Performance of the Belle II Aerogel-Based Ring-Imaging Cherenkov Counter System in SuperKEKB 2019 Phase 3 Operation
Y-T Lai,I Adachi,L. Burmistrov,K. Furui,T. Iijima,S. Iwata,R. Giordano,H. Kakuno,G. Karyan,H. Kawai,T. Kawasaki,H. Kindo, H. Kitamura, M. Kobayashi,T. Kohriki,T. Konno,S. Korpar, P. Krizan,T. Kumita, K. Kuze,G. Nazaryan,S. Nishida,M. Nishimura,K. Ogawa,S. Ogawa, R. Pestotnik,L. Santelj,M. Shoji,T. Sumiyoshi,M. Tabata, M. Tsurufuji,E. Waheed,M. Yonenaga,Y. Yusa Journal of instrumentation(2020)
关键词
Cherenkov detectors,Particle identification methods
AI 理解论文
溯源树
样例
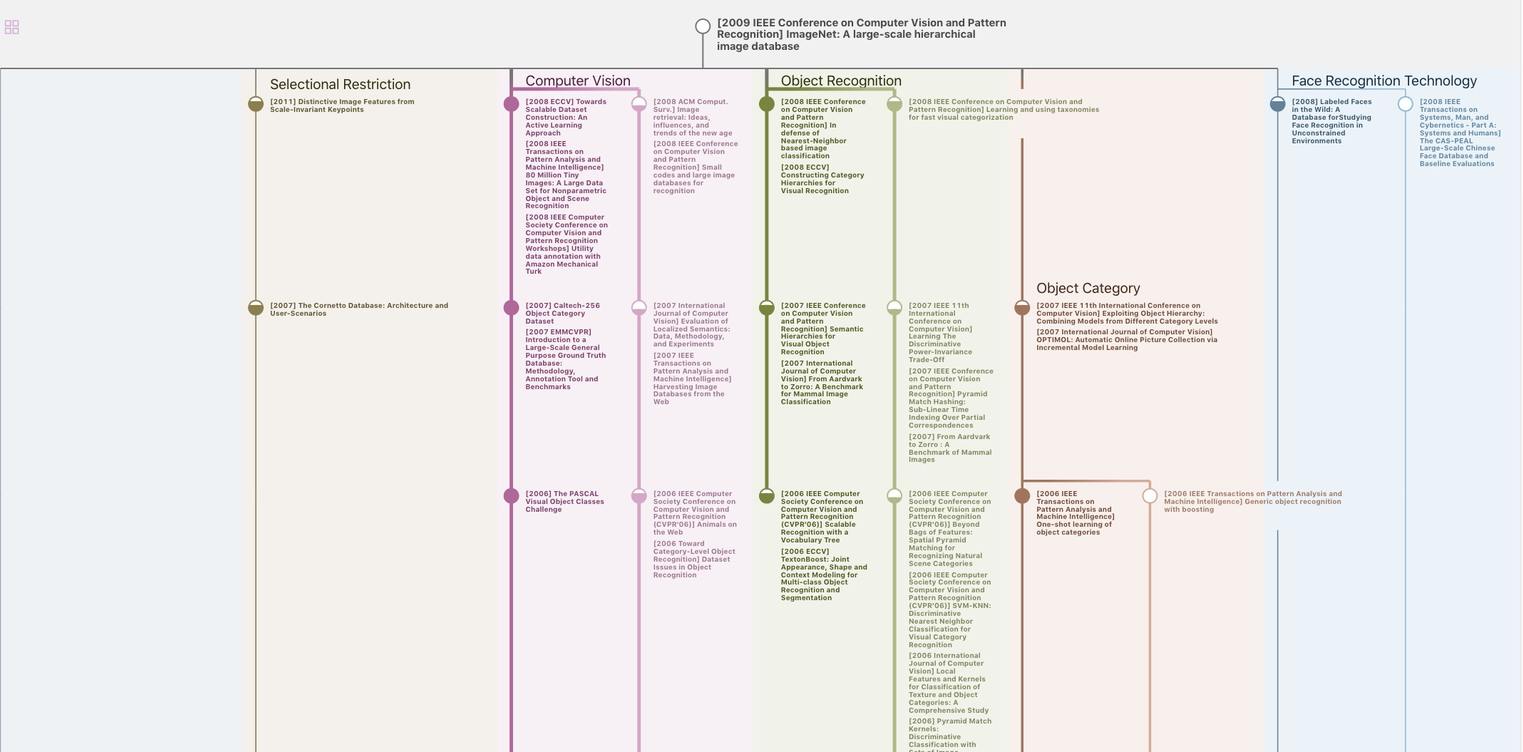