Real-Time Object Navigation With Deep Neural Networks And Hierarchical Reinforcement Learning
IEEE ACCESS(2020)
摘要
In the last years, deep learning and reinforcement learning methods have significantly improved mobile robots in such fields as perception, navigation, and planning. But there are still gaps in applying these methods to real robots due to the low computational efficiency of recent neural network architectures and their poor adaptability to robotic experiments realities. In this article, we consider an important task in mobile robotics - navigation to an object using an RGB-D camera. We develop a new neural network framework for robot control that is fast and resistant to possible noise in sensors and actuators. We propose an original integration of semantic segmentation, mapping, localization, and reinforcement learning methods to improve the effectiveness of exploring the environment, finding the desired object, and quickly navigating to it. We created a new HISNav dataset based on the Habitat virtual environment, which allowed us to use simulation experiments to pre-train the model and then upload it to a real robot. Our architecture is adapted to work in a real-time environment and fully implements modern trends in this area.
更多查看译文
关键词
Neural networks,Navigation,Real-time systems,Task analysis,Reinforcement learning,Simultaneous localization and mapping,Indoor navigation,cognitive robotics,object segmentation,neural networks,intelligent agents,real-time systems,robot learning
AI 理解论文
溯源树
样例
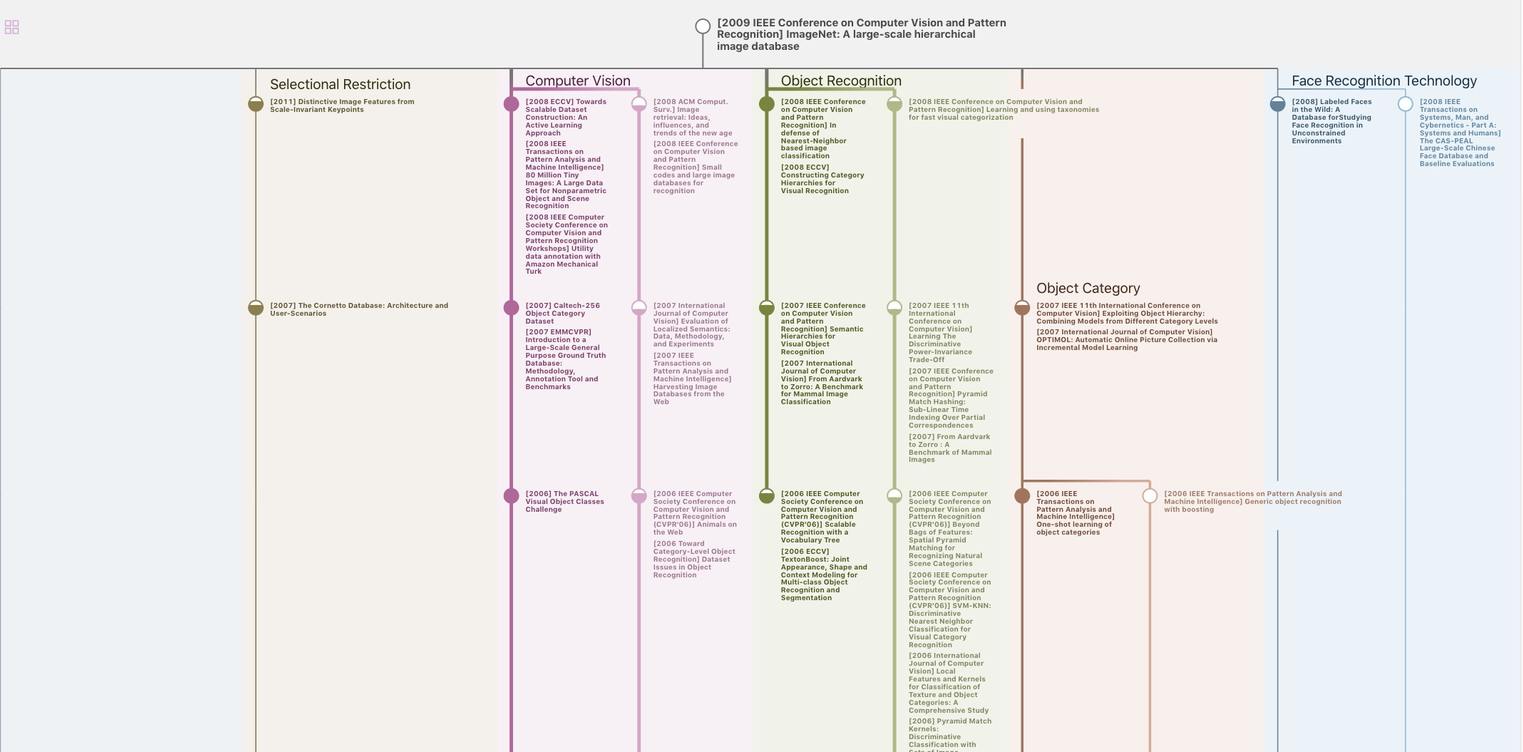
生成溯源树,研究论文发展脉络
Chat Paper
正在生成论文摘要