Memory Requirement Reduction of Deep Neural Networks for Field Programmable Gate Arrays Using Low-Bit Quantization of Parameters
28TH EUROPEAN SIGNAL PROCESSING CONFERENCE (EUSIPCO 2020)(2021)
摘要
Effective employment of deep neural networks (DNNs) in mobile devices and embedded systems, like field programmable gate arrays, is hampered by requirements for memory and computational power. In this paper we propose a method that employs a non-uniform fixed-point quantization and a virtual bit shift (VBS) to improve the accuracy of the quantization of the DNN weights. We evaluate our method in a speech enhancement application, where a fully connected DNN is used to predict the clean speech spectrum from the input noisy speech spectrum. A DNN is optimized, its memory requirement is calculated, and its performance is evaluated using the short-time objective intelligibility (STOI) metric. The application of the low-bit quantization leads to a 50% reduction of the DNN memory requirement while the STOI performance drops only by 2.7%.
更多查看译文
关键词
neural network quantization,memory footprint reduction,FPGA,hardware accelerators
AI 理解论文
溯源树
样例
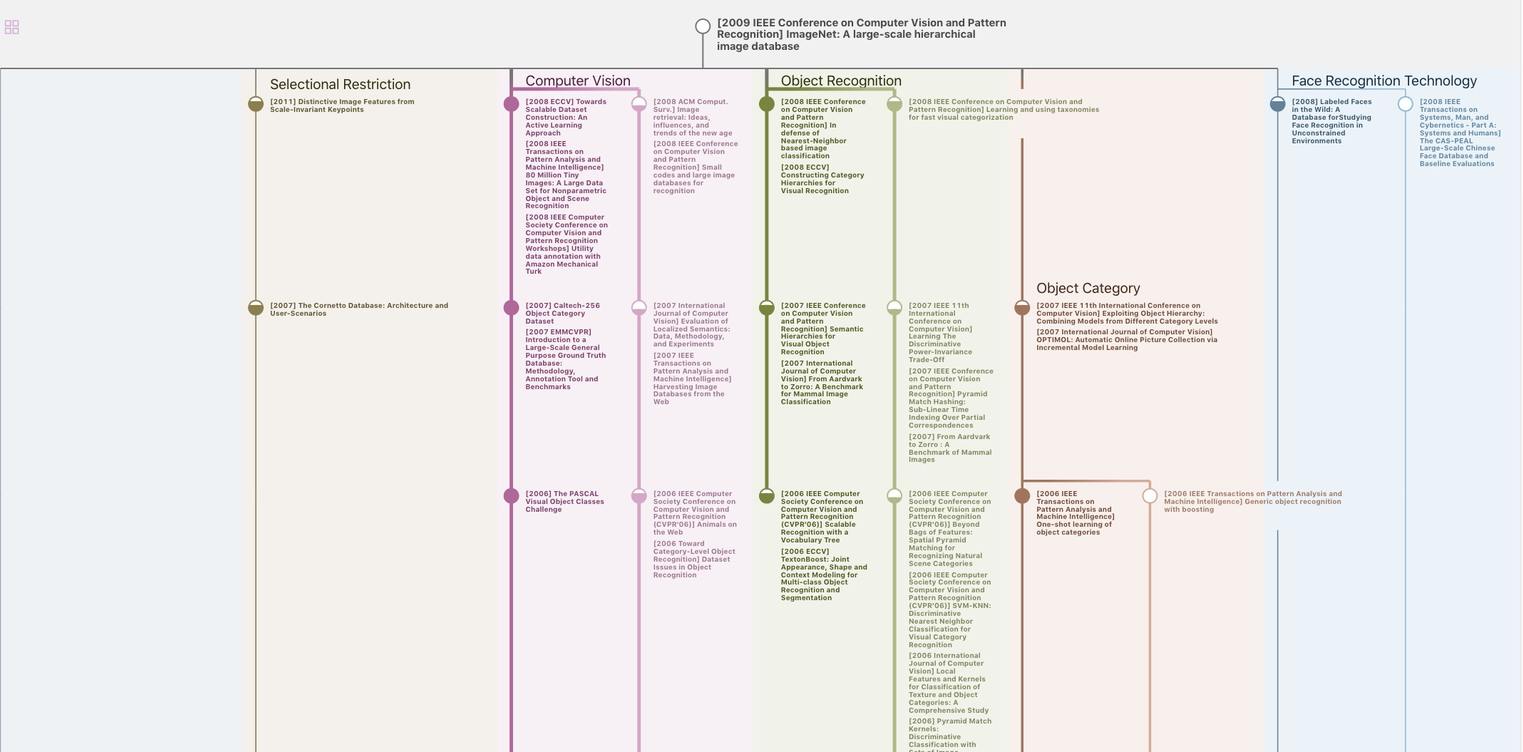
生成溯源树,研究论文发展脉络
Chat Paper
正在生成论文摘要