Spatial temporal flow dynamics prediction with large design space via data driven analysis and LES based surrogate model
ILASS-Americas 29th Annual Conference on Liquid Atomization and Spray Systems, Atlanta, GA, May 2017(2017)
摘要
This is an interdisciplinary study combining machine-learning techniques, statistics, and physics analyses. To develop a robust propulsion system efficiently, understandings of underlying physics, coupling, and conflicting of the design parameters are significantly important to achieve the optimal design. The large eddy simulation (LES) technique has been widely used to simulate flow physics and combustion characteristics inside rocket engines for decades; however, it consumes great amount of time and resources, which is impractical for design purposes. The purpose of this work is to predict spatial-temporal flowfields using data-driven surrogate model in a short turnaround time. Our previous study has demonstrated a novel design strategy based on the Kernel-smoothed proper orthogonal decomposition (KSPOD) for the swirl injector design. The instantaneous flow dynamics in swirl injectors were well predicted in a small geometry range via kriging based weighting function from design matrix. To further discuss the capability of the emulator for the instantaneous turbulent flow dynamics, the current work extends the design method to a large design space across RD-0110 and RD-170 engines. Overall, the emulator captures most of the flow dynamic details. It also well predicts the performance measurements such as the liquid film thickness and the spreading angle. At the same time, the turnaround time for evaluating a new design point is reduced significantly compared with other algorithms and the resultant CPU time is over 2,000 times shorter than that in the LES simulation.
更多查看译文
AI 理解论文
溯源树
样例
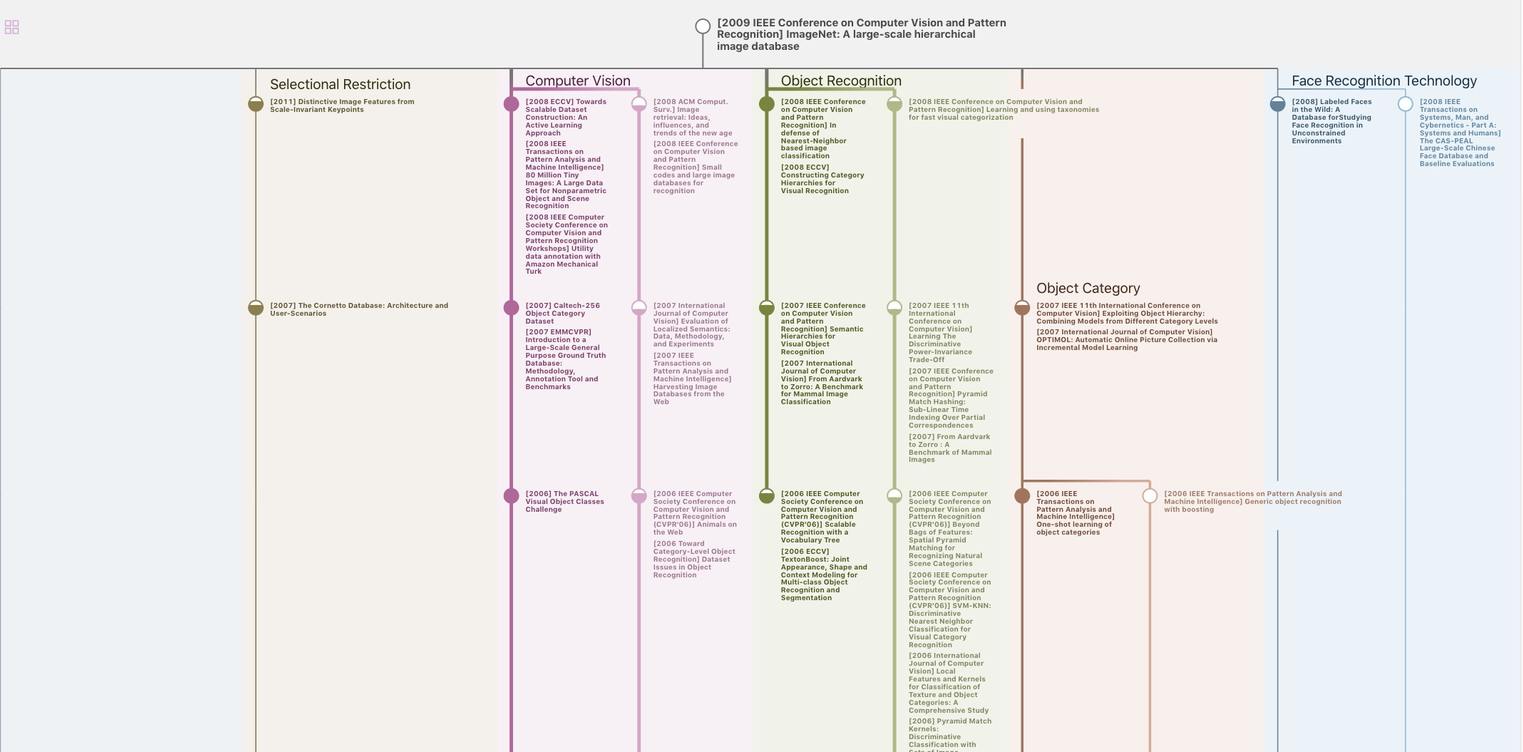
生成溯源树,研究论文发展脉络
Chat Paper
正在生成论文摘要