Use of Machine Learning to Differentiate Residual Tumor from Radiation Changes in Head and Neck Cancer Patients Treated with Definitive Chemoradiotherapy
INTERNATIONAL JOURNAL OF RADIATION ONCOLOGY BIOLOGY PHYSICS(2020)
摘要
Surveillance imaging for head and neck cancer patients treated with definitive chemoradiotherapy has difficulty in differentiating residual disease from radiation changes or inflammation. Early detection of the residual disease is particularly crucial as those patients have poor outcomes if not treated aggressively. On the other hand, these shortcomings in the imaging might lead to unnecessary interventions, including salvage surgery in patients without residual disease. The purpose of this study is to assess the use of machine learning models to differentiate residual disease from radiation changes using radiomics features extracted from surveillance CT and PET scans. A HIPPA-compliant, IRB-approved retrospective posthoc analysis of patients with squamous cell carcinoma of the head and neck treated with definitive chemoradiotherapy at our institution was performed. From them, 25 patients reported having a residual disease on the first surveillance CT soft tissue of the neck (at two months after chemoradiation) were selected. The information regarding further follow up imaging, salvage surgery with pathology, and long-term outcomes were collected. All gross tumor volumes (GTVs) were transferred from the treatment planning CT scan to a DICOM viewer (MIM® MaestroTM Software Inc., v6.7.10). Then, a radiation oncologist contoured the residual lesions in the two months follow up CT (GTV post-CT) and three months follow up PET scan (GTV post PET/CT) through MIM's tools. Next, GTVs were exported to MatLab® using an extension written in Java, which was incorporated into MIM. Then, radiomic features were extracted from each tumor through quantitative in-house MatLab algorithms using different approaches without normalization. Finally, neural network machine learning models were constructed to predict (1) residual tumor from PET/CT radiomics (2) residual tumor from CT radiomics (3) positive PET/CT findings from CT radiomics. Each of the 250 radiomics features without normalization was used to construct the neural network models. The model using PET/CT radiomic features was able to differentiate residual tumor from radiation changes had a modest discriminative ability (AUC =0.69). Predicting residual tumor from CT radiomic features was not successful (AUC=0.52). The model to predict positive PET/CT findings from CT soft tissue neck had a reasonable discriminative ability (AUC =0.70). A machine learning model using PET/CT radiomic features was able to differentiate residual tumor from radiation changes in a small group of head and neck cancer patients treated with definitive chemoradiotherapy. This model could be used to identify patients with a high probability of residual disease, which needs aggressive salvage surgery while preventing other patients with radiation changes or inflammation to avoid unnecessary treatment and associated morbidity. This model needs to be validated in a larger cohort of patients before a generalized use. Reference Wong AJ, Kanwar A, Mohamed AS, Fuller CD. Radiomics in head and neck cancer: from exploration to application. Transl Cancer Res. 2016;5(4):371-382.
更多查看译文
关键词
neck cancer patients,differentiate residual tumor,definitive chemoradiotherapy,cancer patients
AI 理解论文
溯源树
样例
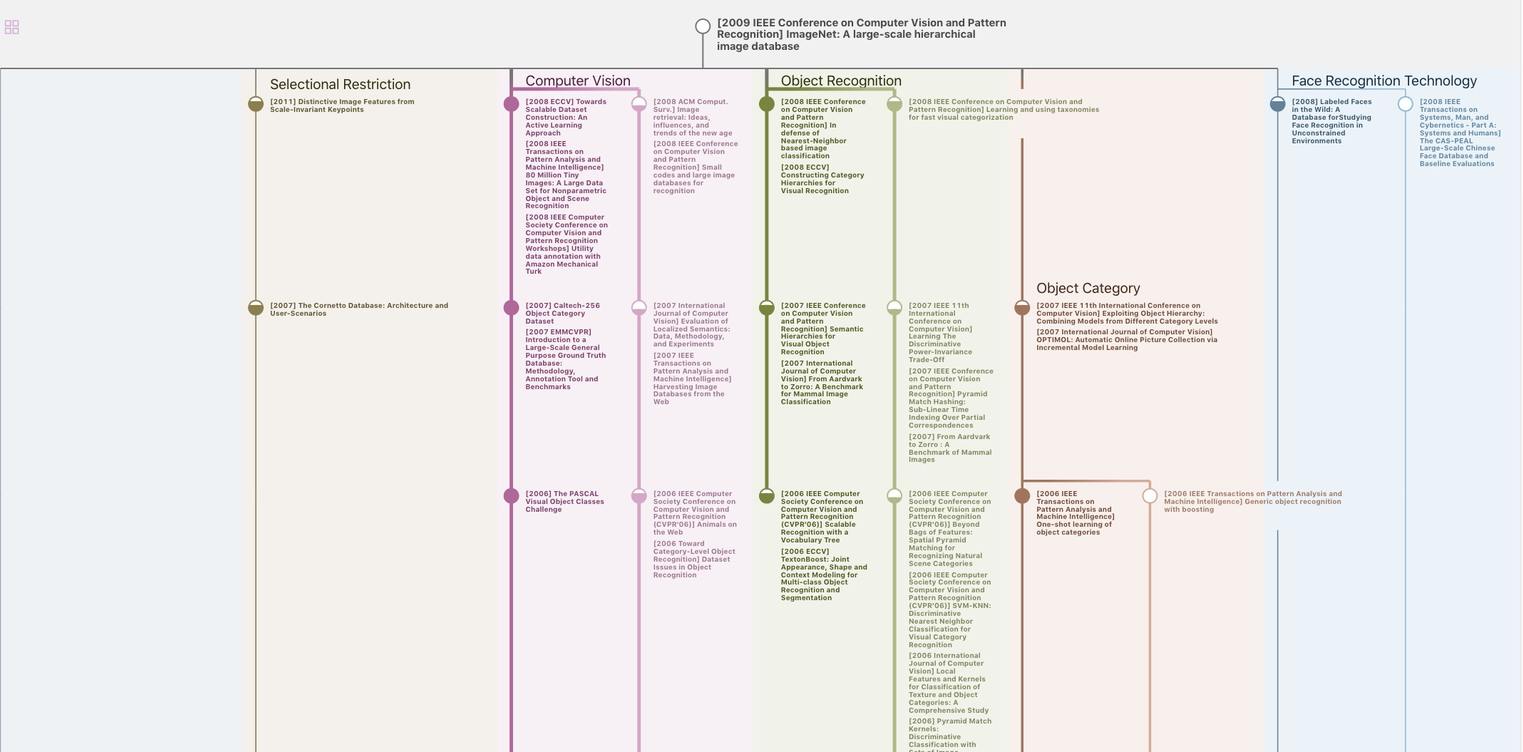
生成溯源树,研究论文发展脉络
Chat Paper
正在生成论文摘要