A Novel Pathogenic Variant in DYNC1H1 Causes Various Upper and Lower Motor Neuron Anomalies.
European Journal of Medical Genetics(2020)SCI 4区
Univ Utah | ARUP Labs | Hop Bretonneau | Hop Charles Nicolle | Hop Mere & Enfant | Hop Dupuytren | CHU Tours | Hop Pellegrin | Ctr Hosp La Cote Basque | CEA | Hop Necker Enfants Malad | Hop La Pitie Salpetriere | Hop Cochin | GeneDx
Abstract
Objective: To perform genotype-phenotype, clinical and molecular analysis in a large 3-generation family with autosomal dominant congenital spinal muscular atrophy. Methods: Using a combined genetic approach including whole genome scanning, next generation sequencing-based multigene panel, whole genome sequencing, and targeted variant Sanger sequencing, we studied the proband and multiple affected individuals of this family who presented bilateral proximal lower limb muscle weakness and atrophy. Results: We identified a novel heterozygous variant, c.1826T > C; p.Ile609Thr, in the DYNC1H1 gene localized within the common haplotype in the 14q32.3 chromosomal region which cosegregated with disease in this large family. Within the family, affected individuals were found to have a wide array of clinical variability. Although some individuals presented the typical lower motor neuron phenotype with areflexia and denervation, others presented with muscle weakness and atrophy, hyperreflexia, and absence of denervation suggesting a predominant upper motor neuron disease. In addition, some affected individuals presented with an intermediate phenotype characterized by hyperreflexia and denervation, expressing a combination of lower and upper motor neuron defects. Conclusion: Our study demonstrates the wide clinical variability associated with a single disease causing variant in DYNC1H1 gene and this variant demonstrated a high penetrance within this large family.
MoreTranslated text
Key words
DYNC1H1,Spinal muscular atrophies,Exome,Next generation sequencing
PDF
View via Publisher
AI Read Science
AI Summary
AI Summary is the key point extracted automatically understanding the full text of the paper, including the background, methods, results, conclusions, icons and other key content, so that you can get the outline of the paper at a glance.
Example
Background
Key content
Introduction
Methods
Results
Related work
Fund
Key content
- Pretraining has recently greatly promoted the development of natural language processing (NLP)
- We show that M6 outperforms the baselines in multimodal downstream tasks, and the large M6 with 10 parameters can reach a better performance
- We propose a method called M6 that is able to process information of multiple modalities and perform both single-modal and cross-modal understanding and generation
- The model is scaled to large model with 10 billion parameters with sophisticated deployment, and the 10 -parameter M6-large is the largest pretrained model in Chinese
- Experimental results show that our proposed M6 outperforms the baseline in a number of downstream tasks concerning both single modality and multiple modalities We will continue the pretraining of extremely large models by increasing data to explore the limit of its performance
Try using models to generate summary,it takes about 60s
Must-Reading Tree
Example
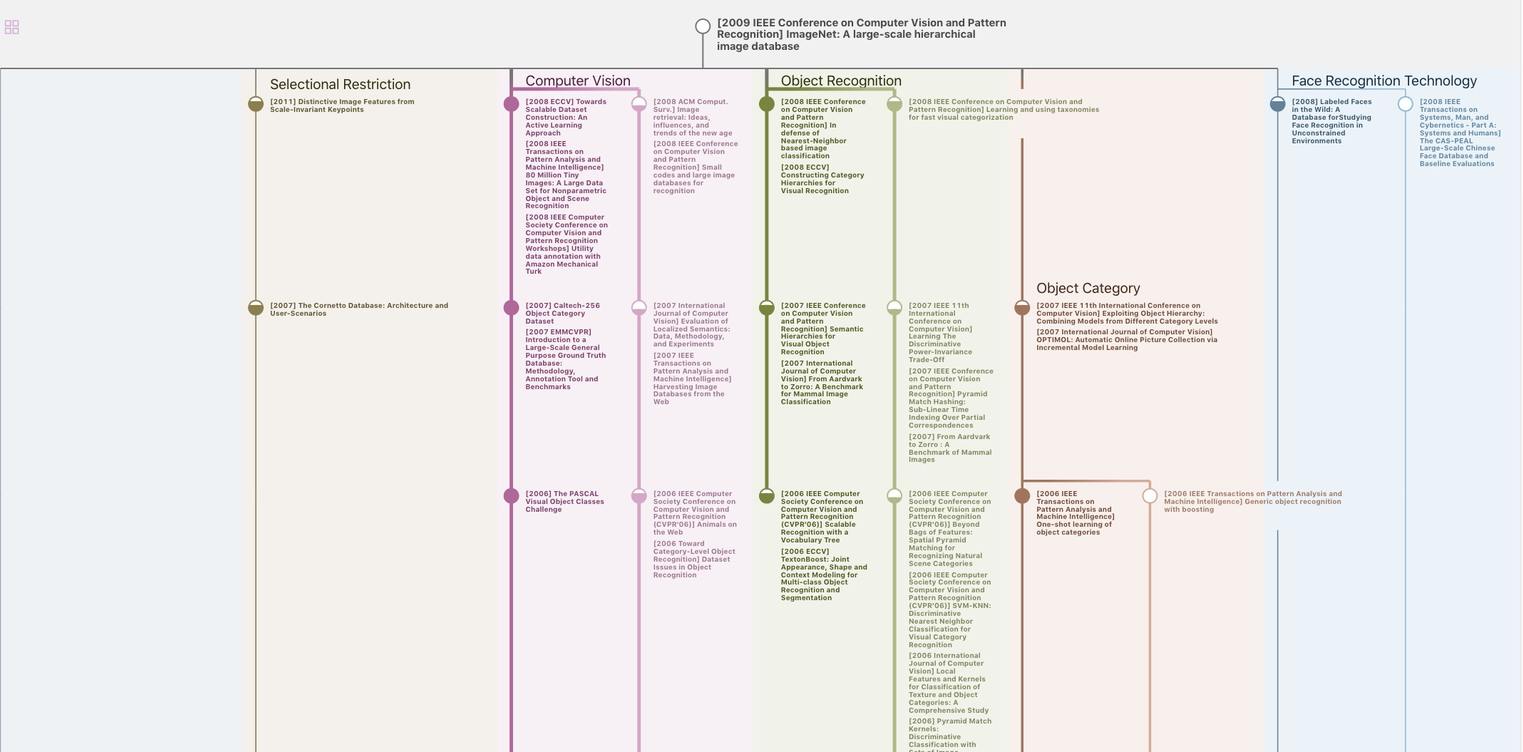
Generate MRT to find the research sequence of this paper
Related Papers
Child Neurology Open 2021
被引用1
Molecular Case Studies 2021
被引用8
Neurological Sciences 2022
被引用1
Frontiers in Neurology 2023
被引用0
Cargo Specificity, Regulation, and Therapeutic Potential of Cytoplasmic Dynein
Experimental & Molecular Medicine 2024
被引用0
Data Disclaimer
The page data are from open Internet sources, cooperative publishers and automatic analysis results through AI technology. We do not make any commitments and guarantees for the validity, accuracy, correctness, reliability, completeness and timeliness of the page data. If you have any questions, please contact us by email: report@aminer.cn
Chat Paper
去 AI 文献库 对话