Baseline Methods for Bayesian Inference in Gumbel Distribution
Entropy(2020)
摘要
Usual estimation methods for the parameters of extreme value distributions only employ a small part of the observation values. When block maxima values are considered, many data are discarded, and therefore a lot of information is wasted. We develop a model to seize the whole data available in an extreme value framework. The key is to take advantage of the existing relation between the baseline parameters and the parameters of the block maxima distribution. We propose two methods to perform Bayesian estimation. Baseline distribution method (BDM) consists in computing estimations for the baseline parameters with all the data, and then making a transformation to compute estimations for the block maxima parameters. Improved baseline method (IBDM) is a refinement of the initial idea, with the aim of assigning more importance to the block maxima data than to the baseline values, performed by applying BDM to develop an improved prior distribution. We compare empirically these new methods with the Standard Bayesian analysis with non-informative prior, considering three baseline distributions that lead to a Gumbel extreme distribution, namely Gumbel, Exponential and Normal, by a broad simulation study.
更多查看译文
关键词
Bayesian inference,highly informative prior,Gumbel distribution,small dataset
AI 理解论文
溯源树
样例
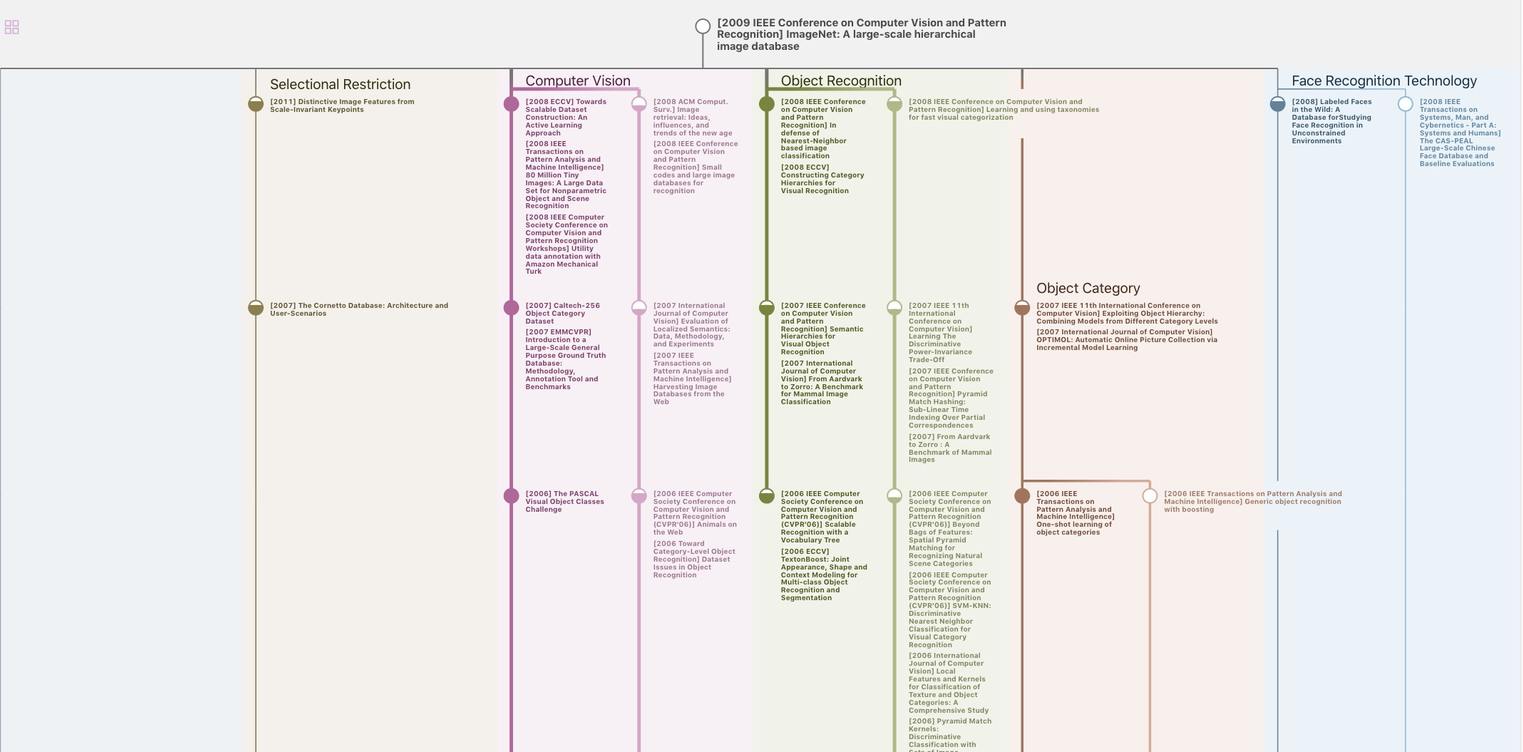
生成溯源树,研究论文发展脉络
Chat Paper
正在生成论文摘要