Application Of Machine Learning Tool To Predict The Porosity Of Clastic Depositional System, Indus Basin, Pakistan
JOURNAL OF PETROLEUM SCIENCE AND ENGINEERING(2021)
摘要
Porosity is one of the key factors of a reservoir system that is typically distributed in a spatially non-uniform and non-linear manner. Nevertheless, spatial distribution of porosity in stratigraphic traps (channel sand bodies) is challenging due to the variations in depositional environments, shale intercalation, and repetition of textural changes in the pore sizes. This paper presents a strategy of joint inversion that combines support vector machine (SVM) and particle swarm optimization (PSO) algorithms to predict the spatial distribution of porosity using well logs and seismic data. In addition, Gaussian simulation algorithms (sequential Gaussian and Gaussian indicator simulations) were used to predict lithology and porosity distribution using wireline logs and core data. Rock physics modeling was performed to bridge the gap between the elastic and petrophysical properties for seismic-inversion based reservoir characterization. The results show that the joint inversion strategy leads to the most stable prediction of AI and porosity distribution in the lower Goru heterogeneous reservoir of Sawan Gas Field, Pakistan. The calibration of the individual spatial distribution of lithology and porosity from wireline logs data using Gaussian simulation algorithms and post-stack seismic inversion using SVM and PSO; revealed favorable matching. In fact, the spatial pattern of low AI corresponds with the high porosity and sandstone lithofacies. The study results provide valuable insights for identifying the sweet spots in the heterogeneous lower Goru clastic packages of Sawan Gas Field through quantitative analysis of rock physics, petrophysics, seismic inversion, and fuzzy optimization. The proposed joint inversion strategy may be considered useful for the prediction of porosity in similar context of extremely heterogeneous stratigraphic traps in other basins of the world.
更多查看译文
关键词
Seismic inversion, Support vector machine, Rock physics, Porosity, Particle swarm optimization, Sawan gas field
AI 理解论文
溯源树
样例
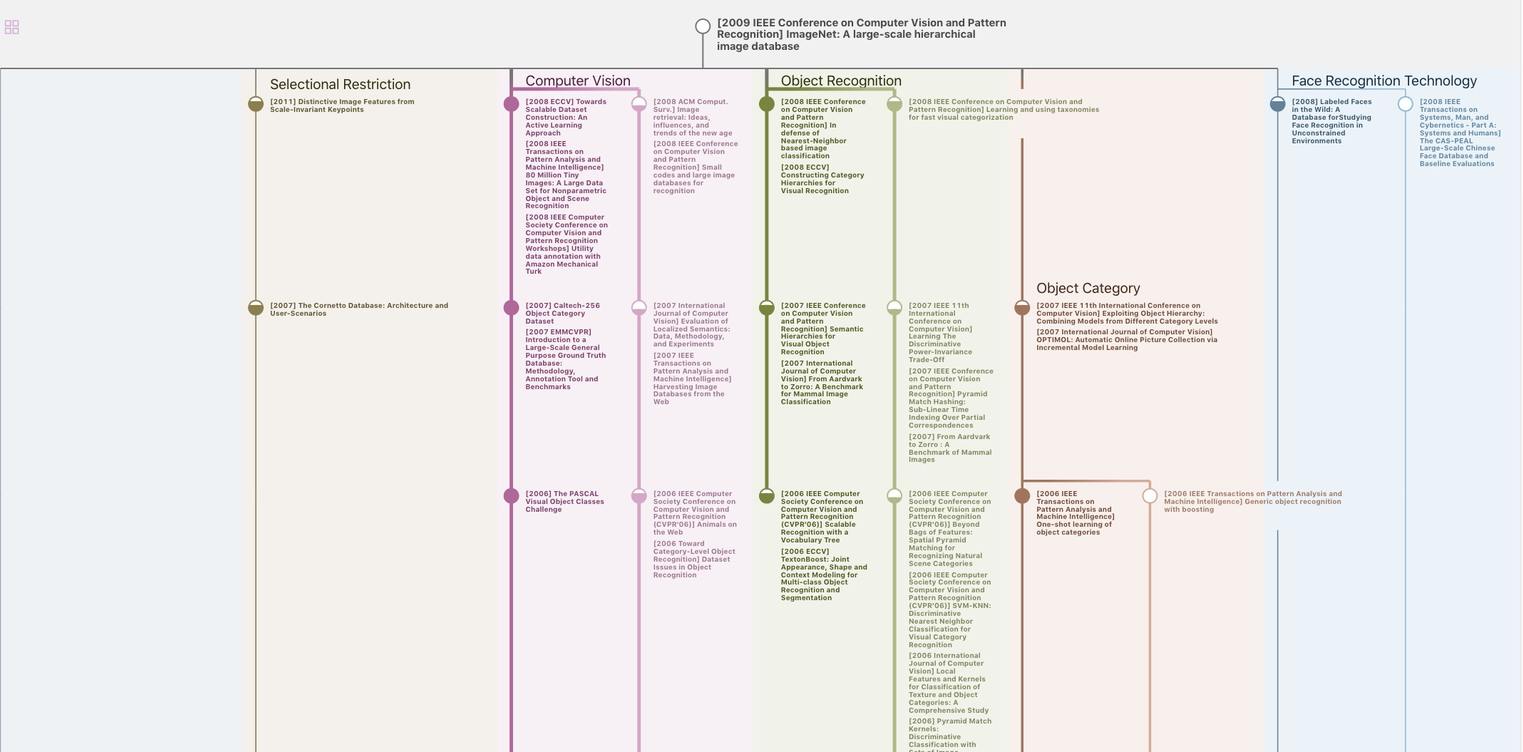
生成溯源树,研究论文发展脉络
Chat Paper
正在生成论文摘要