A threshold self-setting condition monitoring scheme for wind turbine generator bearings based on deep convolutional generative adversarial networks
Measurement(2021)
摘要
Long-term reliable health condition monitoring (HCM) of a wind turbine is an essential method to avoid catastrophic failure results. Existing unsupervised learning methods, such as auto-encoder (AE) and denoising auto-encoder (DAE) models, are utilized to the condition monitoring of wind turbines. The critical bottleneck of these models for monitoring is to determine a threshold for identifying different health conditions. Unfortunately, the threshold is usually set up with different kinds of calculation methods or even based on experience. Therefore, the uncertainty of the threshold will inevitably influence the accuracy of the monitoring process and may lead to misdiagnoses. To overcome this limitation, this research introduces a threshold self-setting HCM scheme, based on deep convolutional generative adversarial networks (DCGAN) and employed for defining a self-setting threshold to monitor wind turbine generator bearings. A threshold for HCM can be automatically created through the output of the G network in the DCGAN model, and the challenging problem of setting up a threshold can be solved. Besides, the use of Nash Equilibrium for training enables this scheme to become self-defined evaluators with a high level of consistency, without any human intervention and can be treated as a self-defined threshold, and it is a model self-tuning process. Furthermore, a sample discrepancy analysis based on the output of the G network is utilized so that a quantitative indicator of the fault severity in wind turbine generator bearings are provided. By tracking a real wind turbine dataset from the LU NAN wind farm in China, the effectiveness of the proposed scheme is verified. (C) 2020 Elsevier Ltd. All rights reserved.
更多查看译文
关键词
Wind turbine,Condition monitoring,Deep learning,Deep convolutional generative adversarial networks
AI 理解论文
溯源树
样例
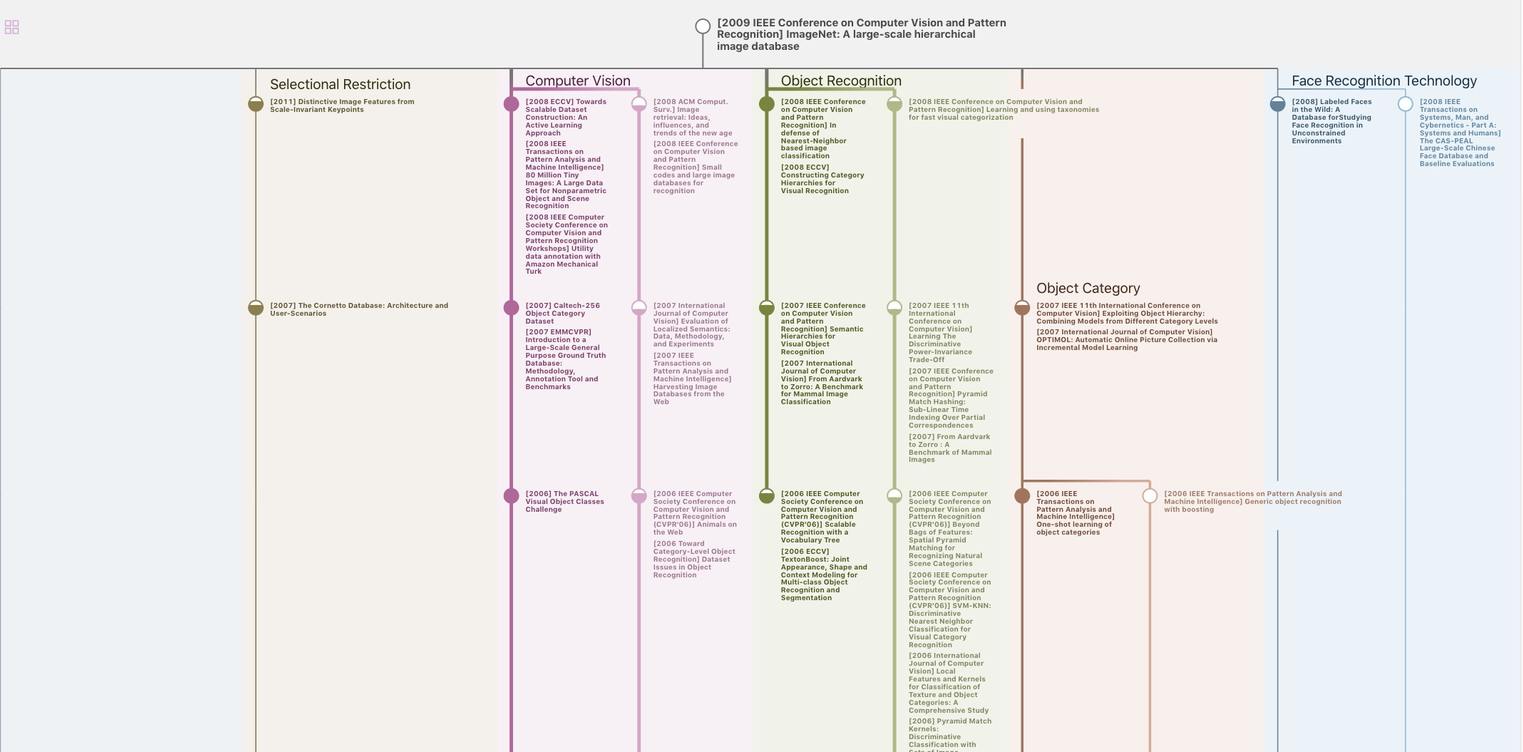
生成溯源树,研究论文发展脉络
Chat Paper
正在生成论文摘要