Imbalanced Kernel Extreme Learning Machines for Fault Detection of Aircraft Engine
JOURNAL OF DYNAMIC SYSTEMS MEASUREMENT AND CONTROL-TRANSACTIONS OF THE ASME(2020)
Abstract
To deal with class imbalance learning (CIL) problems, a novel algorithm is proposed based on kernel extreme learning machine (KELM), named KELM-CIL. To solve it, two algorithms are developed from the dual and primal spaces, respectively, thus yielding D-KELM-CIL and P-KELM-CIL. However, both D-KELM-CIL and P-KELM-CIL are not sparse algorithms. Hence, a sparse strategy based on Cholesky factorization is utilized to realize their sparseness, producing CD-KELM-CIL and CP-KELM-CIL. For large-size problems, a probabilistic trick is applied to accelerate them further, hence obtaining PCD-KELM-CIL and PCP-KELM-CIL. To test the effectiveness and efficacy of the proposed algorithms, experiments on benchmark datasets are carried out. When the proposed algorithms are applied to fault detection of aircraft engine, they show good generalization performance and real-time performance, especially for CP-KELM-CIL and PCP-KELM-CIL, which indicates that they can be developed as candidate techniques for fault detection of aircraft engine.
MoreTranslated text
Key words
machine learning,extreme learning machine,class imbalance learning,fault detection,aircraft engine
AI Read Science
Must-Reading Tree
Example
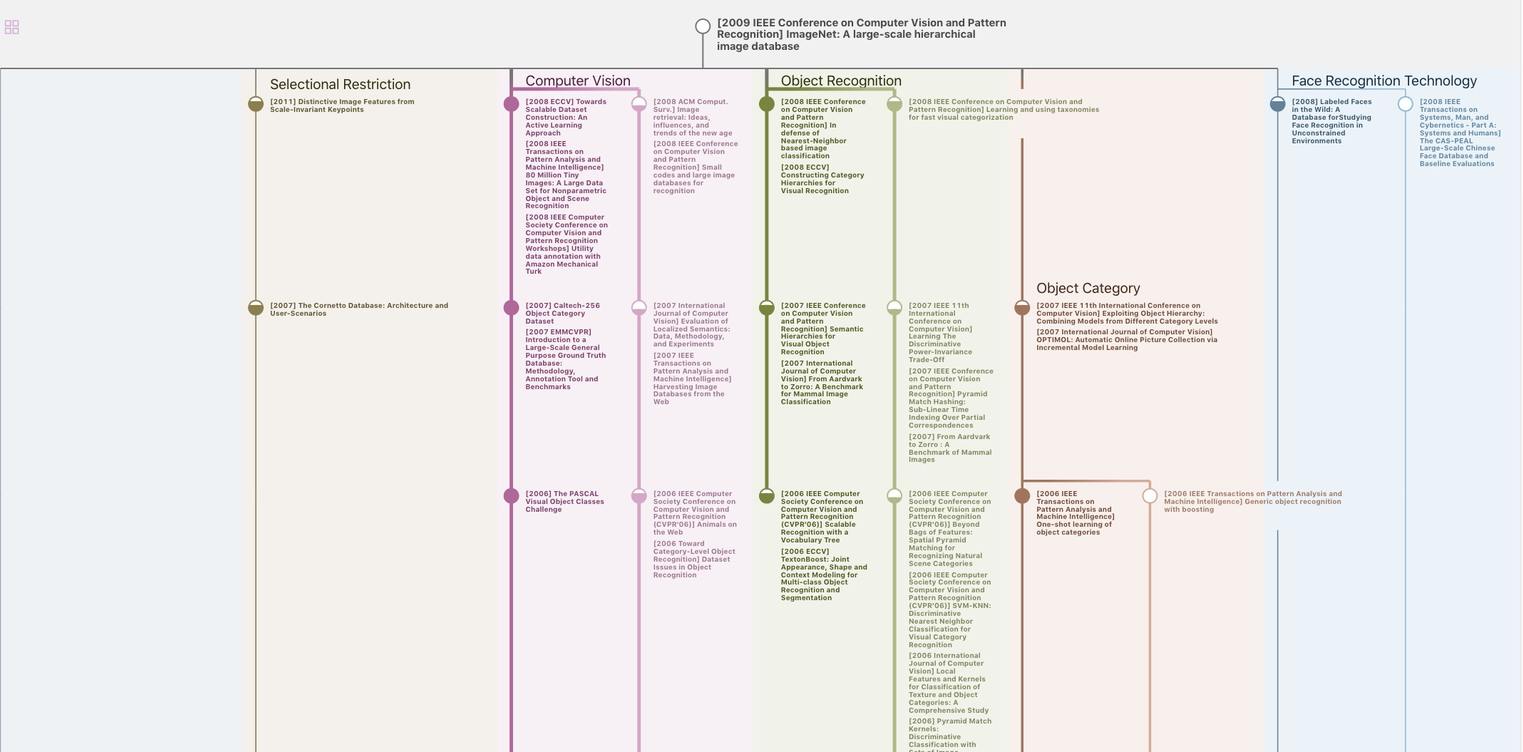
Generate MRT to find the research sequence of this paper
Chat Paper
Summary is being generated by the instructions you defined