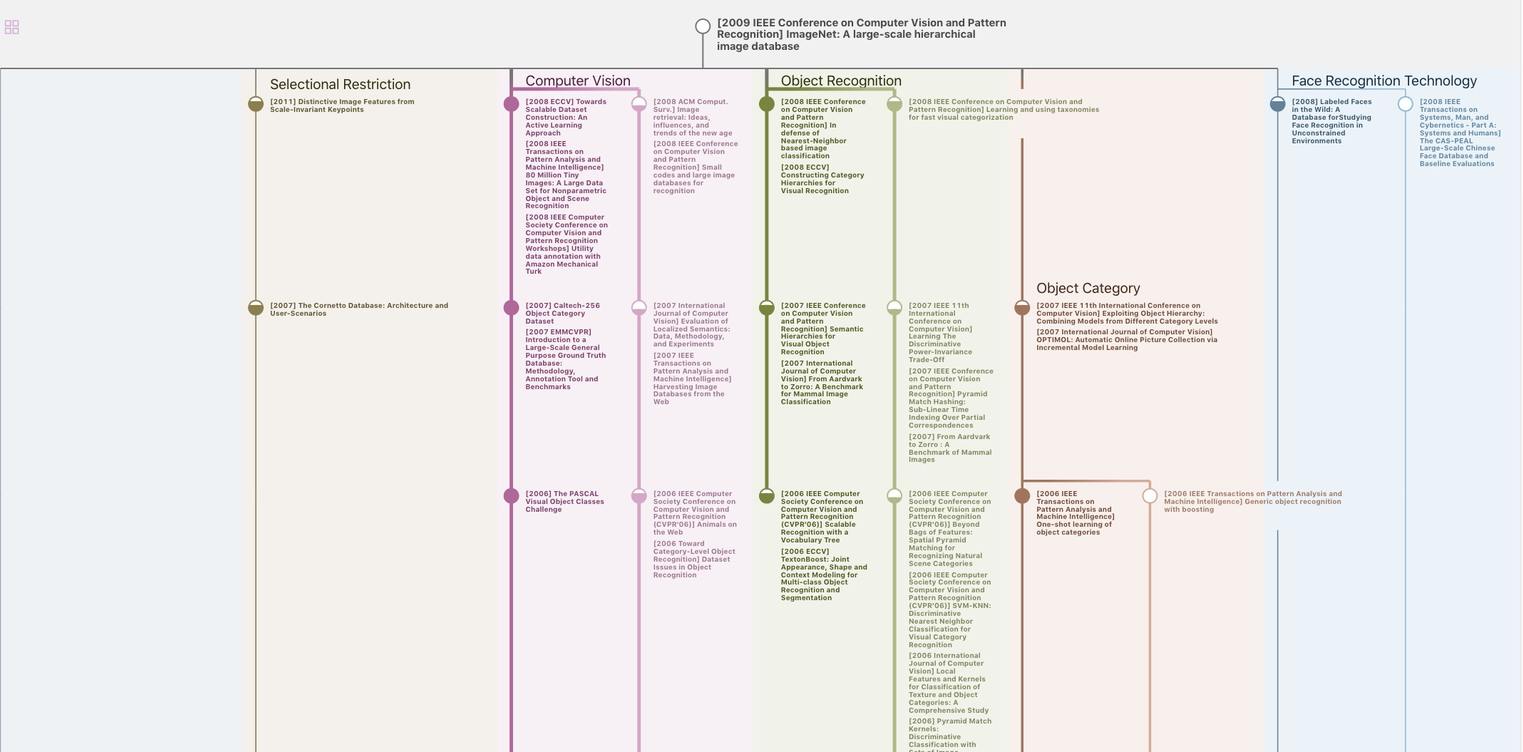
生成溯源树,研究论文发展脉络
Chat Paper
正在生成论文摘要