Exploiting Diverse Characteristics And Adversarial Ambivalence For Domain Adaptive Segmentation
THIRTY-FIFTH AAAI CONFERENCE ON ARTIFICIAL INTELLIGENCE, THIRTY-THIRD CONFERENCE ON INNOVATIVE APPLICATIONS OF ARTIFICIAL INTELLIGENCE AND THE ELEVENTH SYMPOSIUM ON EDUCATIONAL ADVANCES IN ARTIFICIAL INTELLIGENCE(2021)
摘要
Adapting semantic segmentation models to new domains is an important but challenging problem. Recently enlightening progress has been made, but the performance of existing methods is unsatisfactory on real datasets where the new target domain comprises of heterogeneous sub-domains (e.g., diverse weather characteristics). We point out that carefully reasoning about the multiple modalities in the target domain can improve the robustness of adaptation models. To this end, we propose a condition-guided adaptation framework that is empowered by a special attentive progressive adversarial training (APAT) mechanism and a novel self-training policy. The APAT strategy progressively performs condition-specific alignment and attentive global feature matching. The new self-training scheme exploits the adversarial ambivalences of easy and hard adaptation regions and the correlations among target sub-domains effectively. We evaluate our method (DCAA) on various adaptation scenarios where the target images vary in weather conditions. The comparisons against baselines and the state-of-the-art approaches demonstrate the superiority of DCAA over the competitors.
更多查看译文
关键词
adversarial ambivalence,adaptive,domain,diverse characteristics
AI 理解论文
溯源树
样例
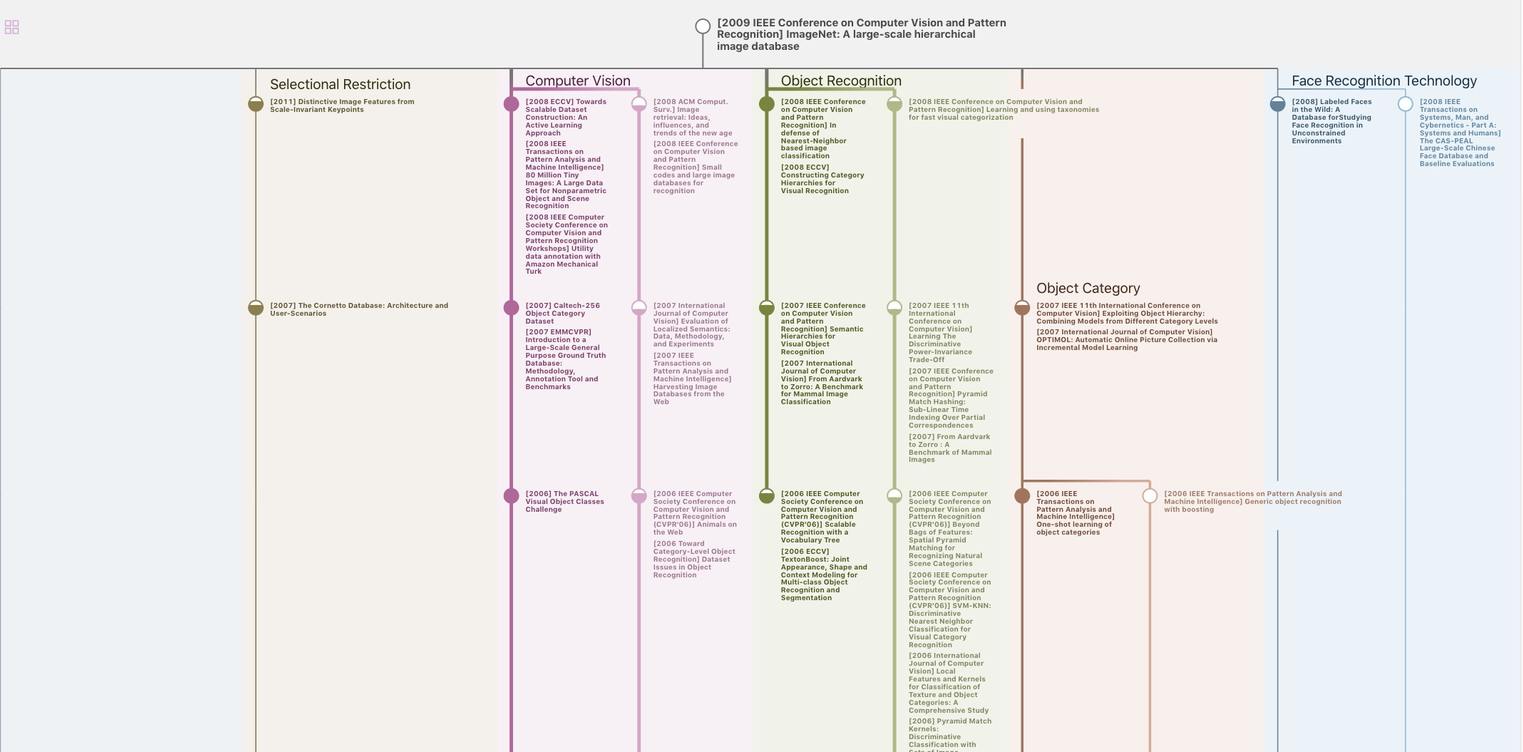
生成溯源树,研究论文发展脉络
Chat Paper
正在生成论文摘要