MP47-14 OBJECTIVE METRICS OF HIGH COGNITIVE WORKLOAD DURING ROBOTIC PARTIAL NEPHRECTOMY: A PILOT STUDY
The Journal of urology/The journal of urology(2020)
摘要
You have accessJournal of UrologySurgical Technology & Simulation: Training & Skills Assessment II (MP47)1 Apr 2020MP47-14 OBJECTIVE METRICS OF HIGH COGNITIVE WORKLOAD DURING ROBOTIC PARTIAL NEPHRECTOMY: A PILOT STUDY Jackie Cha, James Steward*, Jay Sulek, Chandru Sundaram, Juan Wachs, and Denny Yu Jackie ChaJackie Cha More articles by this author , James Steward*James Steward* More articles by this author , Jay SulekJay Sulek More articles by this author , Chandru SundaramChandru Sundaram More articles by this author , Juan WachsJuan Wachs More articles by this author , and Denny YuDenny Yu More articles by this author View All Author Informationhttps://doi.org/10.1097/JU.0000000000000902.014AboutPDF ToolsAdd to favoritesDownload CitationsTrack CitationsPermissionsReprints ShareFacebookLinked InTwitterEmail Abstract INTRODUCTION AND OBJECTIVE: High cognitive load adversely affects surgeon performance. Technology which automatically detects cognitive workload could lead to better training and fewer clinical errors. Electroencephalography (EEG) is one technology to measure this cognitive workload. The aim of this study is to determine if a surgeon's cognitive workload can be accurately predicted by EEG during robotic-assisted laparoscopic partial nephrectomy (RLPN) by comparing high- and low-risk steps of the procedure. METHODS: A 14 channel EEG was performed on one surgeon during seven RLPN cases between 2017 and 2018 at a single academic institution. The procedure was divided into low and high risk segments. The low risk segment included exposure of the renal mass until just before clamping the renal hilum and after the hilum was unclamped. The high cognitive workload segment of the procedure included the entire warm ischemia time. EEGLab was used to process the signals, and a preprocessing pipeline was utilized to determine the alpha, theta, and low/high beta mean power, which was used to determine workload. Cognitive load between the low and high risk periods were compared using t-tests. RESULTS: Twenty-five high cognitive workload steps and 26 low workload steps were analyzed. Among the frontal channels, the alpha and low/high beta power trended toward a significant difference during the low and high workload levels. Significant differences in mean power between low and high workload were observed on the left frontal channel (AF3) low beta (p=0.05) and high beta (p=0.02) bands, while there was a trend toward a significant difference in the alpha (p=0.11) and theta (p=0.32) bands. No significant differences between low/high workload were observed in the remaining channels or bands (Figure 1). CONCLUSIONS: EEG can be used to assess a surgeon’s cognitive workload during procedures such as RLPN. Measuring cognitive workload can accelerate the learning curve of trainees required to perform high cognitive tasks and can be used as a metric for surgical competency. Further studies should be performed to confirm this finding and to explore its application to other aspects of surgical training. Source of Funding: Walther Oncology Physical Sciences and Engineering Research Embedding Program Intuitive Surgical, Inc. © 2020 by American Urological Association Education and Research, Inc.FiguresReferencesRelatedDetails Volume 203Issue Supplement 4April 2020Page: e689-e689 Advertisement Copyright & Permissions© 2020 by American Urological Association Education and Research, Inc.MetricsAuthor Information Jackie Cha More articles by this author James Steward* More articles by this author Jay Sulek More articles by this author Chandru Sundaram More articles by this author Juan Wachs More articles by this author Denny Yu More articles by this author Expand All Advertisement PDF downloadLoading ...
更多查看译文
AI 理解论文
溯源树
样例
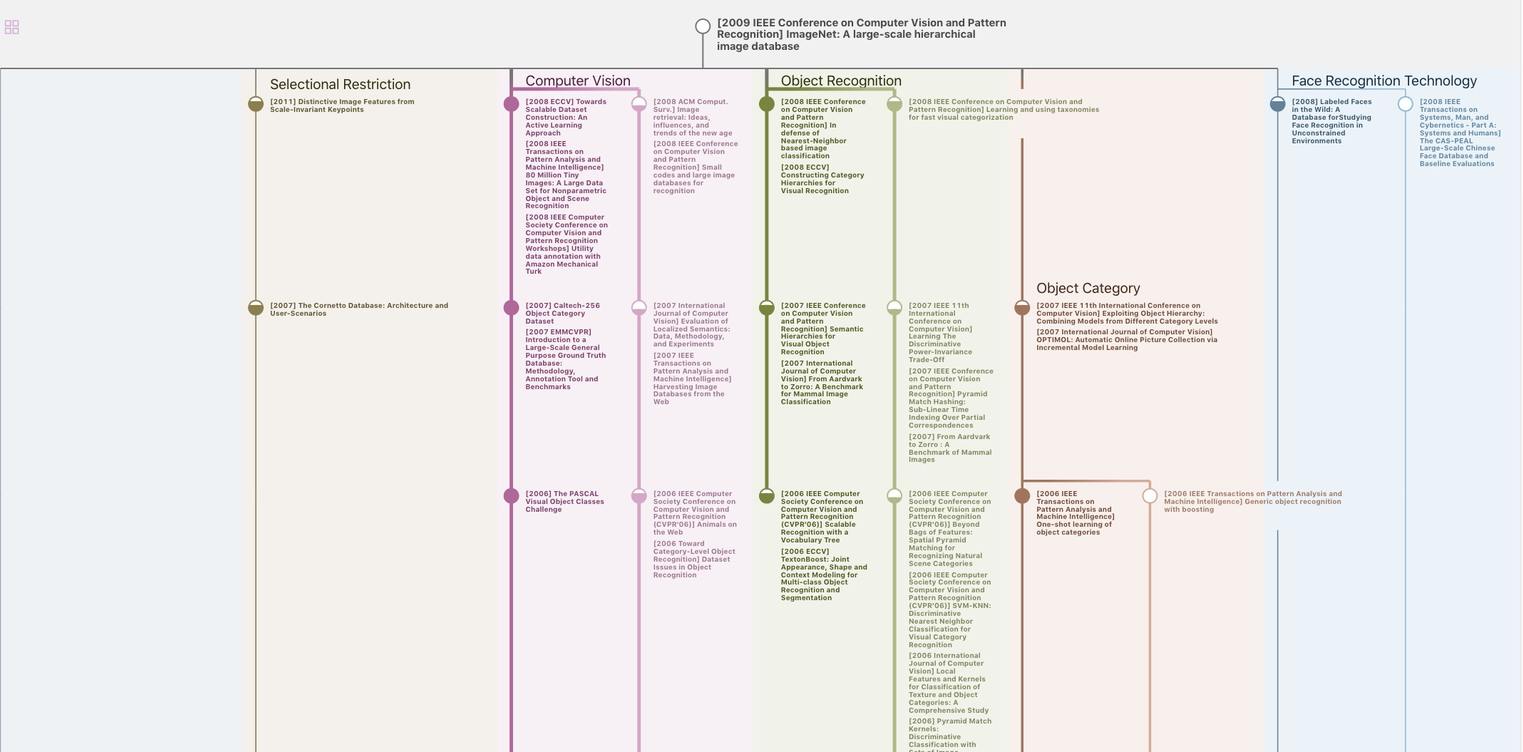
生成溯源树,研究论文发展脉络
Chat Paper
正在生成论文摘要