Multiple Kernel Driven Clustering With Locally Consistent and Selfish Graph in Industrial IoT
IEEE Transactions on Industrial Informatics(2021)
摘要
In the cognitive computing of intelligent industrial Internet of Things, clustering is a fundamental machine learning problem to exploit the latent data relationships. To overcome the challenge of kernel choice for nonlinear clustering tasks, multiple kernel clustering (MKC) has attracted intensive attention. However, existing graph-based MKC methods mainly aim to learn a consensus kernel as well as an affinity graph from multiple candidate kernels, which cannot fully exploit the latent graph information. In this article, we propose a novel pure graph-based MKC method. Specifically, a new graph model is proposed to preserve the local manifold structure of the data in kernel space so as to learn multiple candidate graphs. Afterward, the latent consistency and selfishness of these candidate graphs are fully considered. Furthermore, a graph connectivity constraint is introduced to avoid requiring any postprocessing clustering step. Comprehensive experimental results demonstrate the superiority of our method.
更多查看译文
关键词
Clustering,cognitive computing,graph learning,industrial Internet of Things (IIoT),multiple kernel clustering (MKC)
AI 理解论文
溯源树
样例
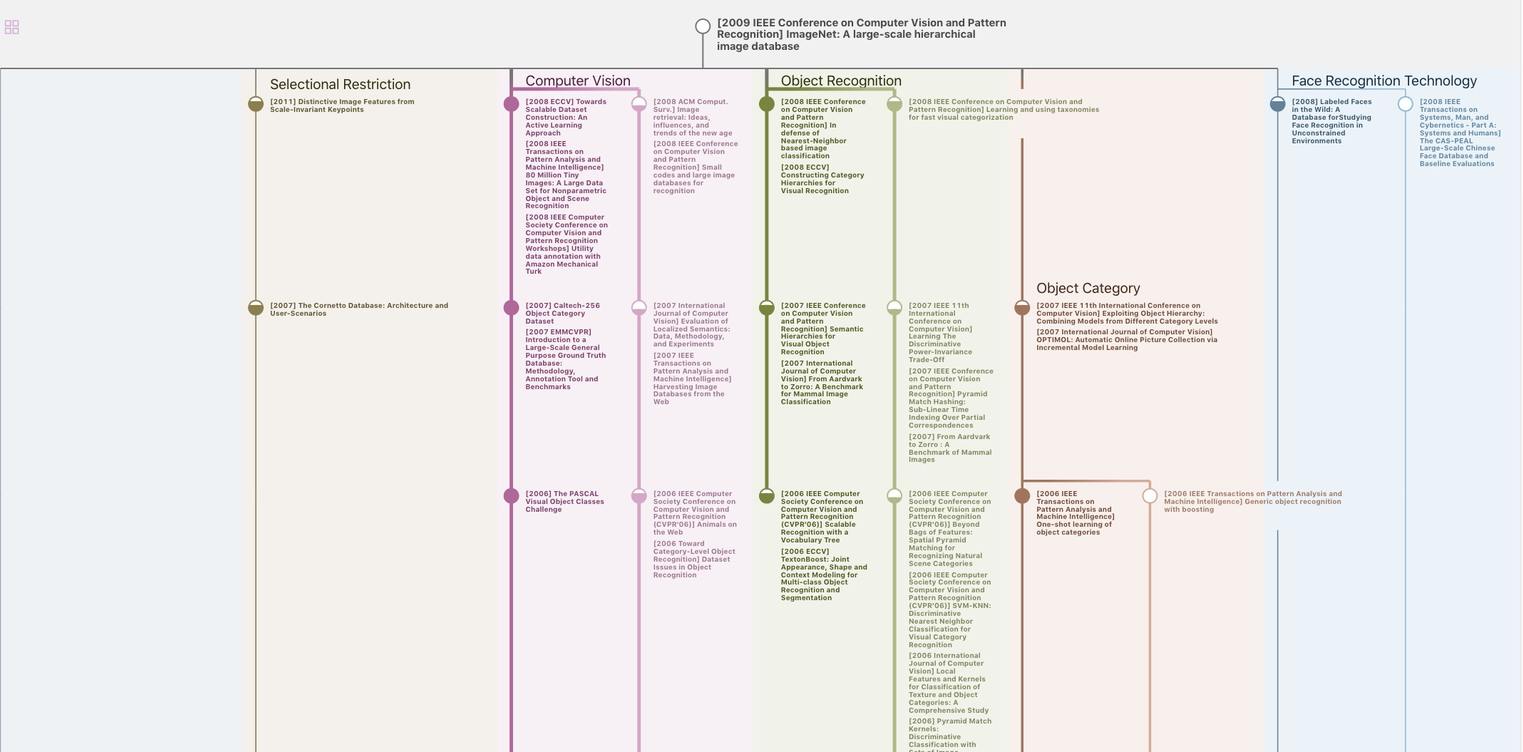
生成溯源树,研究论文发展脉络
Chat Paper
正在生成论文摘要