Adapted Single-Cell Consensus Clustering (Adasc3).
Advances in data analysis and classification(2020)
摘要
The analysis of single-cell RNA sequencing data is of great importance in health research. It challenges data scientists, but has enormous potential in the context of personalized medicine. The clustering of single cells aims to detect different subgroups of cell populations within a patient in a data-driven manner. Some comparison studies denote single-cell consensus clustering (SC3), proposed by Kiselev et al. (Nat Methods 14(5):483–486, 2017), as the best method for classifying single-cell RNA sequencing data. SC3 includes Laplacian eigenmaps and a principal component analysis (PCA). Our proposal of unsupervised adapted single-cell consensus clustering (adaSC3) suggests to replace the linear PCA by diffusion maps, a non-linear method that takes the transition of single cells into account. We investigate the performance of adaSC 3 in terms of accuracy on the data sets of the original source of SC3 as well as in a simulation study. A comparison of adaSC 3 with SC3 as well as with related algorithms based on further alternative dimension reduction techniques shows a quite convincing behavior of adaSC 3.
更多查看译文
关键词
Diffusion maps,Non-linear embedding,Single-cell consensus clustering,Simulation data,Single-cell RNA sequencing data,62H30,68U20
AI 理解论文
溯源树
样例
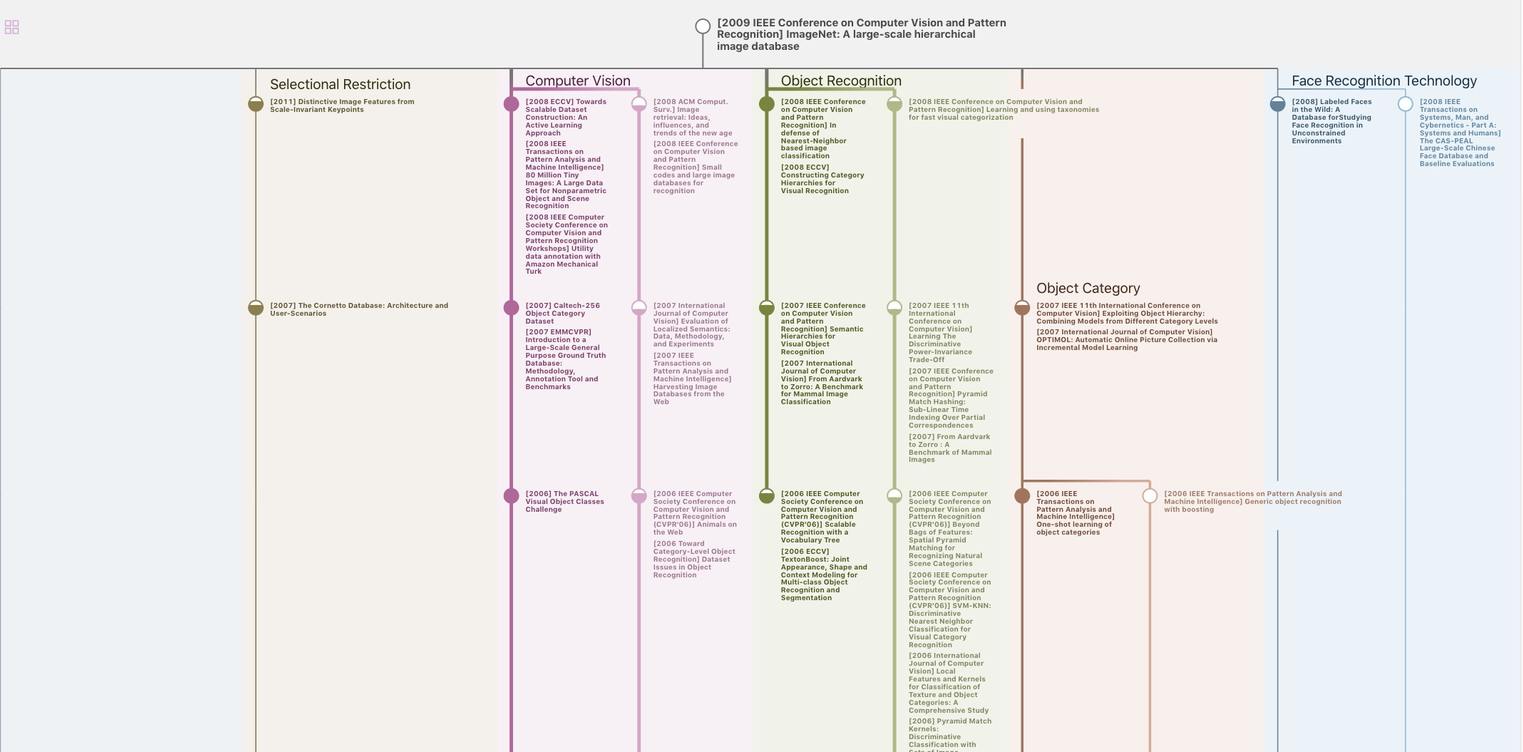
生成溯源树,研究论文发展脉络
Chat Paper
正在生成论文摘要