Single Fundus Image Super-Resolution Via Cascaded Channel-Wise Attention Network.
42ND ANNUAL INTERNATIONAL CONFERENCES OF THE IEEE ENGINEERING IN MEDICINE AND BIOLOGY SOCIETY ENABLING INNOVATIVE TECHNOLOGIES FOR GLOBAL HEALTHCARE EMBC'20(2020)
摘要
Fundus image is commonly used in aiding the diagnosis of ophthalmic diseases. A high-resolution (HR) image is valuable to provide the anatomic information on the eye conditions. Recently, image super-resolution (SR) though learning model has been shown to be an economic yet effective way to satisfy the high demands in the clinical practice. However, the reported methods ignore the mutual dependencies of low-and high-resolution images and did not fully exploit the dependencies between channels. To tackle with the drawbacks, we propose a novel network for fundus image SR, named by Fundus Cascaded Channel-wise Attention Network (FC-CAN). The proposed FCCAN cascades channel attention module and dense module jointly to exploit the semantic interdependencies both frequency and domain information across channels. The channel attention module rescales channel maps in spatial domain, while the dense module preserves the HR components by up- and down-sampling operation. Experimental results demonstrate the superiority of our net-work in comparison with the six methods.
更多查看译文
关键词
deep learning,super-resolution,fundus image,channel-wise attention
AI 理解论文
溯源树
样例
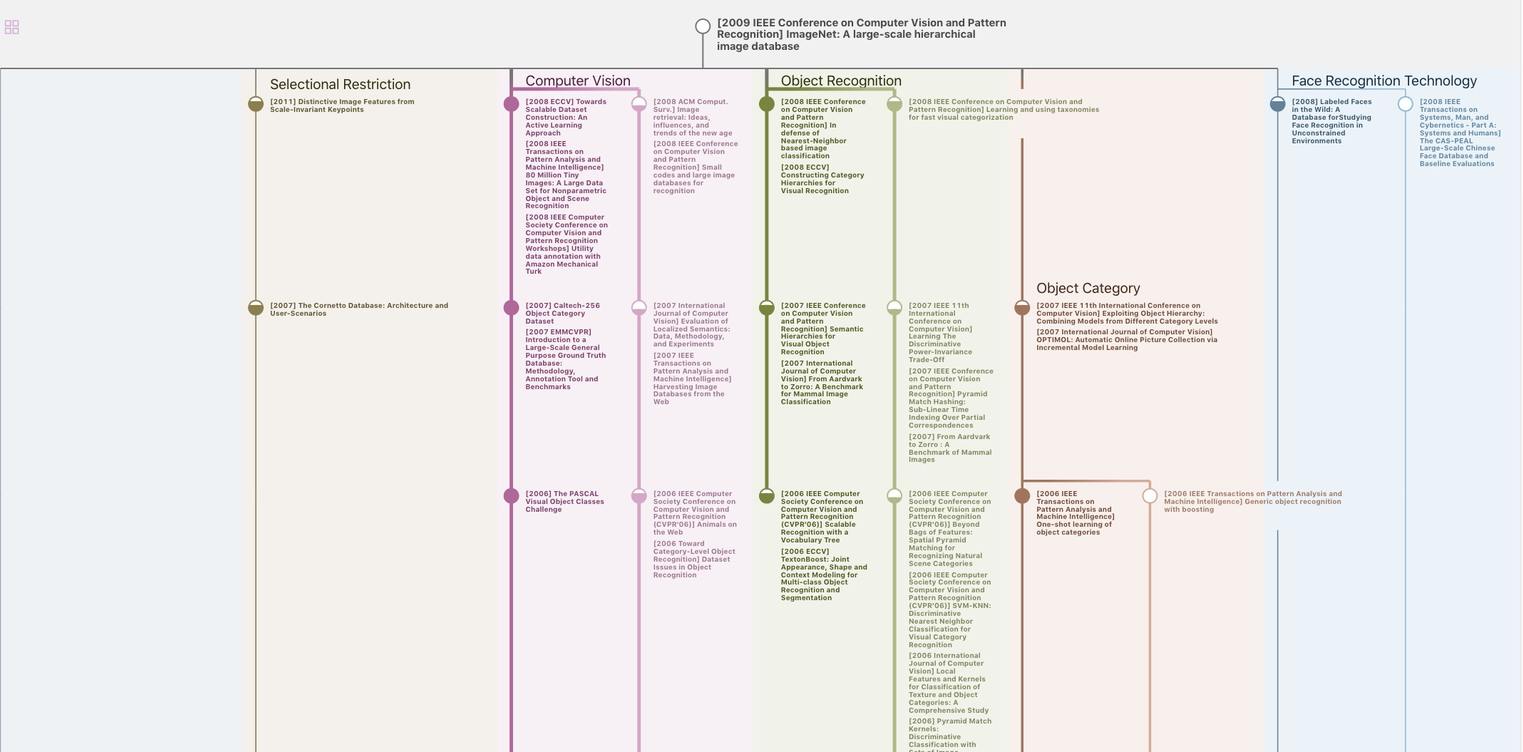
生成溯源树,研究论文发展脉络
Chat Paper
正在生成论文摘要