Clinically Interpretable Predictions of Patient-Reported Adverse Events (PRO-CTCAE) for Prostate Cancer Utilizing Artificial Neural Networks
International journal of radiation oncology, biology, physics(2020)
摘要
Patient reported toxicities (PROs) correlate clinically with patient adverse events. We evaluate the predictive power of artificial neural networks (ANN) using clinical, treatment and dosimetric data (DVH), to predict adverse events (AEs) assessed by the Patient-Reported Outcomes version of the Common Terminology Criteria for Adverse Events (PRO-CTCAETM) in prostate cancer patients. From 2016-2018, 252 patients were treated with curative intent radiotherapy (RT) for localized prostate cancer in our prospective IRB approved registry. PRO-CTCAE questionnaires were administered at baseline, end-of-treatment, 3, 6, 12 months, then annually. Patients were curatively treated for primary prostate cancer and completed baseline and at least one post-RT questionnaire. Patient-reported treatment-related AEs are defined as a “Severe/Frequent” or “Very Severe/Constantly” for any PRO-CTCAE (prostate specific or overall quality-of-life) item that is worse than baseline. We synthesized artificial data from the original data, and then tested it for interval validity and external consistency with the original data set. ANN algorithms were constructed utilizing clinical, treatment, and DVH data to predict patient-reported AEs with local interpretable model-agnostic explanations (LIME) to provide clinical interpretability. Median follow-up was 20 months (range: 2.5–40 months) with a median pre-RT PSA of 6.12 (1.3–129), 6.6% non-white, median T Stage T1c (T1b–T3b), median Gleason Score of 7 (3+4, range 6-10), and 99% N0. The median prescribed dose was 70 Gy (38–79.2) in 5 to 44 fractions. Standard fractionation (1.8-2Gy) was used in 120 patients (48.2%), moderate hypofractionation (2-3 Gy) in 87 patients (35.1%), and extreme hypofractionation (>6Gy) in 42 patients (16.7%). Baseline-adjusted patient-reported AE rates of “Severe” or worse were 38%, while “Very Severe” was 19%. Patients reporting “Severe” or worse were accurately predicted 68.4% (95% CI: 66.6%, 69.3%) by the ANN model. LIME demonstrated that patients with: Non-white race, higher number of biopsy cores taken, higher pre-RT PSA, higher CTV volume, higher baseline ECOG, and BED V51 Gy > 14.376% were at higher risk for developing a “Severe” or worse PRO-CTCAE score greater than their respective pre-RT score. Employing clinical, treatment and DVH data, ANN can predict patient reported toxicities with high validity and confidence. We have shown here that we can not only predict changes in PRO-CTCAE for prostate cancer patients, but also predict with narrow confidence intervals patients at high risk to develop patient reported toxicity months in advance of the event.
更多查看译文
AI 理解论文
溯源树
样例
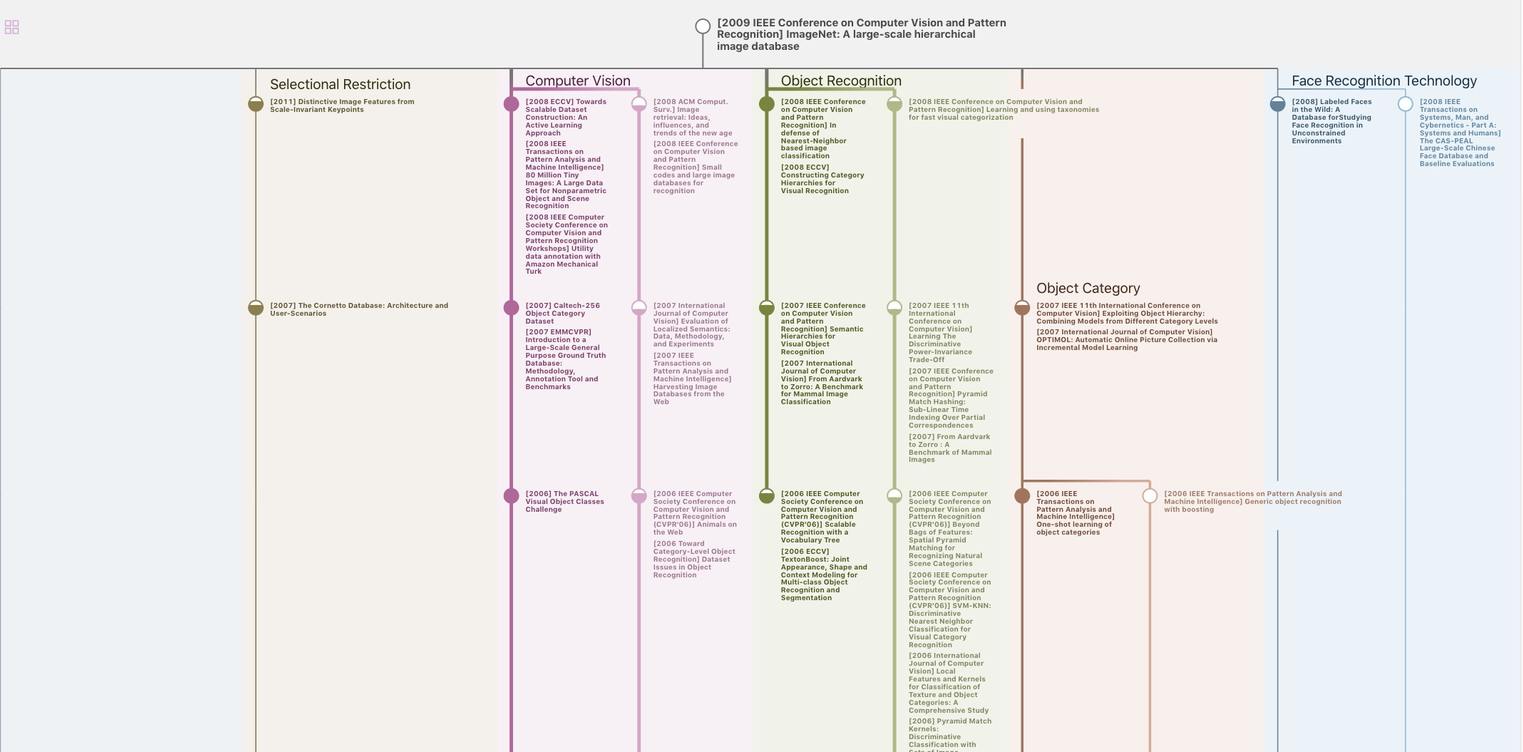
生成溯源树,研究论文发展脉络
Chat Paper
正在生成论文摘要