Domain Adaptation for Degraded Remote Scene Classification
2020 16th International Conference on Control, Automation, Robotics and Vision (ICARCV)(2020)
摘要
Remote scene classification serves a vital role in many applications. However, satellite images are often blurred and degraded due to aerosol scattering under fog, haze, and other weather conditions, reducing the image contrast and color fidelity. State-of-the-art remote sensing classification models building upon convolutional neural networks (CNNs) are mostly trained on annotated datasets of clear satellite images. When applied to blurred images, they will suffer a great degradation in performance. To address this problem, we adopt the domain adaptation algorithm TADA and propose Transferable Attention enhanced Adversarial Adaptation Network (TA
3
N), which utilizes annotated data in clear images by applying knowledge transferring from clear image domain to blurred image domain. Our TA
3
N first integrates spatial attention to focus on salient areas which are discriminative and transferable. In addition, domain discriminator and adversarial training via gradient reversal layer are used to minimize the discrepancies in extracted features from clear and degraded domains. We synthesize degraded remote scene classification dataset SSI based on FoHIS model. Experiments on degraded SSI showed that TA
3
N significantly outperforms baseline and other state-of-the-art domain adaptation methods.
更多查看译文
关键词
degraded remote scene classification,aerosol scattering,image contrast,color fidelity,convolutional neural networks,clear satellite images,domain adaptation algorithm TADA,spatial attention,domain discriminator,adversarial training,remote scene classification dataset SSI,degraded SSI,domain adaptation methods,transferable attention enhanced adversarial adaptation network,remote sensing classification models
AI 理解论文
溯源树
样例
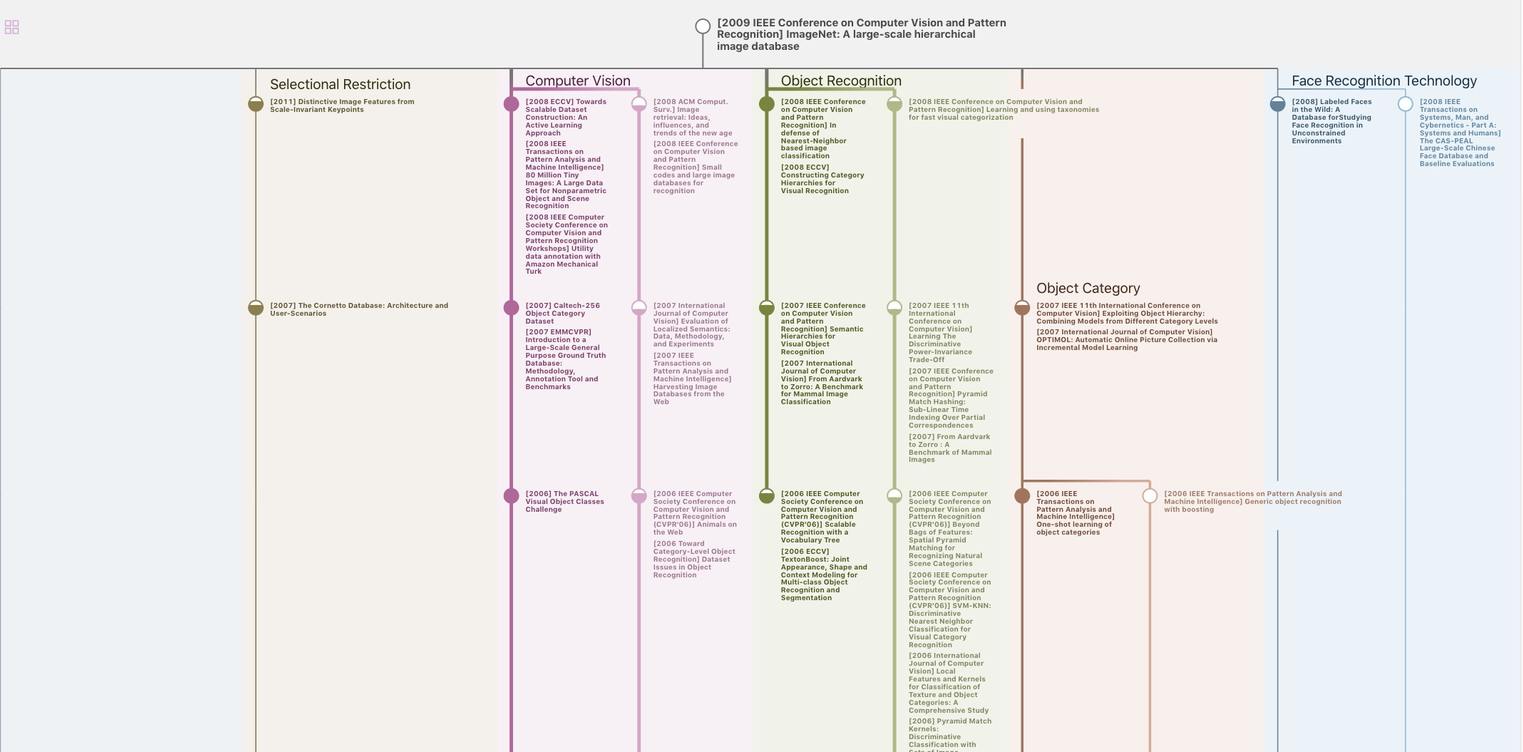
生成溯源树,研究论文发展脉络
Chat Paper
正在生成论文摘要