DeepQoE: Real-time Measurement of Video QoE from Encrypted Traffic with Deep Learning.
IEEE/ACM International Workshop on Quality of Service (IWQoS)(2020)CCF B
Beijing Inst Technol | Tsinghua Univ | Simon Fraser Univ | Temple Univ
Abstract
With the dramatic increase of video traffic on the Internet, video quality of experience (QoE) measurement becomes even more important, which provides network operators with an insight into the quality of their video delivery services. The widespread adoption of end-to-end encryption protocols such as SSL/TLS, however, sets a barrier to QoE monitoring as the most valuable indicators in cleartext traffic are no longer available after encryption. Existing studies on video QoE measurement in encrypted traffic support only coarse-grained QoE metrics or suffer from low accuracy. In this paper, we propose DeepQoE, a new approach that enables real-time video QoE measurement from encrypted traffic. We summarize critical fine-grained QoE metrics, including startup delay, rebuffering, and video resolutions. In order to achieve accurate and real-time inference of these metrics, we build DeepQoE by employing Convolutional Neural Networks (CNNs) with a sophisticated input and architecture design. More specifically, DeepQoE only leverages packet Round-Trip Time (RTT) in upstream traffic as its input. Evaluation results with real-world datasets collected from two popular content providers (i.e., YouTube and Bilibili) show that DeepQoE can improve QoE measurement accuracy by up to 22% over the state-of-the-art methods.
MoreTranslated text
Key words
Encrypted traffic analysis,video QoE,network measurement,deep learning,convolutional neural networks
求助PDF
上传PDF
View via Publisher
AI Read Science
AI Summary
AI Summary is the key point extracted automatically understanding the full text of the paper, including the background, methods, results, conclusions, icons and other key content, so that you can get the outline of the paper at a glance.
Example
Background
Key content
Introduction
Methods
Results
Related work
Fund
Key content
- Pretraining has recently greatly promoted the development of natural language processing (NLP)
- We show that M6 outperforms the baselines in multimodal downstream tasks, and the large M6 with 10 parameters can reach a better performance
- We propose a method called M6 that is able to process information of multiple modalities and perform both single-modal and cross-modal understanding and generation
- The model is scaled to large model with 10 billion parameters with sophisticated deployment, and the 10 -parameter M6-large is the largest pretrained model in Chinese
- Experimental results show that our proposed M6 outperforms the baseline in a number of downstream tasks concerning both single modality and multiple modalities We will continue the pretraining of extremely large models by increasing data to explore the limit of its performance
Upload PDF to Generate Summary
Must-Reading Tree
Example
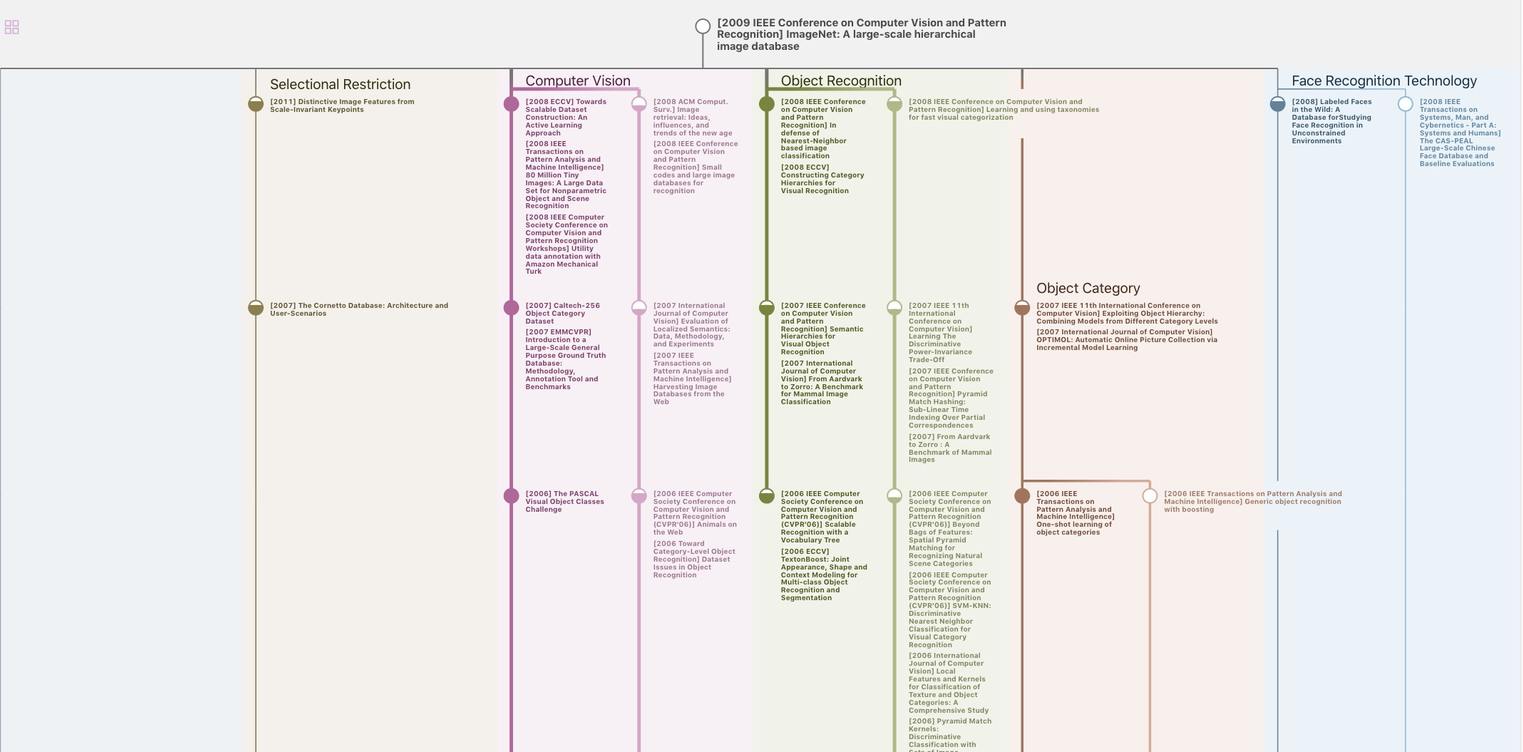
Generate MRT to find the research sequence of this paper
Related Papers
2014
被引用1148 | 浏览
2013
被引用30 | 浏览
2013
被引用143 | 浏览
2011
被引用41 | 浏览
2017
被引用171 | 浏览
2017
被引用109 | 浏览
2019
被引用25 | 浏览
2020
被引用57 | 浏览
Data Disclaimer
The page data are from open Internet sources, cooperative publishers and automatic analysis results through AI technology. We do not make any commitments and guarantees for the validity, accuracy, correctness, reliability, completeness and timeliness of the page data. If you have any questions, please contact us by email: report@aminer.cn
Chat Paper