Dynamic Batch Learning in High-Dimensional Sparse Linear Contextual Bandits
MANAGEMENT SCIENCE(2024)
摘要
We study the problem of dynamic batch learning in high-dimensional sparse linear contextual bandits, where a decision maker, under a given maximum-number-of batch constraint and only able to observe rewards at the end of each batch, can dynamically decide how many individuals to include in the next batch (at the end of the current batch) and what personalized action-selection scheme to adopt within each batch. Such batch constraints are ubiquitous in a variety of practical contexts, including personalized product offerings in marketing and medical treatment selection in clinical trials. We characterize the fundamental learning limit in this problem via a regret lower bound and provide a matching upper bound (up to log factors), thus prescribing an optimal scheme for this problem. To the best of our knowledge, our work provides the first inroad into a theoretical understanding of dynamic batch learning in high-dimensional sparse linear contextual bandits. Notably, even a special case of our result-when no batch constraint is present- yields that the simple exploration-free algorithm using the LASSO estimator already achieves the minimax optimal O similar to( root ffiffiffiffiffififfi ) regret bound (s0 is the sparsity parameter or an s0T upper bound thereof and T is the learning horizon) for standard online learning in high dimensional linear contextual bandits (for the no-margin case), a result that appears unknown in the emerging literature of high-dimensional contextual bandits.
更多查看译文
关键词
dynamic batch learning,LASSO,high-dimensional statistics,contextual bandits,sparsity
AI 理解论文
溯源树
样例
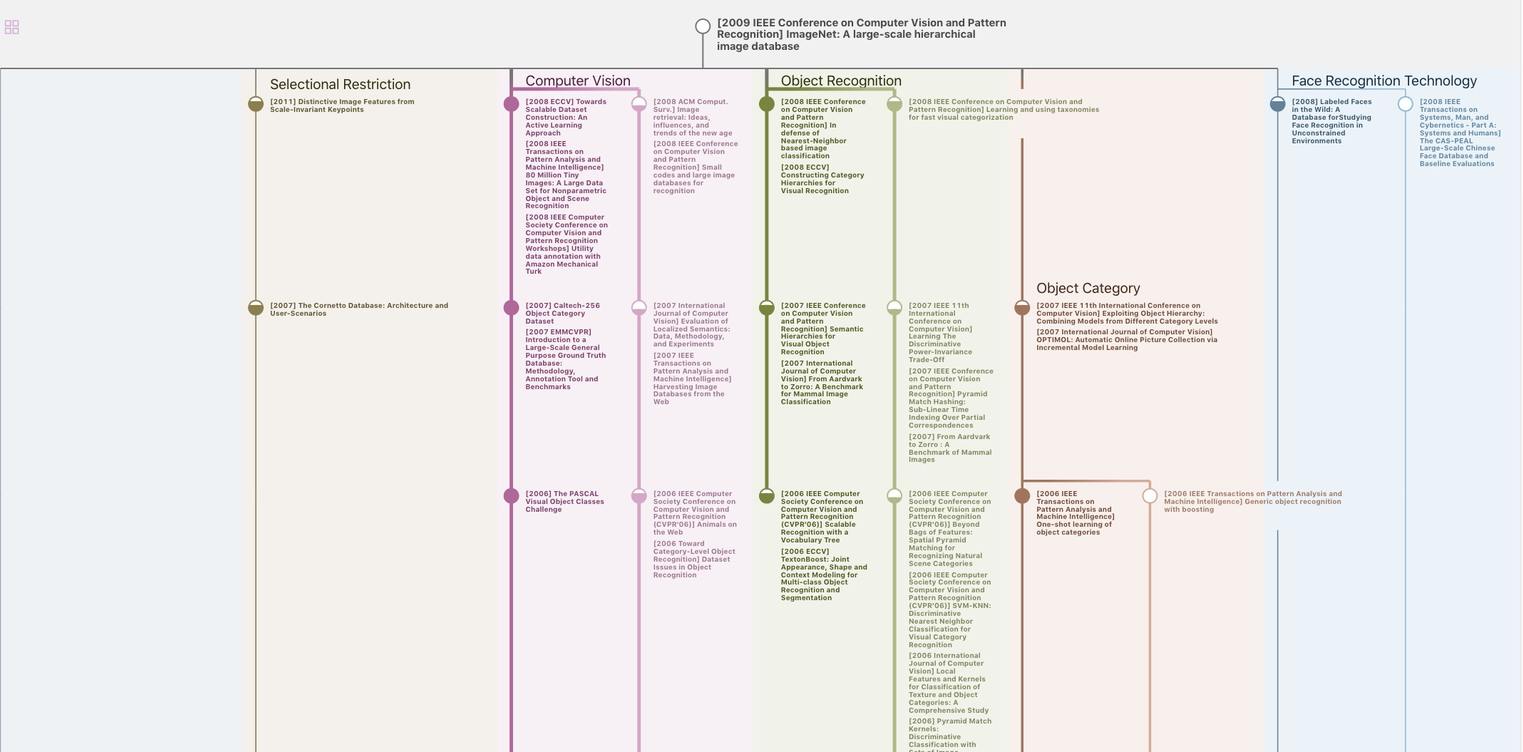
生成溯源树,研究论文发展脉络
Chat Paper
正在生成论文摘要