Machine Learning Tools to Estimate the Severity of Matrix Effects and Predict Analyte Recovery in Inductively Coupled Plasma Optical Emission Spectrometry
Talanta The International Journal of Pure and Applied Analytical Chemistry(2021)
摘要
Supervised and unsupervised machine learning methods are used to evaluate matrix effects caused by carbon and easily ionizable elements (EIEs) on analytical signals of inductively coupled plasma optical emission spectrometry (ICP OES). A simple experimental approach was used to produce a series of synthetic solutions with varying levels of matrix complexity. Analytical lines (n = 29), with total line energies (E-sum) in the 5.0-15.5 eV range, and non-analyte signals (n = 24) were simultaneously monitored throughout the study. Labeled (supervised learning) and unlabeled (unsupervised learning) data on normalized non-analyte signals (from plasma species) were used to train machine learning models to characterize matrix effect severity and predict analyte recoveries. Dimension reduction techniques, including principal component analysis, uniform manifold approximation and projection and t-distributed stochastic neighborhood embedding, were able to provide visual and quantitative representations that correlated well with observed matrix effects on low-energy atomic and high-energy ionic emission lines. Predictive models, including partial least squares regression and generalized linear models fit with the elastic net penalty, were tuned to estimate analyte recovery error when using the external standard calibration method (EC). The best predictive results were found for high-energy ionic analytical lines, e.g. Zn II 202.548 nm (E-sum = 15.5 eV), with accuracy and R-2 of 0.970 and 0.856, respectively. Two certified reference materials (CRMs) were used for method validation. The strategy described here may be used for flagging compromising matrix effects, and complement method validation based on addition/recovery experiments and CRMs analyses. Because the data analysis workflows feature signals from plasma-based species, there is potential for developing instrument software capable of alerting users in real time (i.e. before data processing) of inaccurate results when using EC.
更多查看译文
关键词
Supervised machine learning,Plasma naturally-occurring species,Unsupervised machine learning,Easily ionizable elements,Matrix effects,Accuracy prediction
AI 理解论文
溯源树
样例
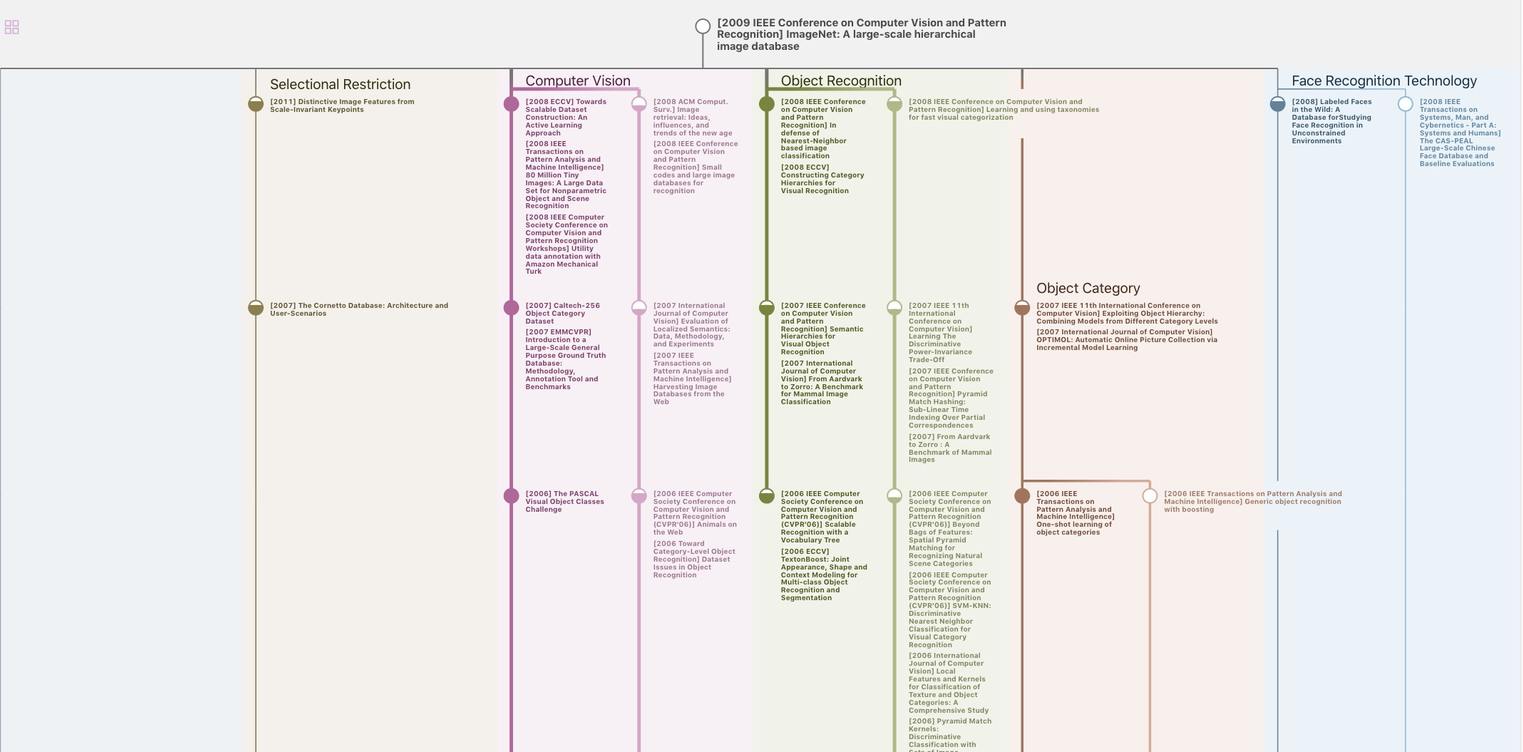
生成溯源树,研究论文发展脉络
Chat Paper
正在生成论文摘要