Optimized Co-Scheduling of Mixed-Precision Neural Network Accelerator for Real-Time Multitasking Applications
Journal of Systems Architecture (JSA)(2020)CCF BSCI 2区
Univ Elect Sci & Technol China | Veoneer Sweden AB
- Pretraining has recently greatly promoted the development of natural language processing (NLP)
- We show that M6 outperforms the baselines in multimodal downstream tasks, and the large M6 with 10 parameters can reach a better performance
- We propose a method called M6 that is able to process information of multiple modalities and perform both single-modal and cross-modal understanding and generation
- The model is scaled to large model with 10 billion parameters with sophisticated deployment, and the 10 -parameter M6-large is the largest pretrained model in Chinese
- Experimental results show that our proposed M6 outperforms the baseline in a number of downstream tasks concerning both single modality and multiple modalities We will continue the pretraining of extremely large models by increasing data to explore the limit of its performance
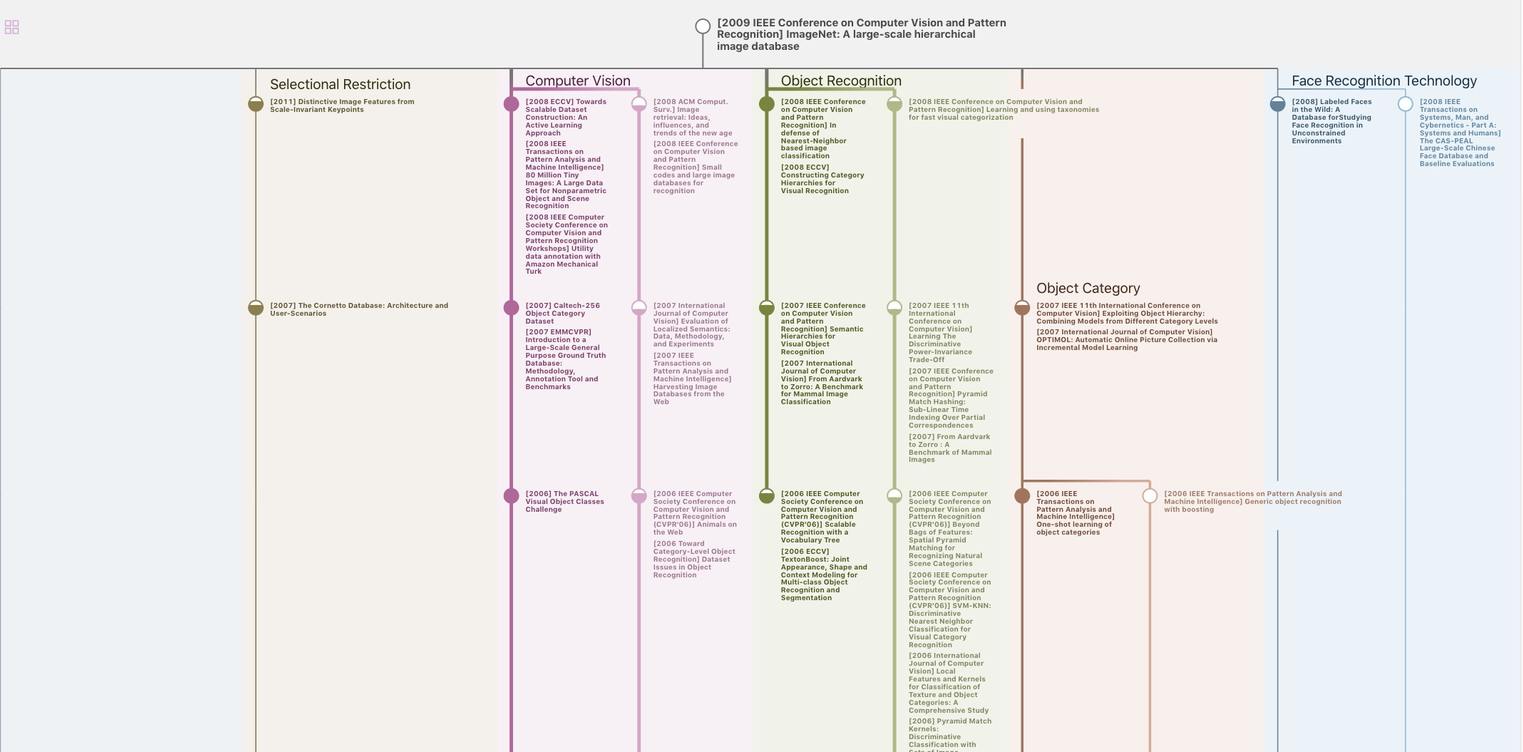
Distractor-Aware Tracking with Multi-Task and Dynamic Feature Learning
被引用2
Diffusion Geometry Derived Keypoints and Local Descriptors for 3D Deformable Shape Analysis.
被引用14
Energy Optimization of Mixed-Criticality Distributed Real-Time Embedded Systems.
被引用1
Object Tracking in Satellite Videos Based on a Lightweight Network
被引用2
Decision-Based Marginal Diffusion for Salt-and-Pepper Noise Removal in Color Images.
被引用0
Learning a Deep Regression Forest for Head Pose Estimation from a Single Depth Image.
被引用7
Learning a Twofold Siamese Network for RGB-T Object Tracking
被引用4
Facial Expression Recognition Method Based on Shallow Small Convolution Kernel Capsule Network
被引用4
Power-Efficient Layer Mapping for CNNs on Integrated CPU and GPU Platforms: A Case Study
被引用5
Energy-efficient Neural Networks with Near-Threshold Processors and Hardware Accelerators
被引用4
EAT-ML: Efficient Automatic Tuning for Machine Learning Models in Cyber Physical System
被引用1
Secure Data Set Operation Protocols for Outsourced Cloud Data to Protect User Privacy in Smart City
被引用3
FPGA Based Co-design of Storage-side Query Filter for Big Data Systems
被引用1
Enhanced Real-Time RGB-T Tracking by Complementary Learners.
被引用4
被引用3
被引用16
Low/Adaptive Precision Computation in Preconditioned Iterative Solvers for Ill-Conditioned Problems
被引用1
Accelerating Queries of Big Data Systems by Storage-Side CPU-FPGA Co-Design
被引用4
Apple Leaf Disease Recognition Method with Improved Residual Network
被引用89
被引用2
Combination of ELM and BEMD for Target Recognition of SAR Images.
被引用0
Joint Compressing and Partitioning of CNNs for Fast Edge-Cloud Collaborative Intelligence for IoT
被引用5
Highly Parallel Multi-FPGA System Compilation from Sequential C/C++ Code in the AWS Cloud
被引用0
被引用18
Winograd-CNN Accelerator Compatible with Sparse and Non-sparse Models.
被引用0
Detecting Spoofed Speeches Via Segment-Based Word CQCC and Average ZCR for Embedded Systems.
被引用1
Amphis: Managing Reconfigurable Processor Architectures with Generative Adversarial Learning.
被引用1