Distortion-Aware Monocular Depth Estimation for Omnidirectional Images
IEEE Signal Processing Letters(2021)
摘要
Image distortion is a main challenge for tasks on panoramas. In this work, we propose a Distortion-Aware Monocular Omnidirectional (DAMO) network to estimate dense depth maps from indoor panoramas. First, we introduce a distortion-aware module to extract semantic features from omnidirectional images. Specifically, we exploit deformable convolution to adjust its sampling grids to geometric distortions on panoramas. We also utilize a strip pooling module to sample against horizontal distortion introduced by inverse gnomonic projection. Second, we introduce a plug-and-play spherical-aware weight matrix for our loss function to handle the uneven distribution of areas projected from a sphere. Experiments on the 360D dataset show that the proposed method can effectively extract semantic features from distorted panoramas and alleviate the supervision bias caused by distortion. It achieves the state-of-the-art performance on the 360D dataset with high efficiency.
更多查看译文
关键词
Distortion,Convolution,Strips,Feature extraction,Estimation,Training,Kernel,Depth estimation,deformable convolution,omnidirectional images
AI 理解论文
溯源树
样例
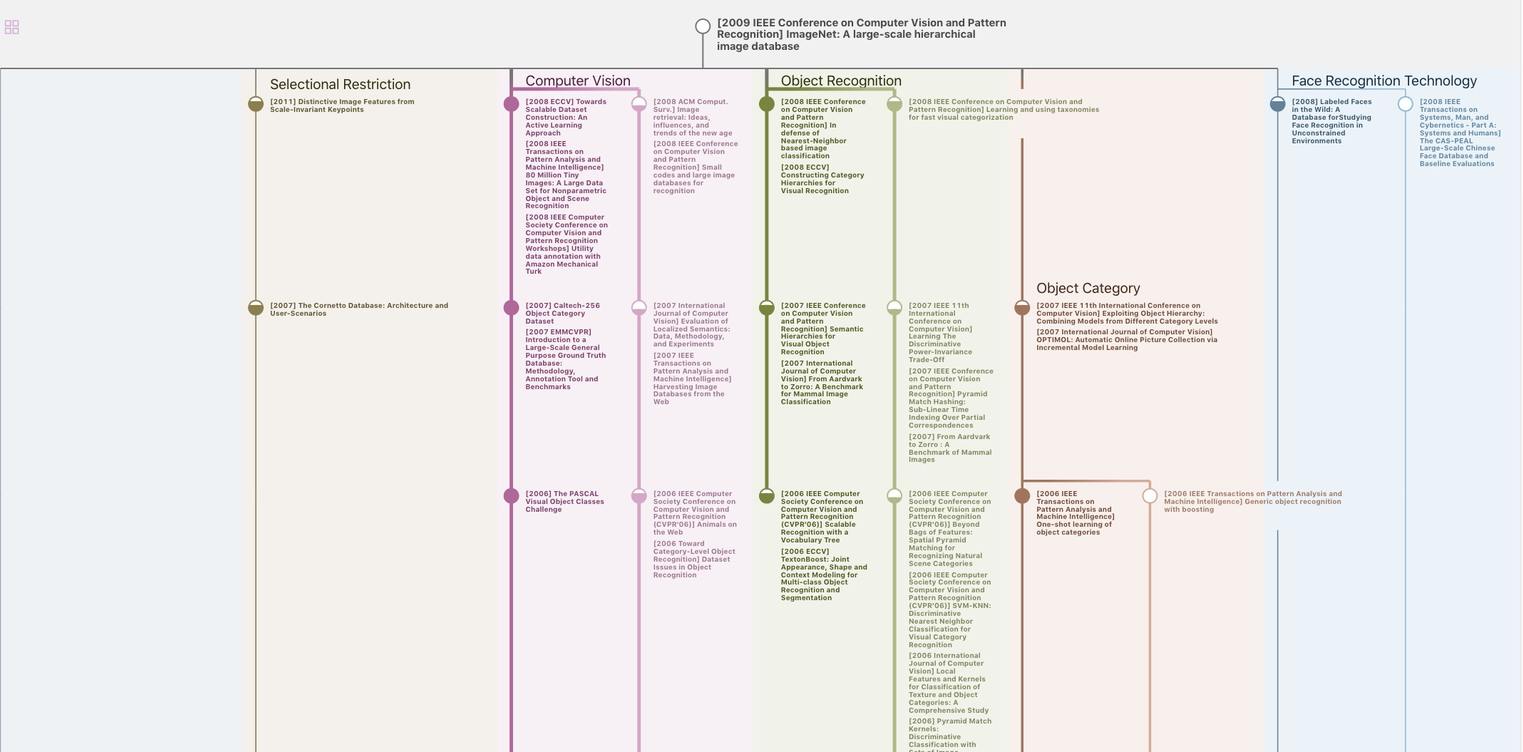
生成溯源树,研究论文发展脉络
Chat Paper
正在生成论文摘要