Real-time Structural Health Monitoring of Nonlinear Hysteretic Structures Using a Fast and Slow Dynamics Separation Method.
IFAC JOURNAL OF SYSTEMS AND CONTROL(2021)
摘要
Structural health monitoring (SHM) is the process of identification, localisation, and quantification of structural damage due to external loads, such as an earthquake. SHM results simplify and automate typical visual or localised experimental approaches and enable more informed structural safety assessment and post-event retrofit. On-line SHM is of particular interest for rapid safety assessment by owners and civil defence authorities, and can also inform active control systems to further avoid or mitigate damage during an event. This significant advantage over off-line SHM has drawn many researchers to on-line or real-time methods. This paper presents an on-line SHM algorithm for model-based identification and monitoring of non-linear hysteretic structures. It separates fast and slow dynamics and uses robust piecewise least squares fitting over different time intervals to identify key structural parameters over time, including stiffness, damping, and the well-accepted Bouc–Wen hysteretic model parameters, which are directly related to well-recognised damage metrics. Proof-of-method simulations of a realistic nonlinear case study structure, subjected to a suite of 20 different ground motions, show the algorithm accurately identifies structural parameters to within 2.7% and 4.3% of the actual as-modelled values for stiffness and damping, respectively. Results for a range of major changes (damage) in structural parameters show the algorithm performs well in tracking these changes in real-time. Overall, the results present an accurate, real-time, model-based SHM method for highly nonlinear structures.
更多查看译文
关键词
Structural health monitoring (SHM),Structural identification,Damage detection,Nonlinear hysteretic structures,The Bouc–Wen model,Fast and slow dynamics separation
AI 理解论文
溯源树
样例
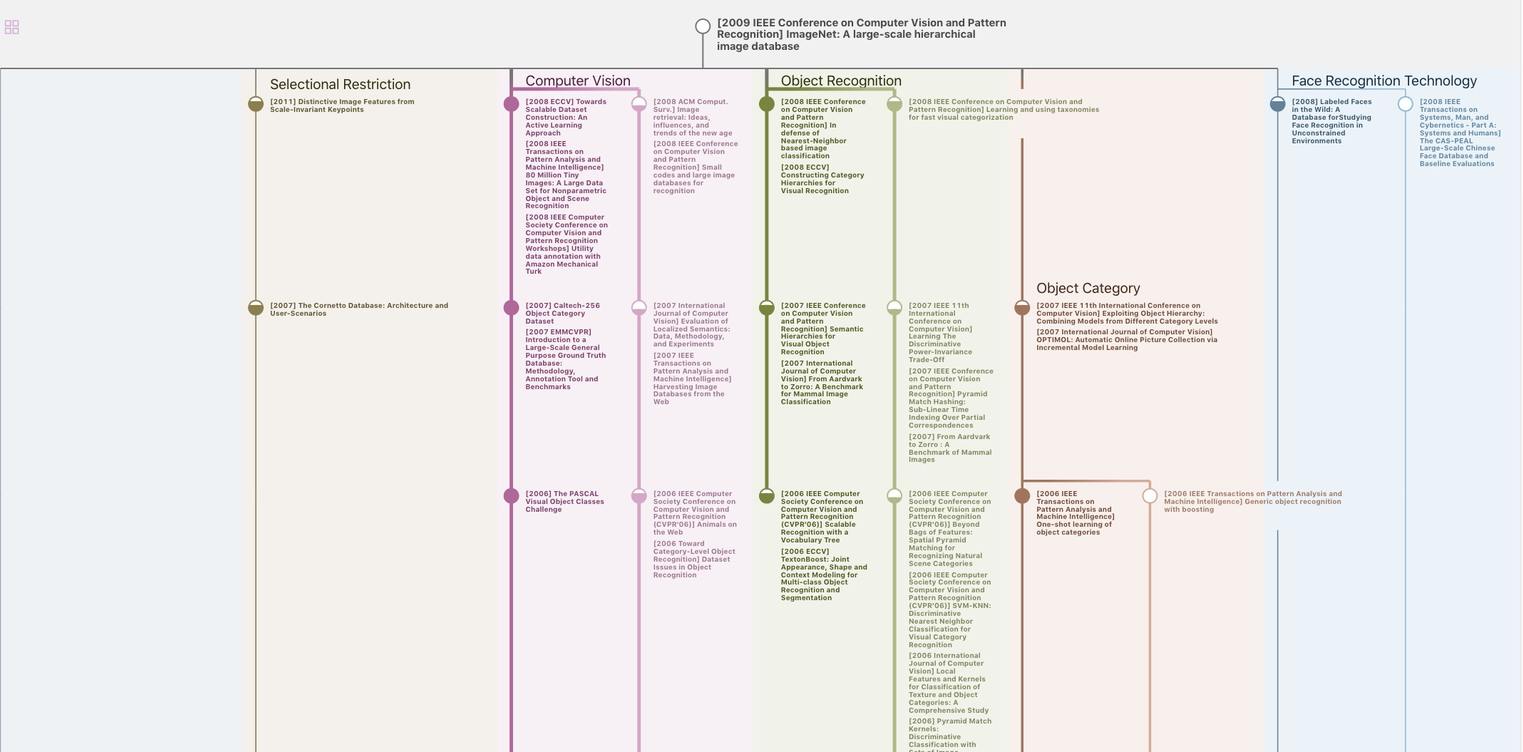
生成溯源树,研究论文发展脉络
Chat Paper
正在生成论文摘要