Global optimality in k-means clustering
Information Sciences(2018)
摘要
We study the problem of finding an optimum clustering, a problem known to be NP-hard. Existing literature contains algorithms running in time proportional to the number of points raised to a power that depends on the dimensionality and on the number of clusters. Published validations of some of these algorithms are unfortunately incomplete; besides, the constant factors (with respect to the number of points) in their running time bounds have seen several published important improvements but are still huge, exponential on the dimension and on the number of clusters, making the corresponding algorithms fully impractical. We provide a new algorithm, with its corresponding complexity-theoretic analysis. It reduces both the exponent and the constant factor, to the extent that it becomes feasible for relevant particular cases. Additionally, it parallelizes extremely well, so that its implementation on current high-performance hardware is quite straightforward. Our proposal opens the door to potential improvements along a research line that had no practical significance so far; besides, a long but single-shot run of our algorithm allows one to identify absolutely optimum solutions for benchmark problems, whereby alternative heuristic proposals can evaluate the goodness of their solutions and the precise price paid for their faster running times.
更多查看译文
关键词
Clustering,Voronoi diagrams,Cell arrangement
AI 理解论文
溯源树
样例
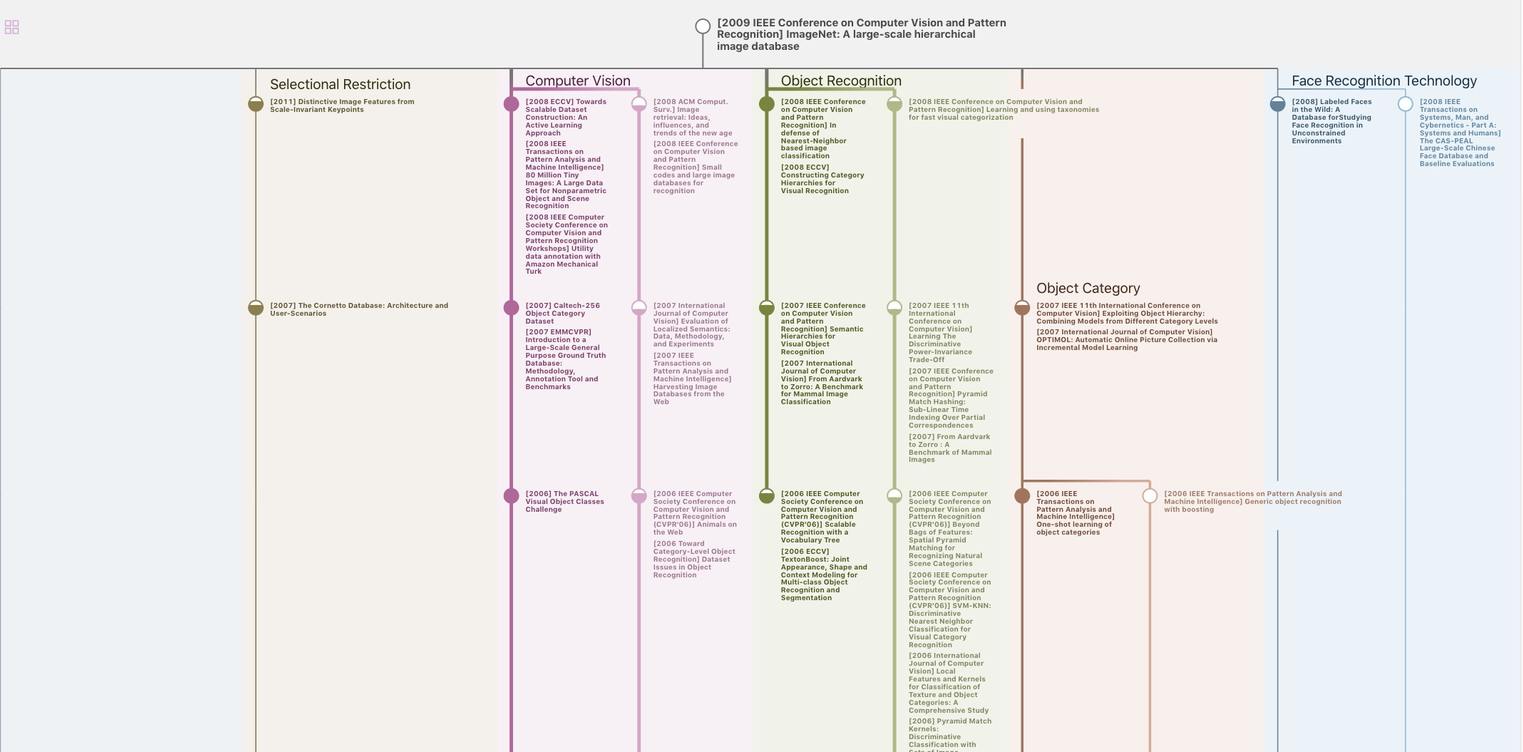
生成溯源树,研究论文发展脉络
Chat Paper
正在生成论文摘要