A Hierarchical Multi-Modal Encoder for Moment Localization in Video Corpus
arxiv(2020)
摘要
Identifying a short segment in a long video that semantically matches a text query is a challenging task that has important application potentials in language-based video search, browsing, and navigation. Typical retrieval systems respond to a query with either a whole video or a pre-defined video segment, but it is challenging to localize undefined segments in untrimmed and unsegmented videos where exhaustively searching over all possible segments is intractable. The outstanding challenge is that the representation of a video must account for different levels of granularity in the temporal domain. To tackle this problem, we propose the HierArchical Multi-Modal EncodeR (HAMMER) that encodes a video at both the coarse-grained clip level and the fine-grained frame level to extract information at different scales based on multiple subtasks, namely, video retrieval, segment temporal localization, and masked language modeling. We conduct extensive experiments to evaluate our model on moment localization in video corpus on ActivityNet Captions and TVR datasets. Our approach outperforms the previous methods as well as strong baselines, establishing new state-of-the-art for this task.
更多查看译文
关键词
moment localization,video corpus,multi-modal
AI 理解论文
溯源树
样例
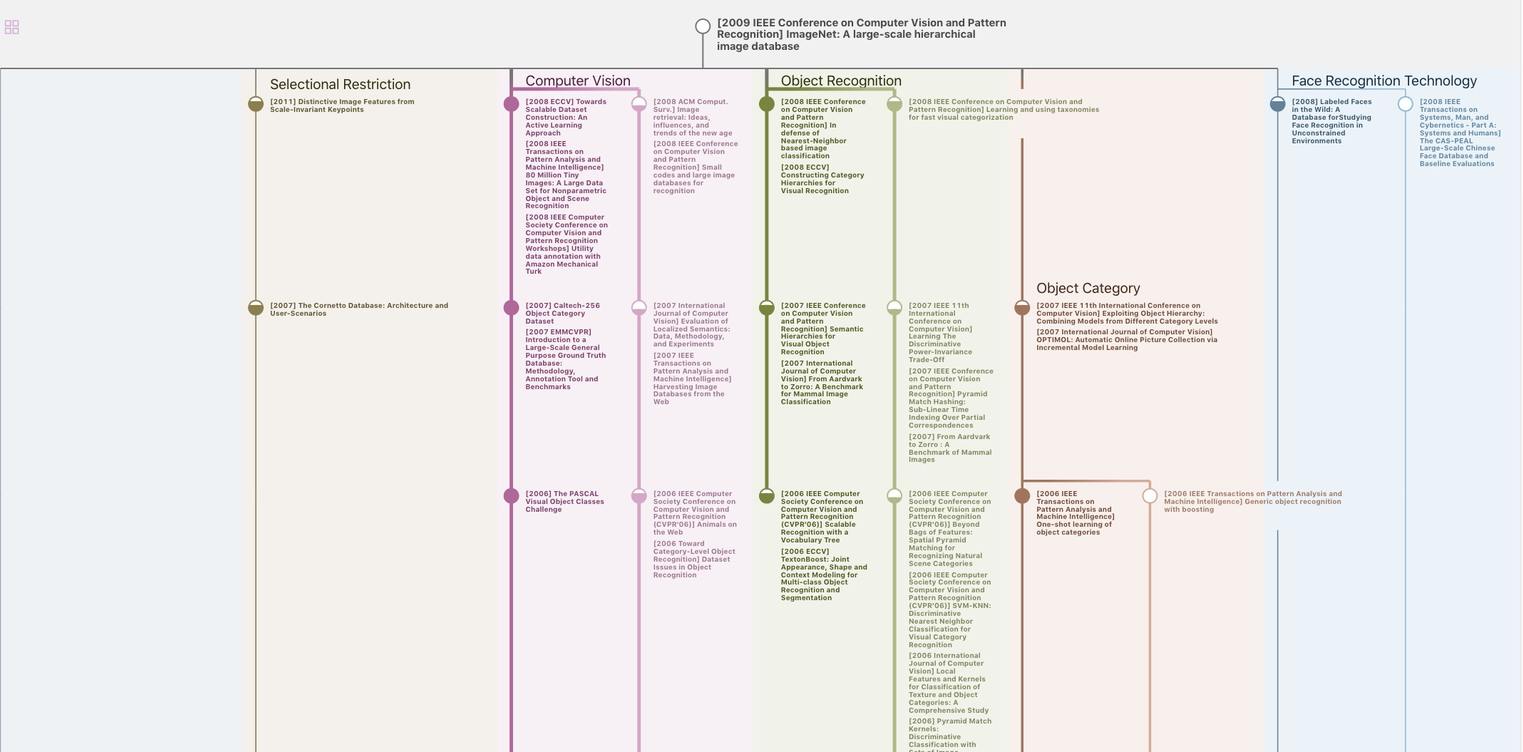
生成溯源树,研究论文发展脉络
Chat Paper
正在生成论文摘要