Gaussian Process-Driven, Nested Experimental Co-Design: Theoretical Framework and Application to an Airborne Wind Energy System
JOURNAL OF DYNAMIC SYSTEMS MEASUREMENT AND CONTROL-TRANSACTIONS OF THE ASME(2021)
Abstract
This paper presents and experimentally evaluates a nested combined plant and controller optimization (co-design) strategy that is applicable to complex systems that require extensive simulations or experiments to evaluate performance. The proposed implementation leverages principles from Gaussian process (GP) modeling to simultaneously characterize performance and uncertainty over the design space within each loop of the co-design framework. Specifically, the outer loop uses a GP model and batch Bayesian optimization to generate a batch of candidate plant designs. The inner loop utilizes recursive GP modeling and a statistically driven adaptation procedure to optimize control parameters for each candidate plant design in real-time, during each experiment. The characterizations of uncertainty made available through the GP models are used to reduce both the plant and control parameter design space as the process proceeds, and the optimization process is terminated once sufficient design space reduction has been achieved. The process is validated in this work on a lab-scale experimental platform for characterizing the flight dynamics and control of an airborne wind energy (AWE) system. The proposed co-design process converges to a design space that is less than 8% of the original design space and results in more than a 50% increase in performance.
MoreTranslated text
Key words
wind energy,process-driven,co-design
AI Read Science
Must-Reading Tree
Example
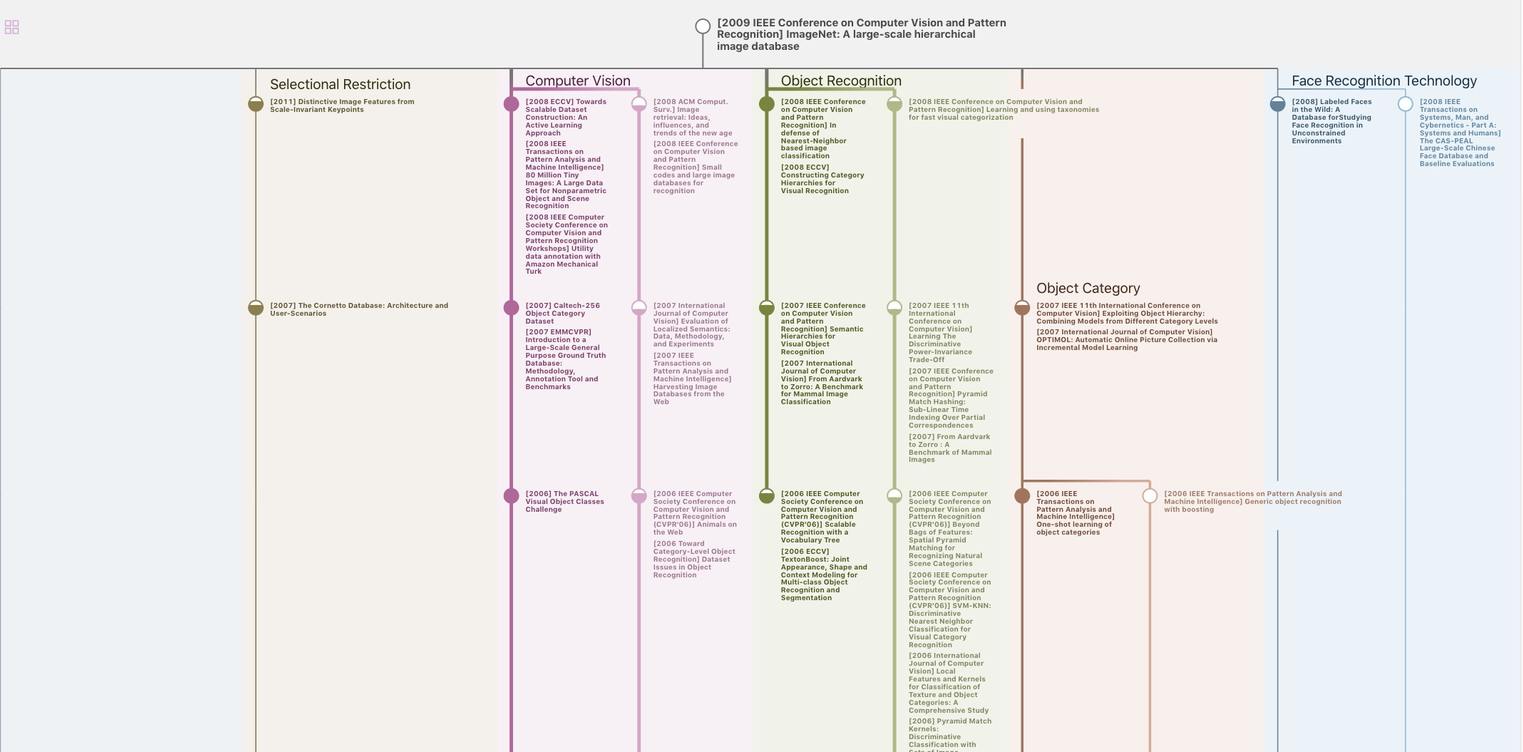
Generate MRT to find the research sequence of this paper
Chat Paper
Summary is being generated by the instructions you defined