A CNN LSTM Deep Learning Classifier for Motor Imagery EEG Detection Using a Low invasive and Low Cost BCI Headband
2020 International Conference on Intelligent Environments(2020)
摘要
Brain Computer Interfaces (BCI) can be used not only to monitor users, recognizing their mental state and the activities they perform, but also to make decisions or control their environment. Hence, BCI could improve the health and the independence of users, for example those with low mobility disabilities. In this work, we use a low-cost and low-invasive BCI headband to detect Electroencephalography (EEG) motor imagery. In particular, we propose a deep learning classifier based on Convolutional Neural Networks (CNN) and Long Short-Term Memory (LSTM) in order to detect EEG motor imagery for left and right hands. Our results report a 96.5% validation accuracy in the correct classification. Additionally, we discuss the influence of using raw data over using the data split in frequency bands in the model proposed. We also discuss the influence of certain frequency bands activity over other frequency bands in the task proposed. These results represent a promising discovery in order to democratize users’ independence by the adoption of low-cost and low-invasive technologies in combination with deep learning.
更多查看译文
关键词
Neural networks,Deep learning,EEG signals,motor imagery,BCI,user’s interaction
AI 理解论文
溯源树
样例
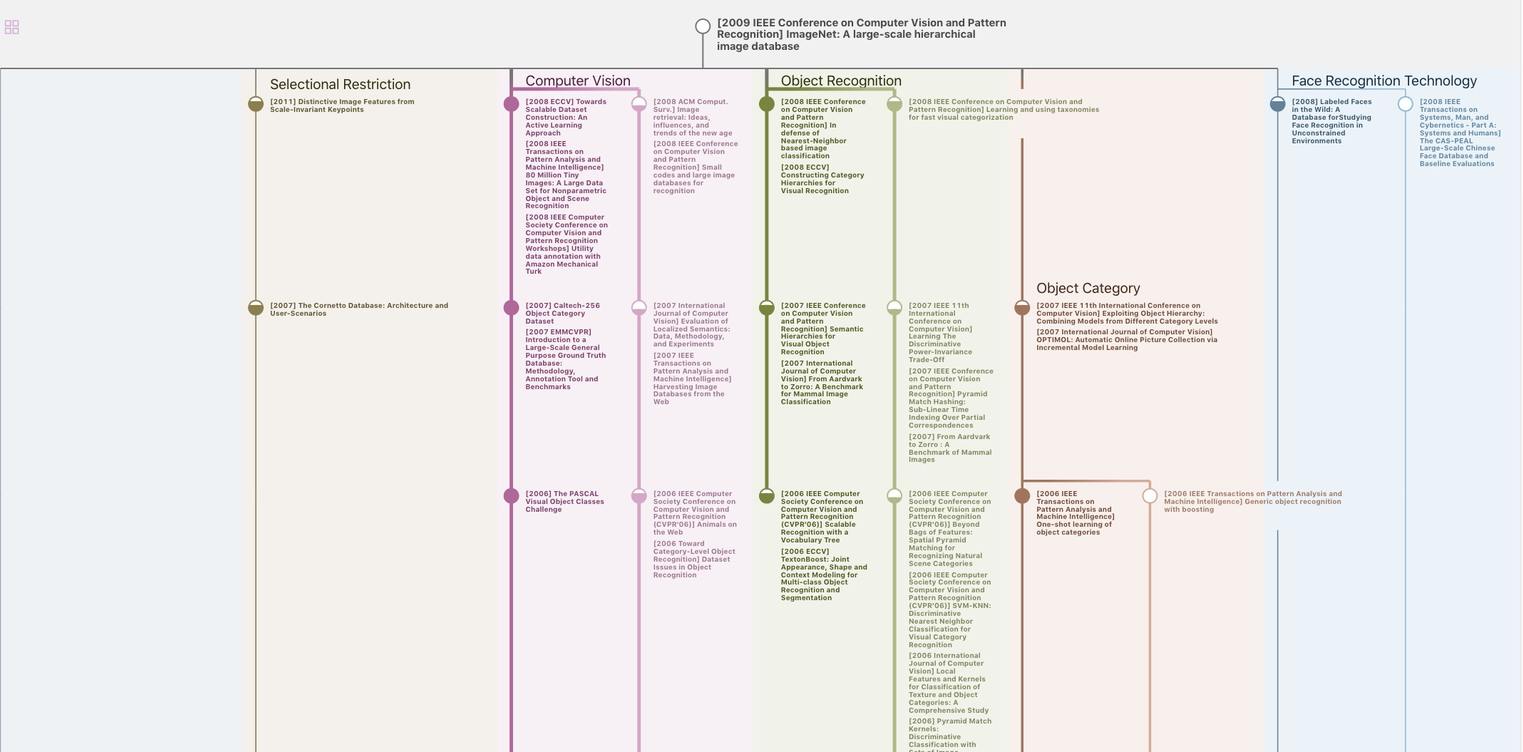
生成溯源树,研究论文发展脉络
Chat Paper
正在生成论文摘要