A Time-Phased Machine Learning Model for Real-Time Prediction of Sepsis in Critical Care
Critical care medicine(2020)
摘要
Objectives: As a life-threatening condition, sepsis is one of the major public health issues worldwide. Early prediction can improve sepsis outcomes with appropriate interventions. With the PhysioNet/Computing in Cardiology Challenge 2019, we aimed to develop and validate a machine learning algorithm with high prediction performance and clinical interpretability for prediction of sepsis onset during critical care in real-time. Design: Retrospective observational cohort study. Setting: The dataset was collected from three ICUs in three different U.S. hospitals. Two of them were publicly available for model development (offline) and one was used for testing (online). Patients: Forty-thousand three-hundred thirty-six ICU patients from the two model development databases and 24,819 from the test database. There are up to 40 hourly-recorded clinical variables for each ICU stay. The Sepsis-3 criteria were used to confirm sepsis onset. Interventions: None. Measurements and Main Results: Three-hundred twelve features were constructed hourly as the input of our proposed Time-phAsed machine learning model for Sepsis Prediction. Time-phAsed machine learning model for Sepsis Prediction first estimates the likelihood of sepsis onset for each hour of an ICU stay in the following 6 hours, and then makes a binary prediction with three time-phased cutoff values. On the internal validation set, the utility score (official challenge measurement) achieved by Time-phAsed machine learning model for Sepsis Prediction was 0.430. On the test set, the utility score reached was 0.354. Furthermore, Time-phAsed machine learning model for Sepsis Prediction provides an intuitive way to illustrate the impact of the input features on the outcome prediction, which makes it clinically interpretable. Conclusions: The proposed Time-phAsed machine learning model for Sepsis Prediction model is accurate and interpretable for real-time prediction of sepsis onset in critical care, which holds great potential for further evaluation in prospective studies.
更多查看译文
关键词
clinical interpretability,competition,machine learning,real-time prediction,sepsis,time-phased strategy
AI 理解论文
溯源树
样例
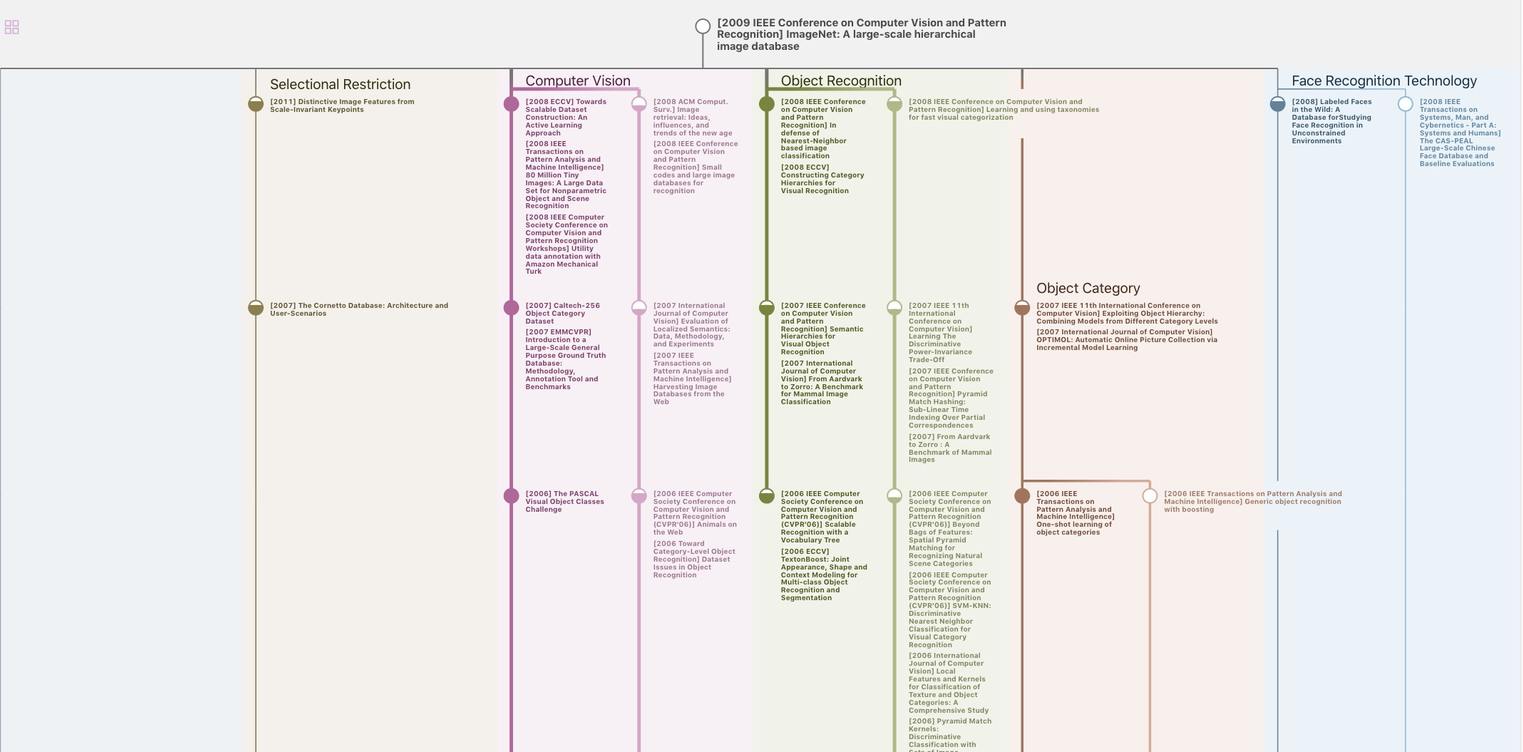
生成溯源树,研究论文发展脉络
Chat Paper
正在生成论文摘要