Highly Uniform Two-Terminal Artificial Synapses Based on Polycrystalline Hf(0.5)Zr(0.5)O(2)for Sparsified Back Propagation Networks
ADVANCED ELECTRONIC MATERIALS(2020)
摘要
Artificial neural networks with nonvolatile memristors as synapses represent an in-memory computing paradigm, where the online training of networks with rapidly growing scale places high requirements on device uniformity and endurance as well as training time and energy. Here, a highly uniform two-terminal Hf(0.5)Zr(0.5)O(2)based artificial synapse is reported, which shows 4-bit weight precision, cycle-to-cycle variation (sigma/mu) of <4%, device-to-device variation (sigma/mu) of <9%, retention of >10(4) s at 85 degrees C, and endurance of >10(6). The improved uniformity can be attributed to the nonfilamentary resistive switching mechanism mediated by ion exchange at the interface. A holistic optimization using such reliable synapses and modified algorithm with sparsified back propagation can accelerate training by 166 times, decrease the energy consumption by 83 times, reduce the total updating rate by 138 times in multilayer perceptron, and the approach can be extended to convolutional neural networks as well. The synergistically optimized approach thus paves the way for the construction of memristor based systems capable of learning and interacting adaptively.
更多查看译文
关键词
hafnium zirconium oxide,in-memory computing,memristors,online learning,sparsified back propagation
AI 理解论文
溯源树
样例
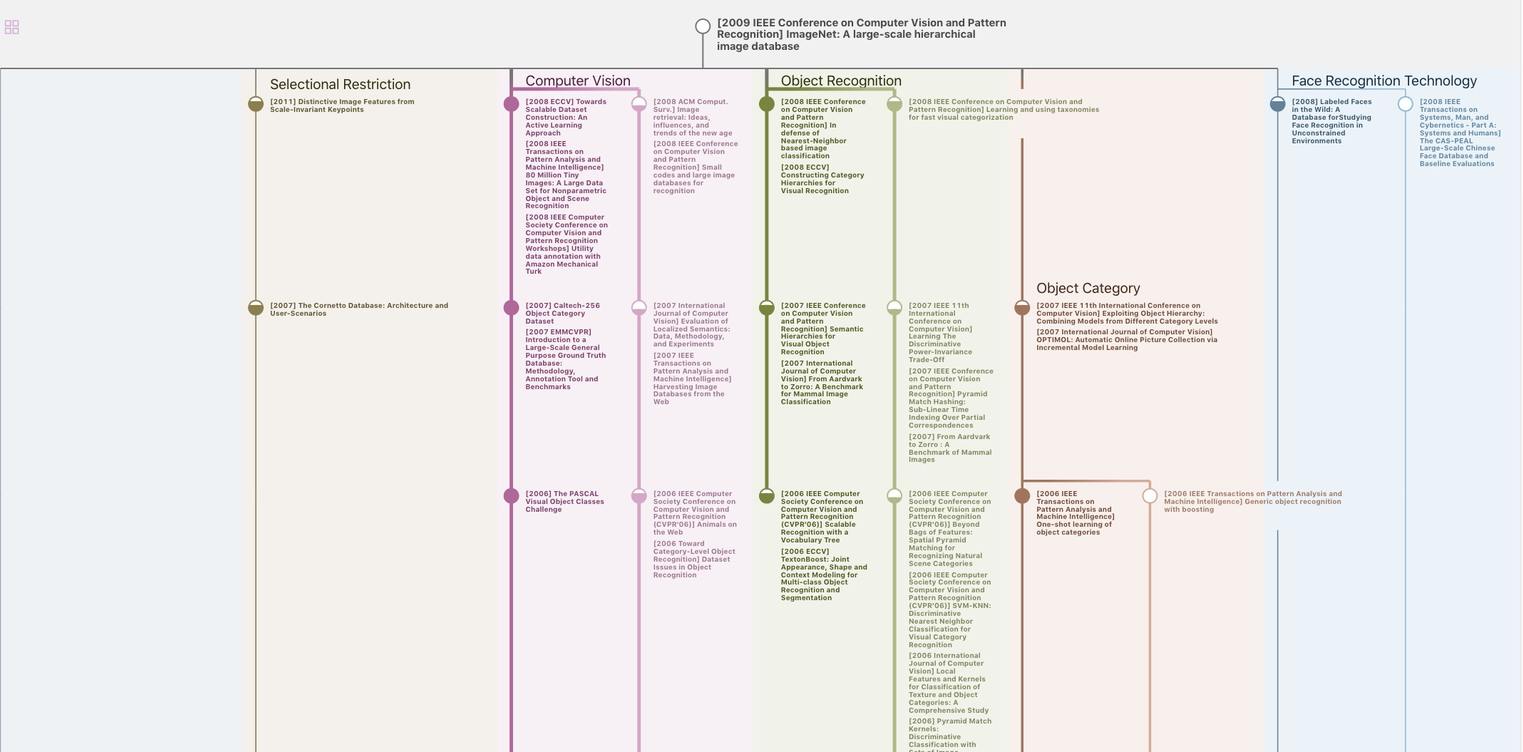
生成溯源树,研究论文发展脉络
Chat Paper
正在生成论文摘要