A New Method of Secure Authentication Based on Electromagnetic Signatures of Chipless RFID Tags and Machine Learning Approaches.
Sensors(2020)SCI 3区
Mil Tech Acad | Univ Grenoble Alpes
Abstract
In this study, we present the implementation of a neural network model capable of classifying radio frequency identification (RFID) tags based on their electromagnetic (EM) signature for authentication applications. One important application of the chipless RFID addresses the counterfeiting threat for manufacturers. The goal is to design and implement chipless RFID tags that possess a unique and unclonable fingerprint to authenticate objects. As EM characteristics are employed, these fingerprints cannot be easily spoofed. A set of 18 tags operating in V band (65–72 GHz) was designed and measured. V band is more sensitive to dimensional variations compared to other applications at lower frequencies, thus it is suitable to highlight the differences between the EM signatures. Machine learning (ML) approaches are used to characterize and classify the 18 EM responses in order to validate the authentication method. The proposed supervised method reached a maximum recognition rate of 100%, surpassing in terms of accuracy most of RFID fingerprinting related work. To determine the best network configuration, we used a random search algorithm. Further tuning was conducted by comparing the results of different learning algorithms in terms of accuracy and loss.
MoreTranslated text
Key words
chipless RFID tags,classification,authentication,machine learning,electromagnetic signature,data augmentation,python,keras
PDF
View via Publisher
AI Read Science
AI Summary
AI Summary is the key point extracted automatically understanding the full text of the paper, including the background, methods, results, conclusions, icons and other key content, so that you can get the outline of the paper at a glance.
Example
Background
Key content
Introduction
Methods
Results
Related work
Fund
Key content
- Pretraining has recently greatly promoted the development of natural language processing (NLP)
- We show that M6 outperforms the baselines in multimodal downstream tasks, and the large M6 with 10 parameters can reach a better performance
