Bayesian Inference Of 1d Activity Profiles From Segmented Gamma Scanning Of A Heterogeneous Radioactive Waste Drum
APPLIED RADIATION AND ISOTOPES(2021)
摘要
We present a Bayesian approach to probabilistically infer vertical activity profiles within a radioactive waste drum from segmented gamma scanning (SGS) measurements. Our approach resorts to Markov chain Monte Carlo (MCMC) sampling using the state-of-the-art Hamiltonian Monte Carlo (HMC) technique and accounts for two important sources of uncertainty: the measurement uncertainty and the uncertainty in the source distribution within the drum. In addition, our efficiency model simulates the contributions of all considered segments to each count measurement. Our approach is first demonstrated with a synthetic example, after which it is used to resolve the vertical activity distribution of 5 nuclides in a real waste package.
更多查看译文
关键词
Segmented gamma scanning (SGS), Markov chain Monte Carlo (MCMC), Source distribution uncertainty, Uncertainty quantification, Spatially-distributed inference
AI 理解论文
溯源树
样例
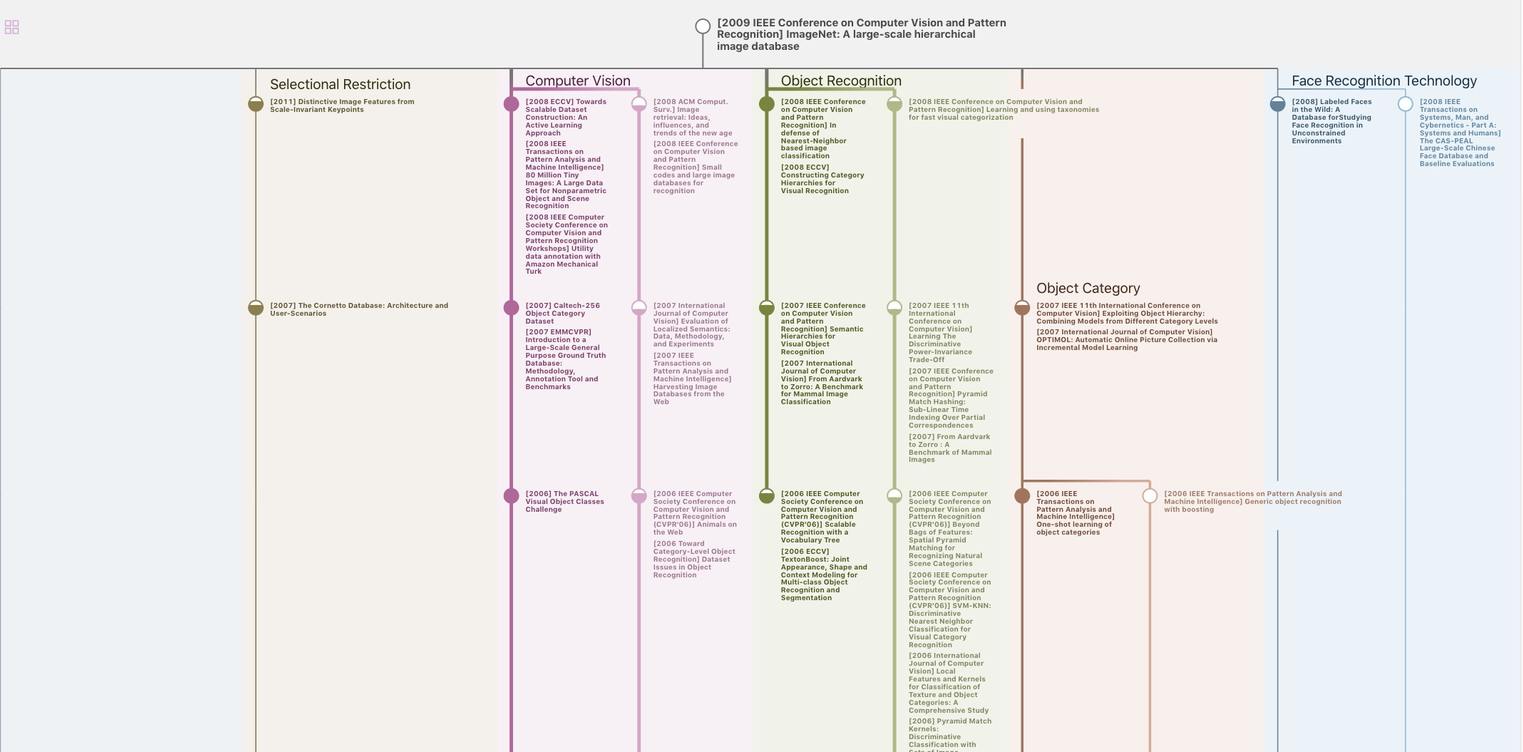
生成溯源树,研究论文发展脉络
Chat Paper
正在生成论文摘要