Generation and Evaluation of Factual and Counterfactual Explanations for Decision Trees and Fuzzy Rule-based Classifiers
IEEE International Conference on Fuzzy Systems(2020)
关键词
Explainable Artificial Intelligence,Counterfactuals,Decision Trees,Fuzzy Inference Systems,Natural Language Generation
AI 理解论文
溯源树
样例
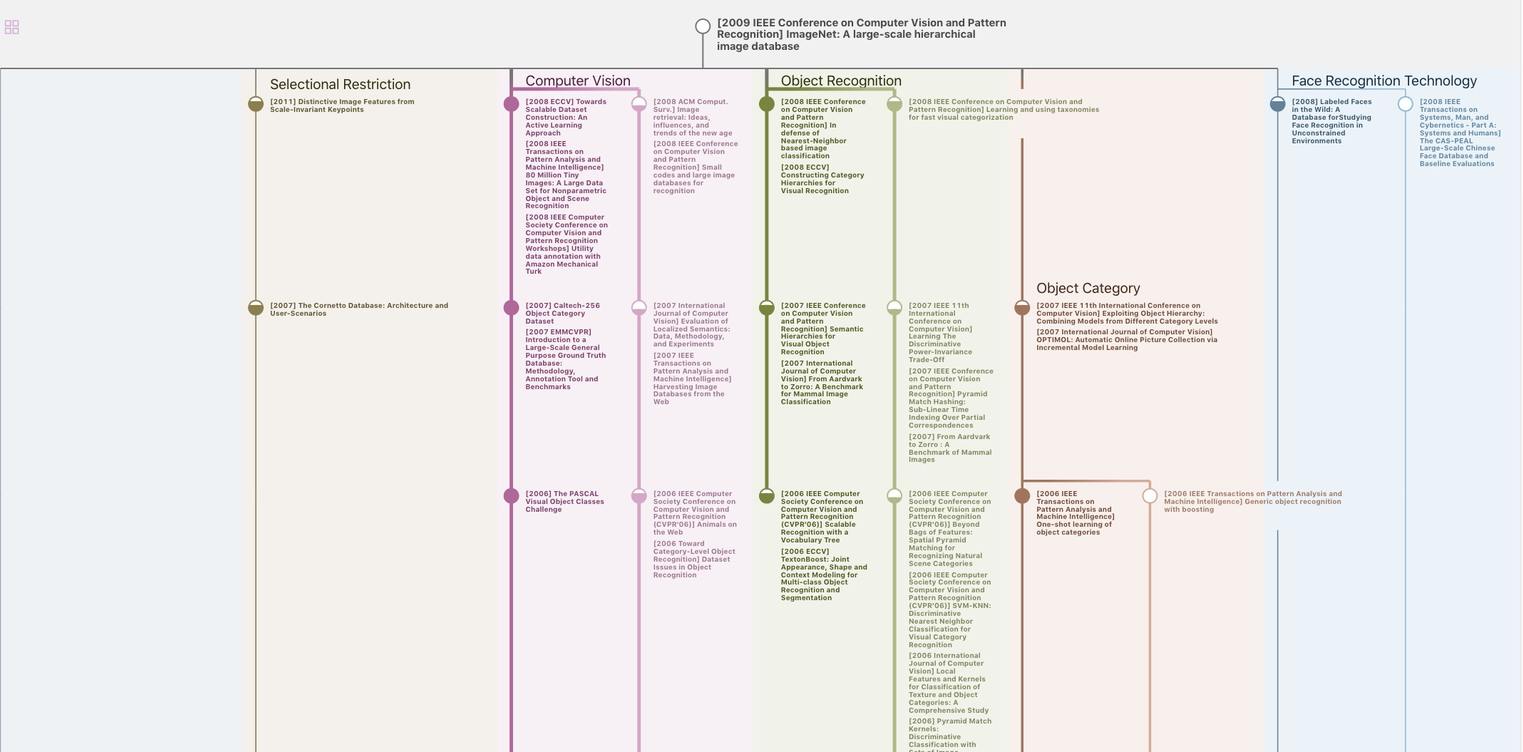
生成溯源树,研究论文发展脉络
Chat Paper
正在生成论文摘要