- We propose a method called M6 that is able to process information of multiple modalities and perform both single-modal and cross-modal understanding and generation
- The model is scaled to large model with 10 billion parameters with sophisticated deployment, and the 10 -parameter M6-large is the largest pretrained model in Chinese
- Experimental results show that our proposed M6 outperforms the baseline in a number of downstream tasks concerning both single modality and multiple modalities We will continue the pretraining of extremely large models by increasing data to explore the limit of its performance
Try using models to generate summary,it takes about 60s
Must-Reading Tree
Example
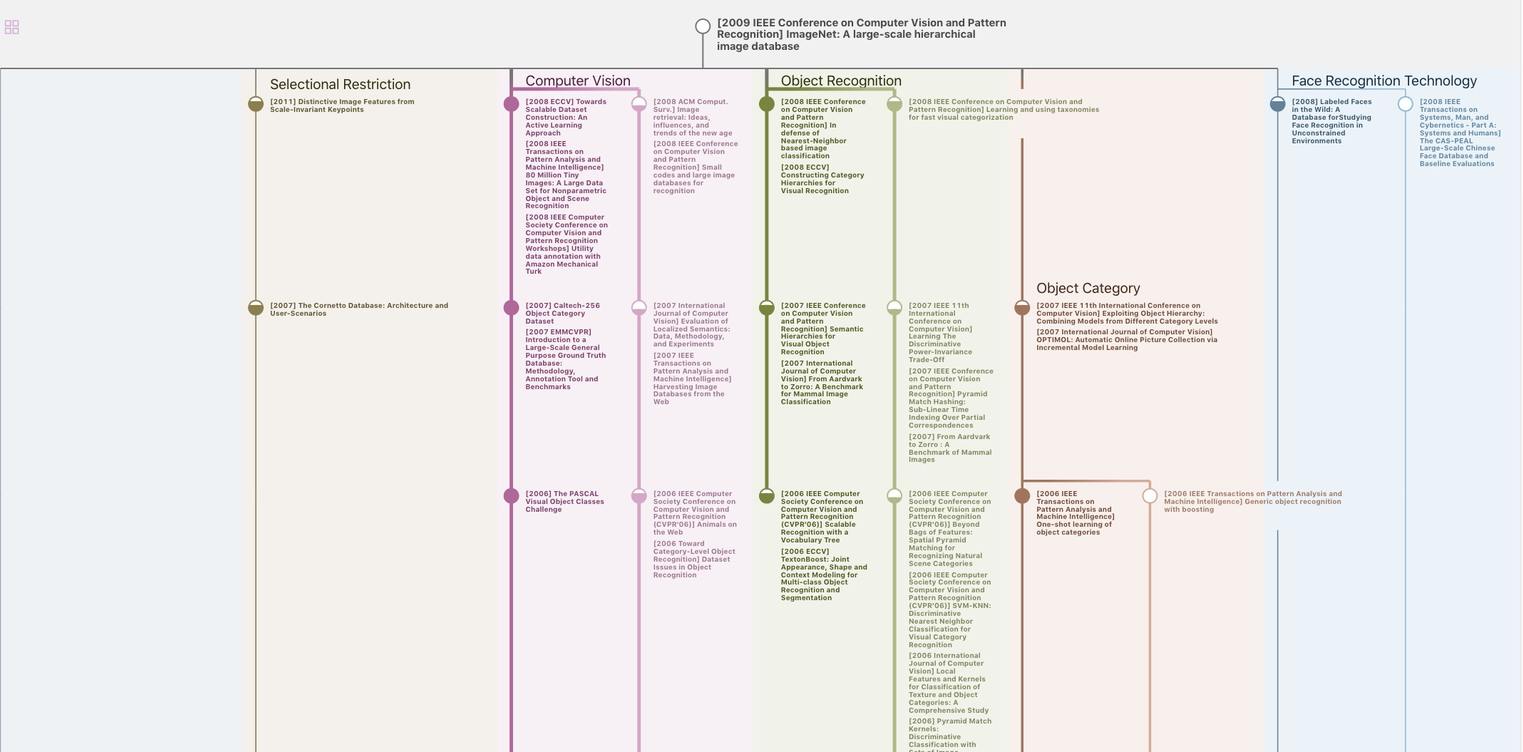
Generate MRT to find the research sequence of this paper
Related Papers
Chipless RFID Sensors for IoT Sensing and Potential Applications in Underground Mining-A Review
IEEE SENSORS JOURNAL 2023
被引用9
Robust Deep Learning-Based Detection and Classification System for Chipless Arabic RFID Letters.
ENGINEERING APPLICATIONS OF ARTIFICIAL INTELLIGENCE 2023
被引用1
Chipless RFID Tag Implementation and Machine Learning Workflow for Robust Identification
IEEE Transactions on Microwave Theory and Techniques 2023
被引用1
Evaluation of Machine Learning Models for a Chipless RFID Sensor Tag
2023 17TH EUROPEAN CONFERENCE ON ANTENNAS AND PROPAGATION, EUCAP 2023
被引用3
Analysis of Machine Learning Algorithms for USRP-based Smart Chipless RFID Readers
2023 17TH EUROPEAN CONFERENCE ON ANTENNAS AND PROPAGATION, EUCAP 2023
被引用2
Chipless Wireless Sensor Coupled with Machine Learning for Oil Temperature Monitoring
IEEE SENSORS JOURNAL 2023
被引用0
Evaluation of a U-Shaped Convolutional Neural Network for RCS Based Chipless RFID Systems
2023 IEEE 13TH INTERNATIONAL CONFERENCE ON RFID TECHNOLOGY AND APPLICATIONS, RFID-TA 2023
被引用0
JOURNAL OF INFORMATION AND TELECOMMUNICATION 2024
被引用0
IEEE INTERNET OF THINGS JOURNAL 2024
被引用2
Data Disclaimer
The page data are from open Internet sources, cooperative publishers and automatic analysis results through AI technology. We do not make any commitments and guarantees for the validity, accuracy, correctness, reliability, completeness and timeliness of the page data. If you have any questions, please contact us by email: report@aminer.cn
Chat Paper
去 AI 文献库 对话