Using a Neural Network Approach to Accelerate Disequilibrium Chemistry Calculations in Exoplanet Atmospheres.
Monthly Notices of the Royal Astronomical Society(2023)
摘要
In this era of exoplanet characterization with JWST, the need for a fast implementation of classical forward models to understand the chemical and physical processes in exoplanet atmospheres is more important than ever. Notably, the time-dependent ordinary differential equations to be solved by chemical kinetics codes are very time-consuming to compute. In this study, we focus on the implementation of neural networks to replace mathematical frameworks in one-dimensional chemical kinetics codes. Using the gravity gradient, temperature-pressure profiles, initial mixing ratios, and stellar flux of a sample of hot-Jupiter's atmospheres as free parameters, the neural network is built to predict the mixing ratio outputs in steady state. The architecture of the network is composed of individual autoencoders for each input variable to reduce the input dimensionality, which is then used as the input training data for an LSTM-like neural network. Results show that the autoencoders for the mixing ratios, stellar spectra, and pressure gradients are exceedingly successful in encoding and decoding the data. Our results show that in 90 per cent of the cases, the fully trained model is able to predict the evolved mixing ratios of the species in the hot-Jupiter atmosphere simulations. The fully trained model is & SIM;10(3) times faster than the simulations done with the forward, chemical kinetics model while making accurate predictions.
更多查看译文
关键词
exoplanets,planets and satellites: atmospheres,planets and satellites: gaseous planets
AI 理解论文
溯源树
样例
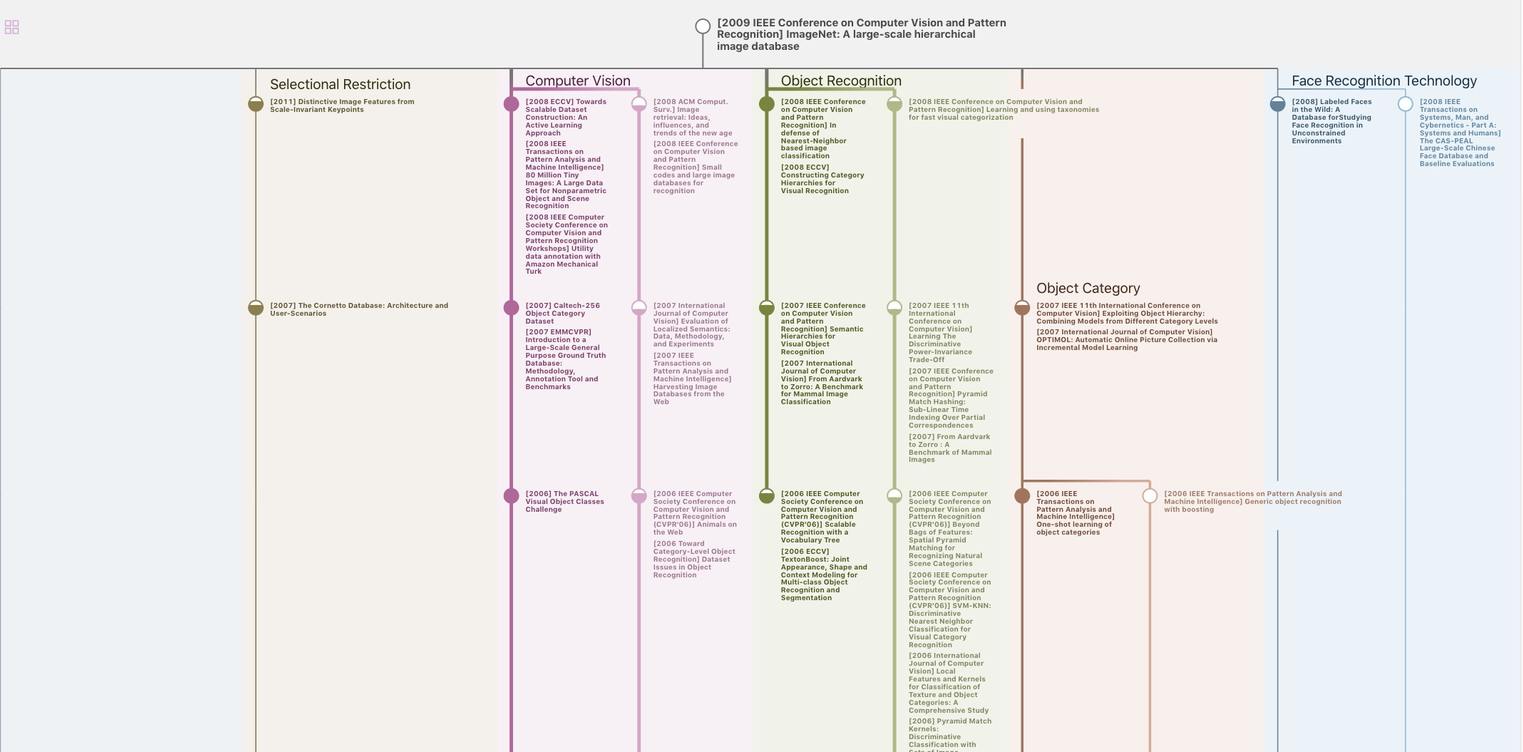
生成溯源树,研究论文发展脉络
Chat Paper
正在生成论文摘要