Improved-StoryGAN for sequential images visualization
Journal of Visual Communication and Image Representation(2020)
摘要
Story visualization is a novel and challenging topic that intersects computer vision and natural language processing, which needs to generate sequential images based on a story. It is related to text-to-image generation and video generation. Apart from ensuring the quality of the results, the synthesized images of story visualization are supposed to be consistent with each other and reflect the input story. In order to improve the performance of generated sequential images, we have developed the baseline model StoryGAN. Firstly, we use Dilated Convolution in the discriminators to expand the receptive field of the convolution kernel in the feature maps, thus enhancing the quality of the generated sequential images. In addition, Weighted Activation Degree (WAD) is introduced in the discriminators to provide a robust evaluation in view of similarity between the generated images and the target story, which results in enhancement on the consistency between the generated images and the target story. Last but not least, Bi-GRU stores the historical and future information of each sentence to effectively extract the textual features. What’s more, in order to make full use of the features of the long story features, Gated Convolution is used to replace the original MLP in the Initial State Encoder to improve the consistence between the generated sequential images. Experimental results and visual sequential images demonstrate the outperformance of the model we develop, compared with the other models.
更多查看译文
关键词
Story visualization,Weighted Activation Degree (WAD),Dilated Convolution,Gated Convolution
AI 理解论文
溯源树
样例
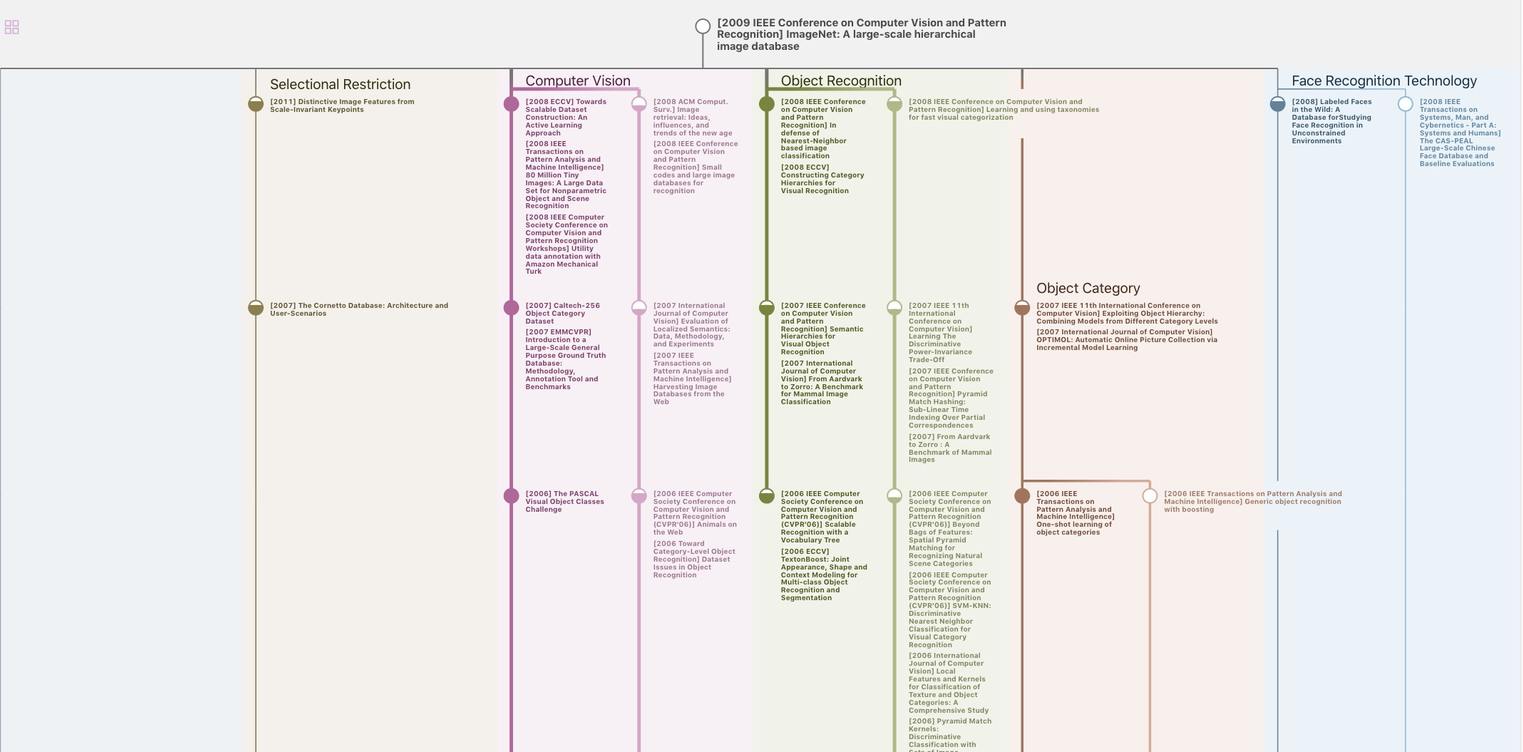
生成溯源树,研究论文发展脉络
Chat Paper
正在生成论文摘要