SAR Image Ship Object Generation and Classification With Improved Residual Conditional Generative Adversarial Network
IEEE GEOSCIENCE AND REMOTE SENSING LETTERS(2022)
摘要
Image generation by the conditional generative adversarial network (CGAN) can provide rich samples for supervised classification tasks. However, the unstable gradient updating problem makes it difficult to be used for image generation, especially for the high-resolution SAR image. In this letter, an improved residual condition generation network is proposed to enhance the quality of generated images and classification accuracy for hard negative examples. First, a residual convolutional-based block is built to clear the detail texture of different types of targets. Then, the gradient penalty and Wasserstein loss are used as discriminators to improve the similarity between real samples and the intra diversity of a generated images. We test the proposed method on generation and classification of three kinds of commercial ships using C-band 3-m Gaofen-3 (GF-3) SAR images. Experimental results show that our method can generate high-quality ship samples and achieve good classification accuracy.
更多查看译文
关键词
Marine vehicles,Synthetic aperture radar,Gallium nitride,Generators,Generative adversarial networks,Image generation,Training,Generative adversarial network (GAN),high-resolution image generation,SAR,ship classification
AI 理解论文
溯源树
样例
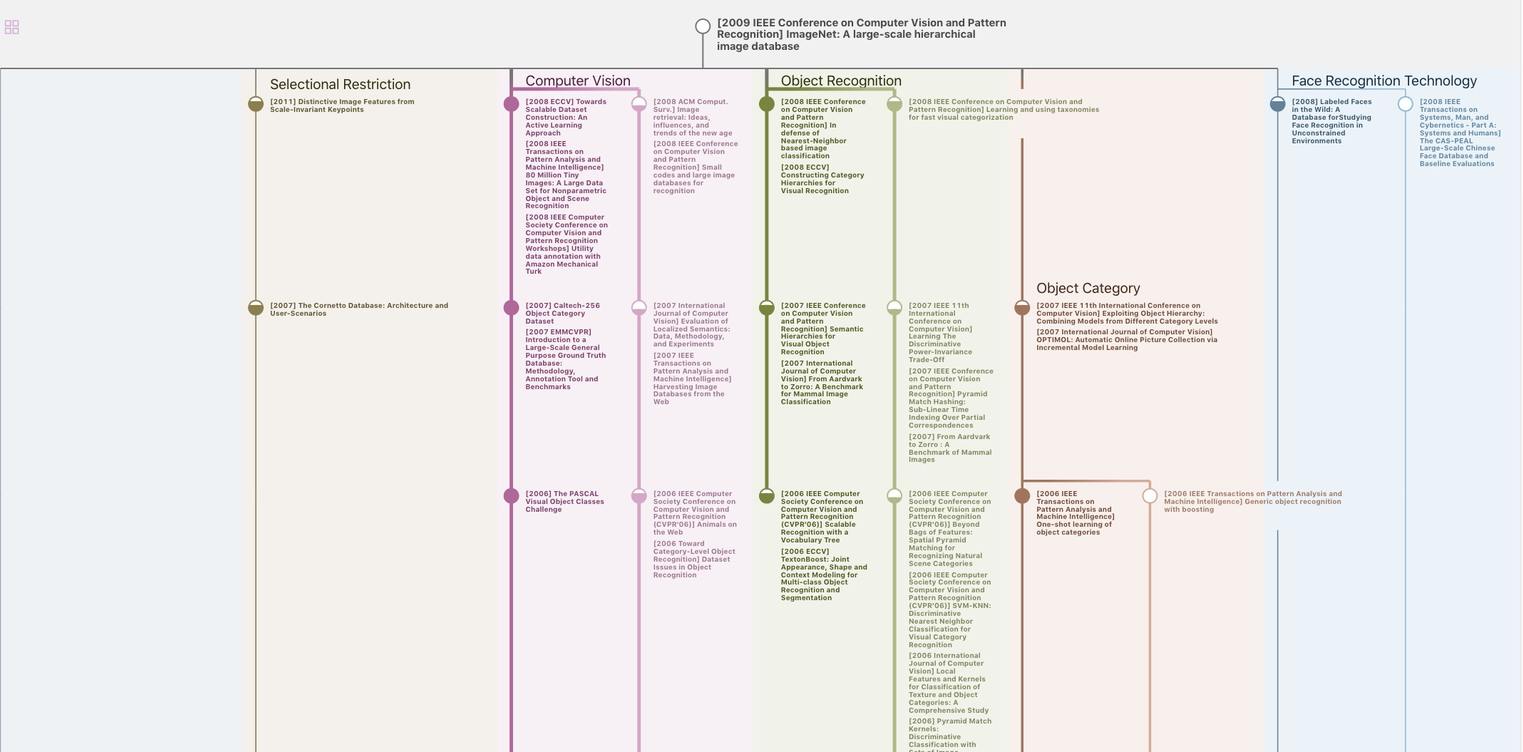
生成溯源树,研究论文发展脉络
Chat Paper
正在生成论文摘要