Predicting the Electrical Conductivity of Brine-Saturated Rocks Using Machine Learning Methods
Journal of applied geophysics(2021)
摘要
Machine learning (ML) methods are used for predicting the electrical conductivity of brine-saturated rocks. Three relevant ML methods including the extreme gradient boosting regression (XGB), the artificial neural networks (ANN) and the support vector machine regression (SVM) methods are considered and compared. The basic features such as the porosity, electrical conductivity of pore fluid as well as the normalized cation-exchange capacity of the solid phase are considered for such prediction. A relevant laboratory measurement dataset available in literature is considered to train and test the ML models. All the three considered ML methods provide very good predictive capacity on the testing set. But the XGB method is more robust and extremely fast to train comparing to the ANN method and the SVM methods. A sensitivity analysis was also realized to clarify the effect of the model parameters including the number of estimators, the learning rate and the regularization parameters. A set of optimal parameters issue from such analysis is provided for the winning XGB model. Using those optimal parameters, the XGB method predicts an average R-squared score of 0.96 for different random splitting between the training and testing sets.
更多查看译文
关键词
Rock,Electrical conductivity,Machine learning,XGBoost
AI 理解论文
溯源树
样例
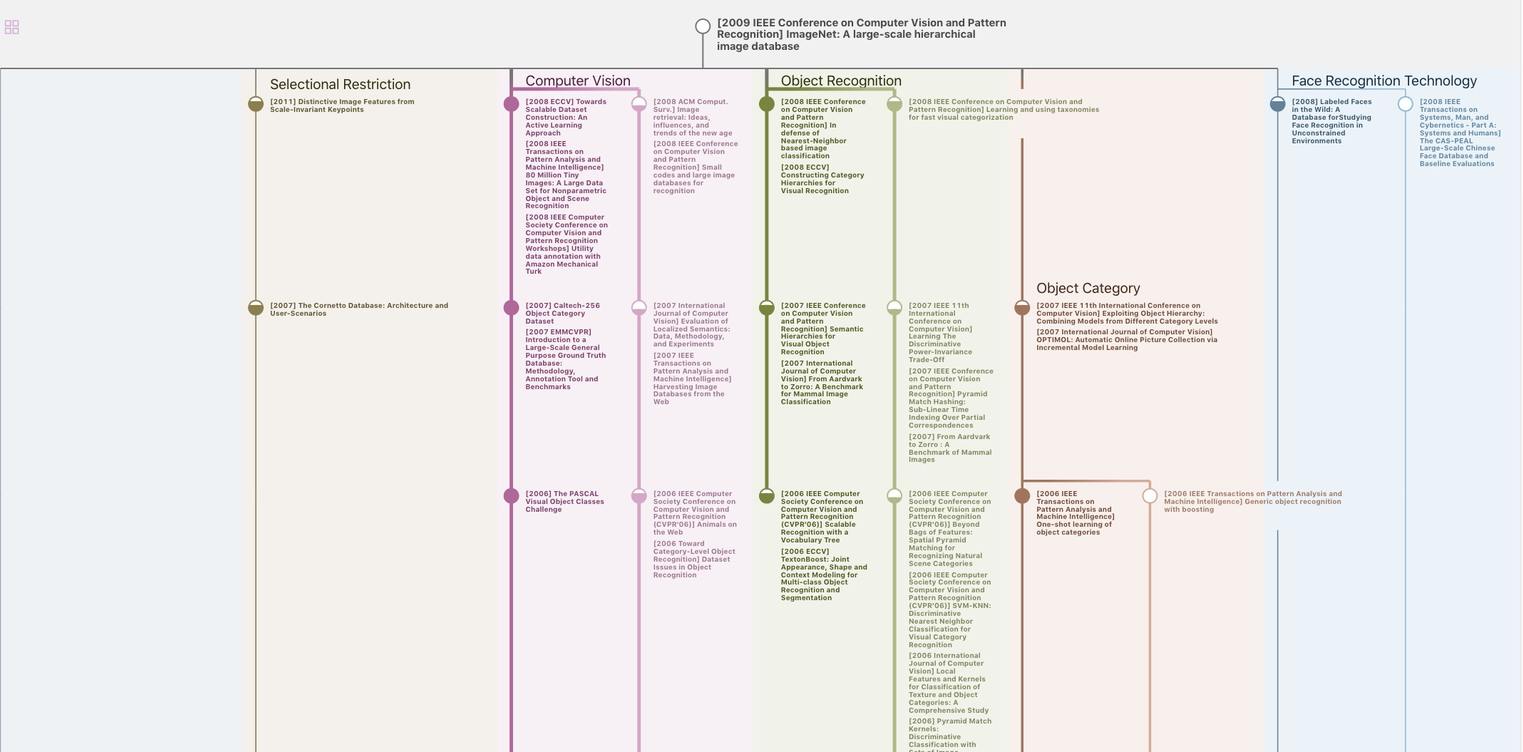
生成溯源树,研究论文发展脉络
Chat Paper
正在生成论文摘要