Dynamic Attention Aggregation With Bert For Neural Machine Translation
2020 INTERNATIONAL JOINT CONFERENCE ON NEURAL NETWORKS (IJCNN)(2020)
摘要
The recently proposed BERT has demonstrated great power in various natural language processing tasks. However, the model does not perform effectively on cross-lingual tasks, especially on machine translation. In this work, we propose three methods to introduce pre-trained BERT into neural machine translation without fine-tuning. Our approach consists of a) a linear-attention aggregation that leverages a parameter matrix to capture the key knowledge of BERT, b) a self-attention aggregation which aims to learn what is vital for input and output, and c) a switch-gate aggregation to dynamically control the balance of the information flowing from the pre-trained BERT or the NMT model. We conduct experiments on several translation benchmarks and substantially improve over 2 BELU points on the IWSLT'14 English - German task with switch-gate aggregation method compared to a strong baseline, while our proposed model also performs remarkably on the other tasks.
更多查看译文
关键词
neural machine translation,linear-attention aggregation,self-attention aggregation,pretrained BERT,NMT model,translation benchmarks,German task,switch-gate aggregation method,dynamic attention aggregation,natural language processing tasks,cross-lingual tasks
AI 理解论文
溯源树
样例
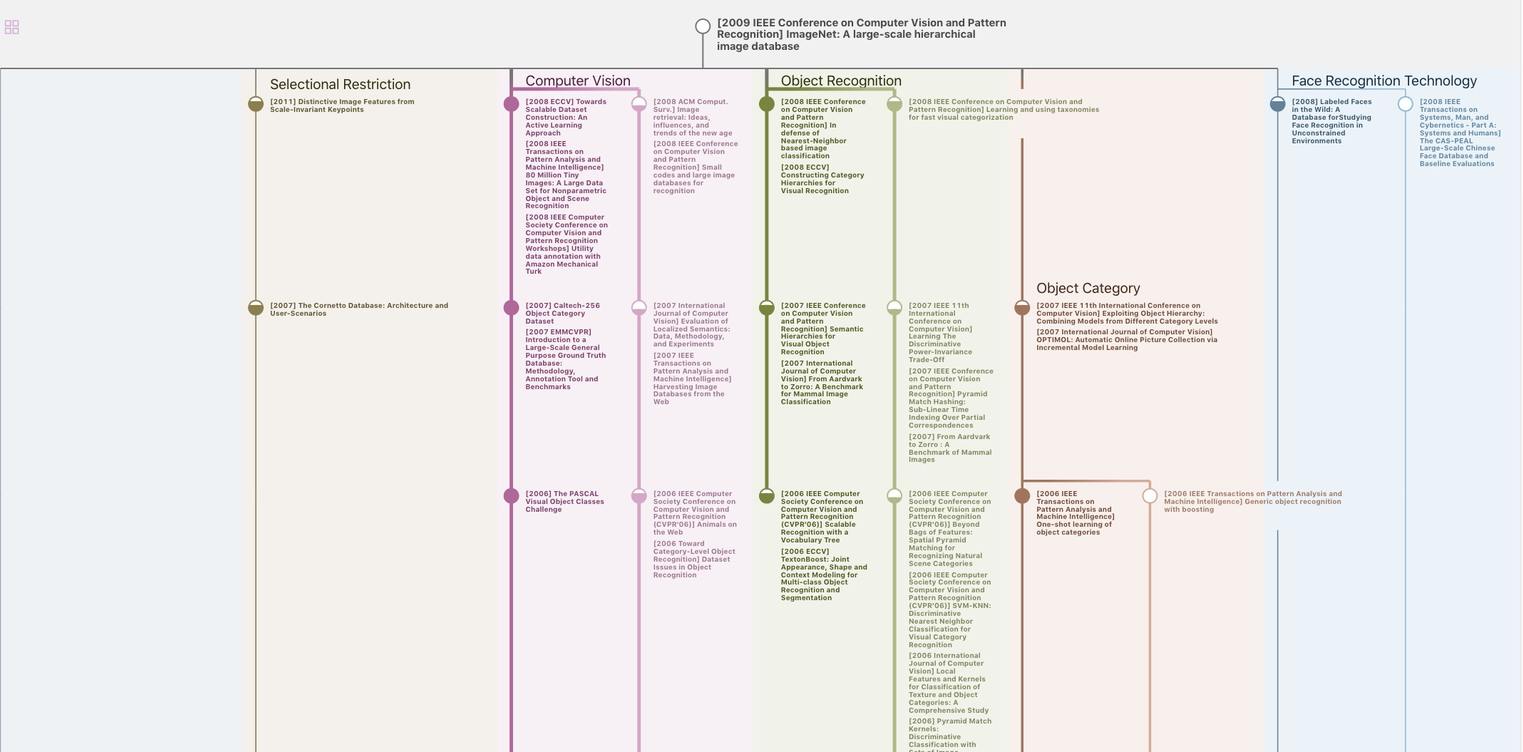
生成溯源树,研究论文发展脉络
Chat Paper
正在生成论文摘要