Pre‐clerkship Physical Examination Assessment Rubric
Morsani Coll Med | Univ S Florida
Abstract
INTRODUCTION:The physical examination is a core competency in the training of pre-clerkship medical students. It is important to certify proficiency in the physical examination before students start their clinical rotations. Many institutions use home grown assessment tools for this purpose; however, there currently are no validated rubrics designed to assess the performance a head to toe physical examination by a pre-clerkship medical student. The goal of this study is to assess the reliability (inter-rater and intra-rater) of our institutionally developed rubric.METHODS:Clinical faculty with various levels of teaching experience watched videos of students doing a head to toe physical examination and scored the students using our assessment rubric. These scores were evaluated for intra-rater and inter-rater reliability.RESULTS:A total of 15 student videos were reviewed by five faculty members with varying levels of teaching experience. The degree of inter-rater agreement (between raters) for single and average measure was excellent and the degree of intra-rater agreement (same rater twice) for single and average measure was excellent.DISCUSSION:We conclude that our institutionally developed physical examination assessment rubric is a reliable means to certify proficiency in the physical examination before students start their clinical clerkships.
MoreTranslated text
求助PDF
上传PDF
View via Publisher
AI Read Science
AI Summary
AI Summary is the key point extracted automatically understanding the full text of the paper, including the background, methods, results, conclusions, icons and other key content, so that you can get the outline of the paper at a glance.
Example
Background
Key content
Introduction
Methods
Results
Related work
Fund
Key content
- Pretraining has recently greatly promoted the development of natural language processing (NLP)
- We show that M6 outperforms the baselines in multimodal downstream tasks, and the large M6 with 10 parameters can reach a better performance
- We propose a method called M6 that is able to process information of multiple modalities and perform both single-modal and cross-modal understanding and generation
- The model is scaled to large model with 10 billion parameters with sophisticated deployment, and the 10 -parameter M6-large is the largest pretrained model in Chinese
- Experimental results show that our proposed M6 outperforms the baseline in a number of downstream tasks concerning both single modality and multiple modalities We will continue the pretraining of extremely large models by increasing data to explore the limit of its performance
Upload PDF to Generate Summary
Must-Reading Tree
Example
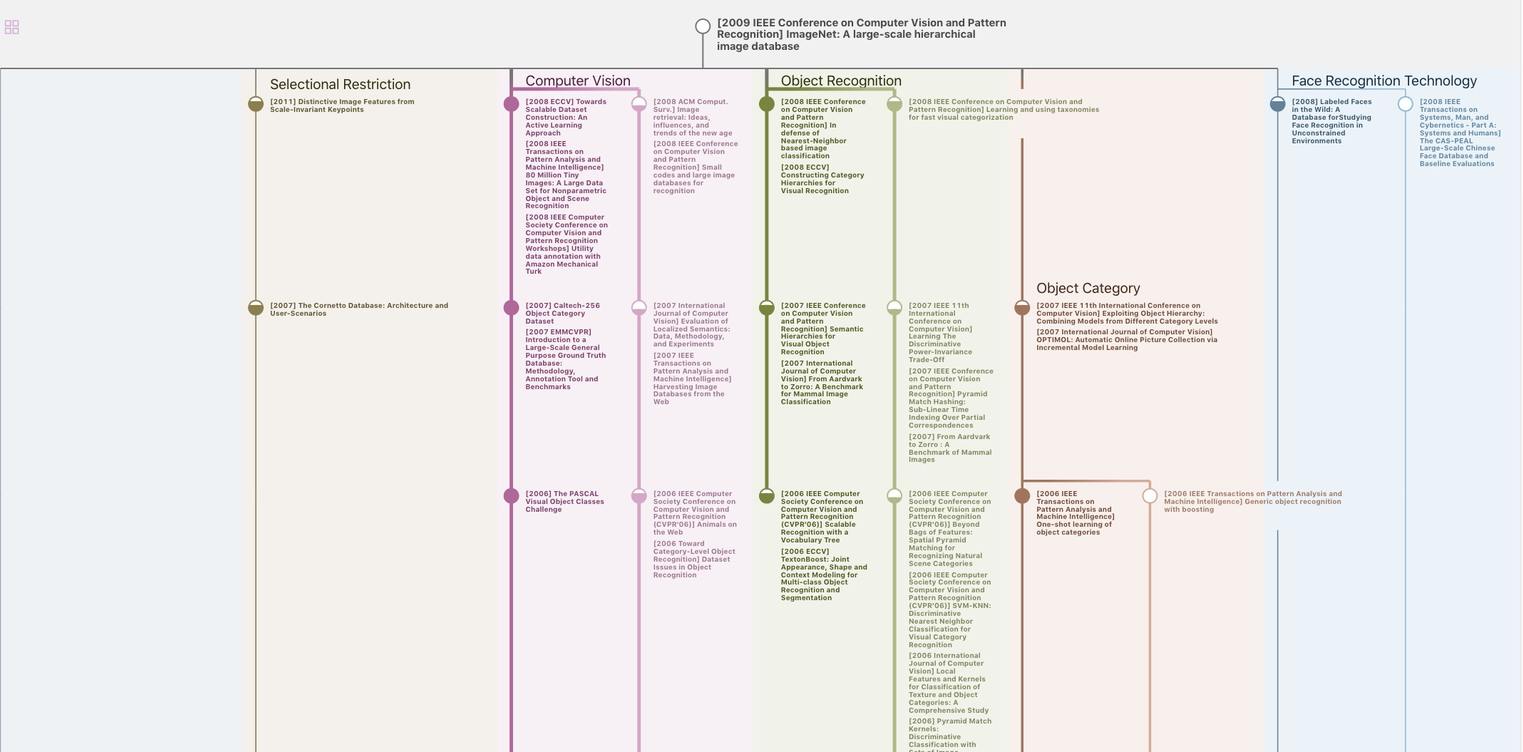
Generate MRT to find the research sequence of this paper
Related Papers
Education of Chinese Medicine 2023
被引用0
Data Disclaimer
The page data are from open Internet sources, cooperative publishers and automatic analysis results through AI technology. We do not make any commitments and guarantees for the validity, accuracy, correctness, reliability, completeness and timeliness of the page data. If you have any questions, please contact us by email: report@aminer.cn
Chat Paper
GPU is busy, summary generation fails
Rerequest