Sparse dimension reduction based on energy and ball statistics
Advances in Data Analysis and Classification(2021)
Abstract
Two new methods for sparse dimension reduction are introduced, based on martingale difference divergence and ball covariance, respectively. These methods can be utilized straightforwardly as sufficient dimension reduction (SDR) techniques to estimate a sufficient dimension reduced subspace, which contains all information sufficient to explain a dependent variable. Moreover, owing to their sparsity, they intrinsically perform sufficient variable selection (SVS) and present two attractive new approaches to variable selection in a context of nonlinear dependencies that require few model assumptions. The two new methods are compared to a similar existing approach for SDR and SVS based on distance covariance, as well as to classical and robust sparse partial least squares. A simulation study shows that each of the new estimators can achieve correct variable selection in highly nonlinear contexts, yet are sensitive to outliers and computationally intensive. The study sheds light on the subtle differences between the methods. Two examples illustrate how they can be applied in practice, with a slight preference for the option based on martingale difference divergence in a bioinformatics example.
MoreTranslated text
Key words
(Sufficient) dimension reduction,SDR,(Sufficient) variable selection,SVS,Nonparametric multivariate statistics,Sparse estimators
AI Read Science
Must-Reading Tree
Example
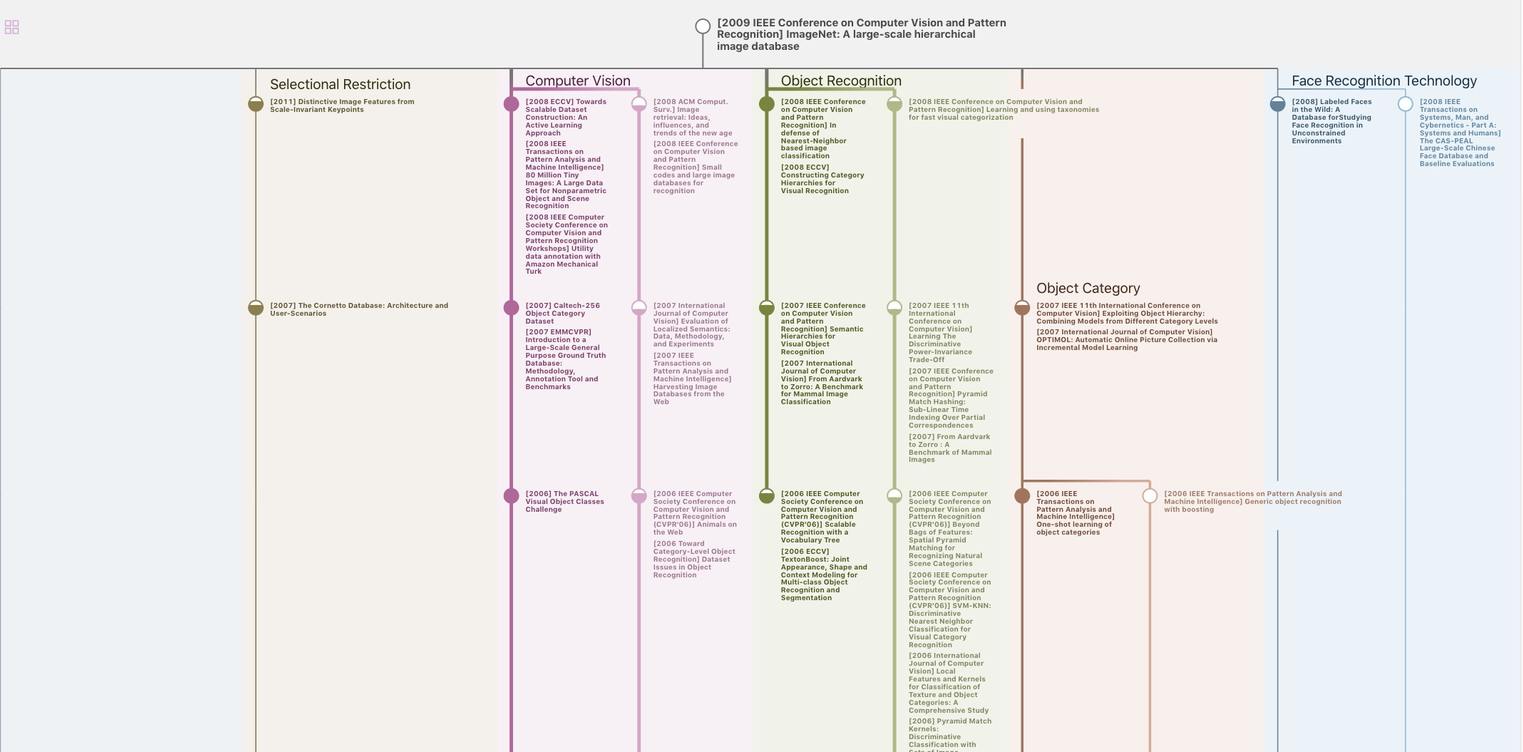
Generate MRT to find the research sequence of this paper
Chat Paper
Summary is being generated by the instructions you defined