Alpha Shape Analysis (ASA) Framework for Post- Clustering Property Determination in Atom Probe Tomographic Data
MICROSCOPY AND MICROANALYSIS(2021)
摘要
While application of clustering algorithms to atom probe tomography data have enabled quantification of solute clusters in terms of number density, size, and subcomposition there exist other properties (e.g., volume, surface area, and composition) that are better determined by defining an interface between the cluster and the surrounding matrix. The limitation in composition results from an ion selection step where the expected matrix ion types are omitted from the cluster search algorithm to enhance the contrast between the matrix and cluster and to reduce the complexity of the search. Previously, composition determination within solute clusters has utilized a secondary envelopment and erosion step on top of conventional methods such as maximum separation. In this work, we present a novel stochastic method that combines the particle identification fidelity of a conventional clustering algorithm with the analytical flexibility of mesh-based approaches through the generation of alpha shapes for each identified cluster. The corresponding mesh accounts for concave components of the clusters and determines the volume and surface area of the clusters; additionally, the mesh boundary is utilized to update the total composition according to the internal ions.
更多查看译文
关键词
alpha shapes,atom probe tomography,cluster-analysis,surface reconstruction
AI 理解论文
溯源树
样例
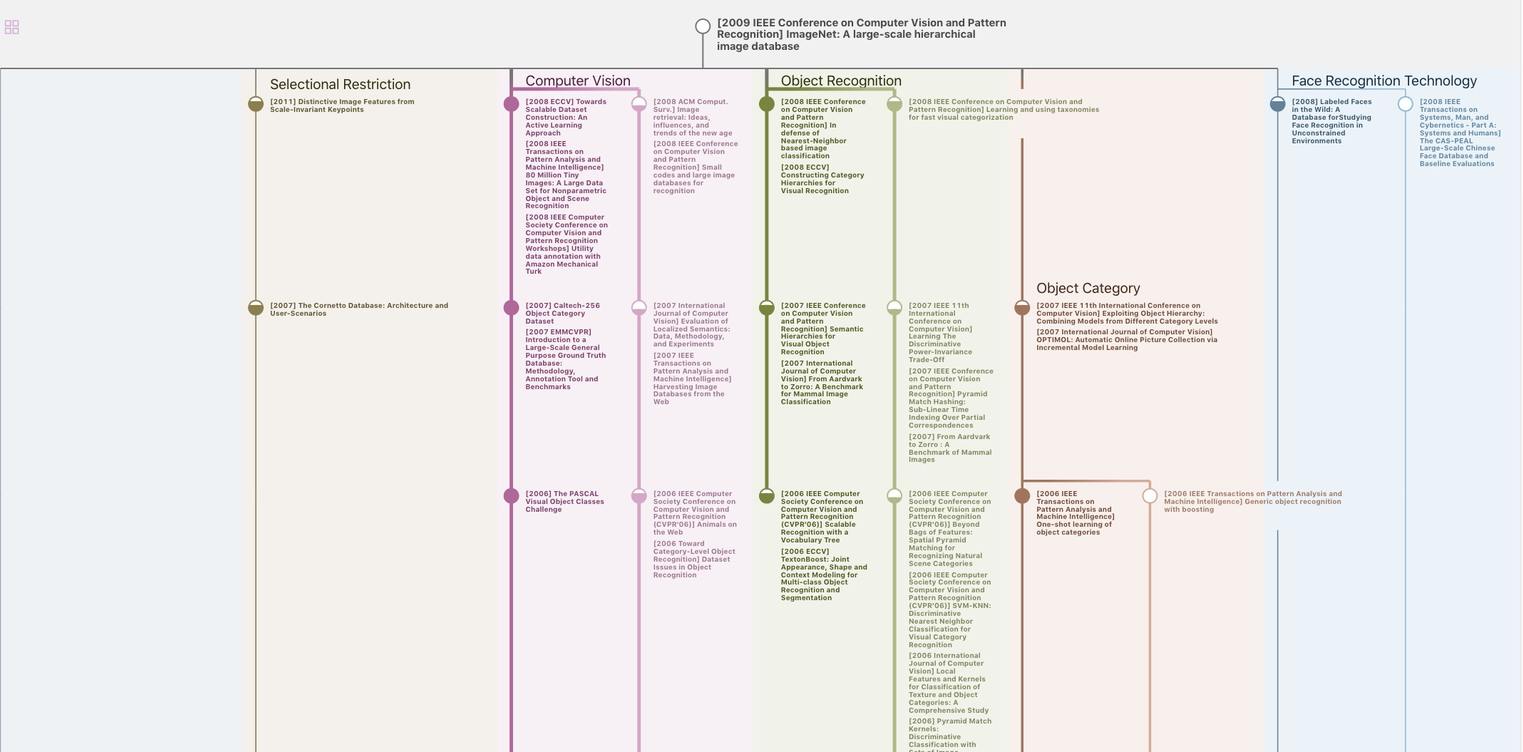
生成溯源树,研究论文发展脉络
Chat Paper
正在生成论文摘要