Deep Radar Sensor Models for Accurate and Robust Object Tracking
2020 IEEE 23RD INTERNATIONAL CONFERENCE ON INTELLIGENT TRANSPORTATION SYSTEMS (ITSC)(2020)
摘要
Object tracking is one of the key challenges for perception systems of autonomous vehicles. Recursive Bayesian state estimation can be used to obtain object tracks. Both the measurement association and the object update within such Bayesian filters rely on sensor measurement models. These models offer an approximation of the expected sensor values that can be error-prone due to a mismatch between model and reality. The discrepancy is caused by the limited descriptive capacity of measurement models since sensor measurements are highly object and situation dependent. We address this problem in a data-driven approach by using Deep Neural Networks (DNNs) to learn situation dependent sensor measurement models. In detail, the DNN acts as a virtual sensor that uses current sensor measurements to regress necessary corrections of predicted object states. It can be directly plugged into existing tracking frameworks, substituting the previously hand-modeled association and update steps during Bayesian Filtering. We apply the proposed DNN-based measurement models to the problem of vehicle tracking using radar data in an Extended Kalman Filter setup and compare it to a classical closest reflex and an L-shape measurement update model. Extensive evaluation on a real-world dataset shows that our model improves performances significantly compared to state of the art methods.
更多查看译文
关键词
situation dependent sensor measurement models,virtual sensor,current sensor measurements,predicted object states,hand-modeled association,Bayesian Filtering,DNN-based measurement models,vehicle tracking,radar data,Extended Kalman Filter setup,L-shape measurement update model,Deep radar sensor models,object tracking,perception systems,autonomous vehicles,Recursive Bayesian state estimation,measurement association,Bayesian filters,expected sensor values,data-driven approach,Deep Neural Networks,real-world dataset
AI 理解论文
溯源树
样例
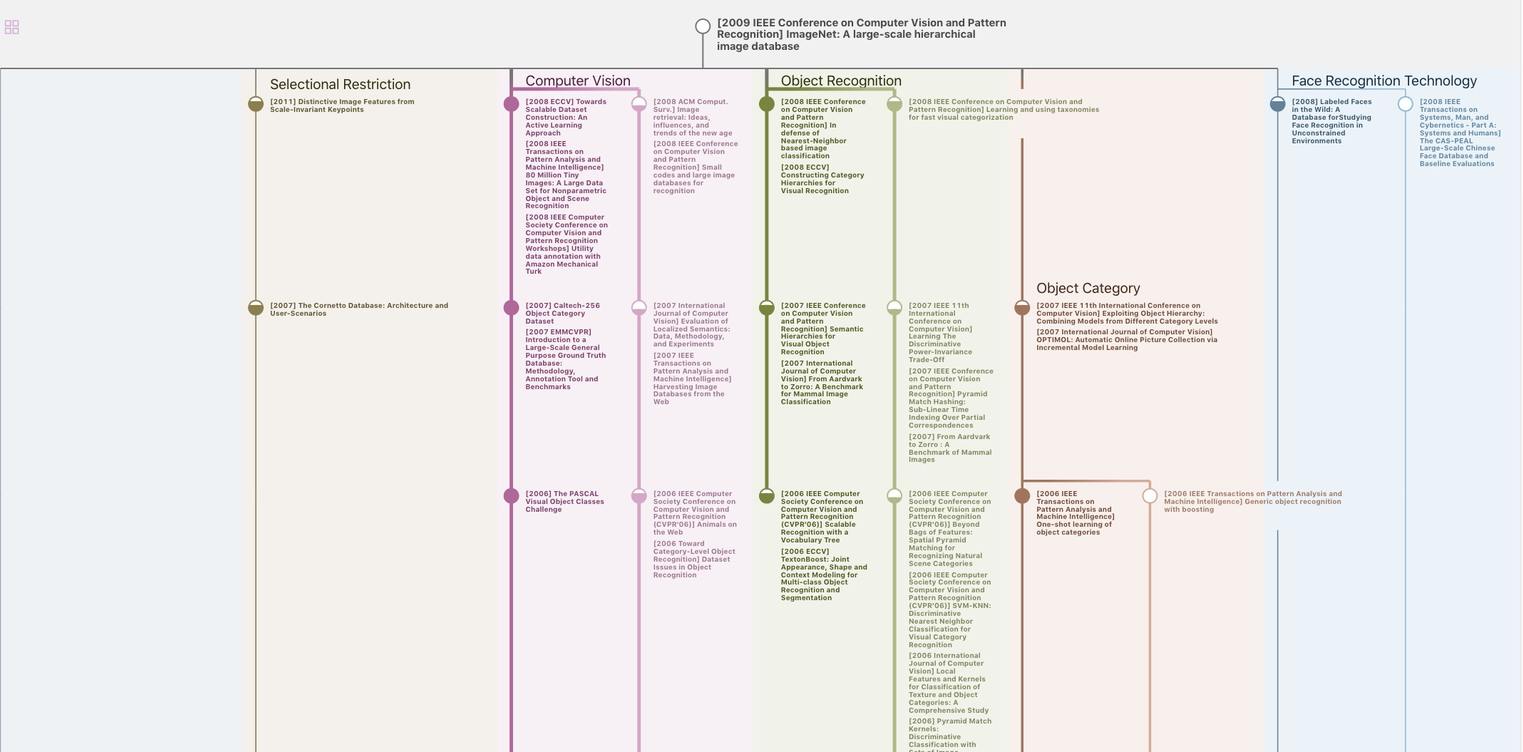
生成溯源树,研究论文发展脉络
Chat Paper
正在生成论文摘要