Perceptions, Knowledge, and Behaviors Related to COVID-19 among Social Media Users: Cross-Sectional Study
Journal of medical Internet research(2020)SCI 2区
Med Univ Bahrain | Kuwait Univ | Clin Dent Ctr
Abstract
Background Social media is one of the most rapid and impactful ways of obtaining and delivering information in the modern era. Objective The aim of this study was to rapidly obtain information on public perceptions, knowledge, and behaviors related to COVID-19 in order to identify deficiencies in key areas of public education. Methods Using a cross-sectional study design, a survey web link was posted on the social media and messaging platforms Instagram, Twitter, and WhatsApp by the study investigators. Participants, aged ≥18 years, filled out the survey on a voluntary basis. The main outcomes measured were knowledge of COVID-19 symptoms, protective measures against COVID-19, and source(s) of information about COVID-19. Subgroup analyses were conducted to determine the effects of age, gender, underlying illness, and working or studying in the health care industry on the perceived likelihood of acquiring COVID-19 and getting vaccinated. Results A total of 5677 subjects completed the survey over the course of 1 week. “Fever or chills” (n=4973, 87.6%) and “shortness of breath” (n=4695, 82.7%) were identified as the main symptoms of COVID-19. Washing and sanitizing hands (n=4990, 87.9%) and avoiding public places and crowds (n=4865, 85.7%) were identified as the protective measures most frequently used against COVID-19. Social media was the most utilized source for information on the disease (n=4740, 83.5%), followed by the World Health Organization (n=2844, 50.1%). Subgroup analysis revealed that younger subjects (<35 years), males, and those working or studying in health care reported a higher perceived likelihood of acquiring COVID-19, whereas older subjects, females, and those working or studying in non–health care areas reported a lower perceived likelihood of acquiring COVID-19. Similar trends were observed for vaccination against COVID-19, with older subjects, females, and those working or studying in non–health care sectors reporting a lower likelihood of vaccinating against COVID-19. Conclusions Our results are indicative of a relatively well-informed cohort implementing appropriate protective measures. However, key knowledge deficiencies exist with regards to vaccination against COVID-19, which future efforts should aim at correcting.
MoreTranslated text
Key words
COVID-19,social media,public health,perception,knowledge,health information,health education,virus
PDF
View via Publisher
AI Read Science
AI Summary
AI Summary is the key point extracted automatically understanding the full text of the paper, including the background, methods, results, conclusions, icons and other key content, so that you can get the outline of the paper at a glance.
Example
Background
Key content
Introduction
Methods
Results
Related work
Fund
Key content
- Pretraining has recently greatly promoted the development of natural language processing (NLP)
- We show that M6 outperforms the baselines in multimodal downstream tasks, and the large M6 with 10 parameters can reach a better performance
- We propose a method called M6 that is able to process information of multiple modalities and perform both single-modal and cross-modal understanding and generation
- The model is scaled to large model with 10 billion parameters with sophisticated deployment, and the 10 -parameter M6-large is the largest pretrained model in Chinese
- Experimental results show that our proposed M6 outperforms the baseline in a number of downstream tasks concerning both single modality and multiple modalities We will continue the pretraining of extremely large models by increasing data to explore the limit of its performance
Try using models to generate summary,it takes about 60s
Must-Reading Tree
Example
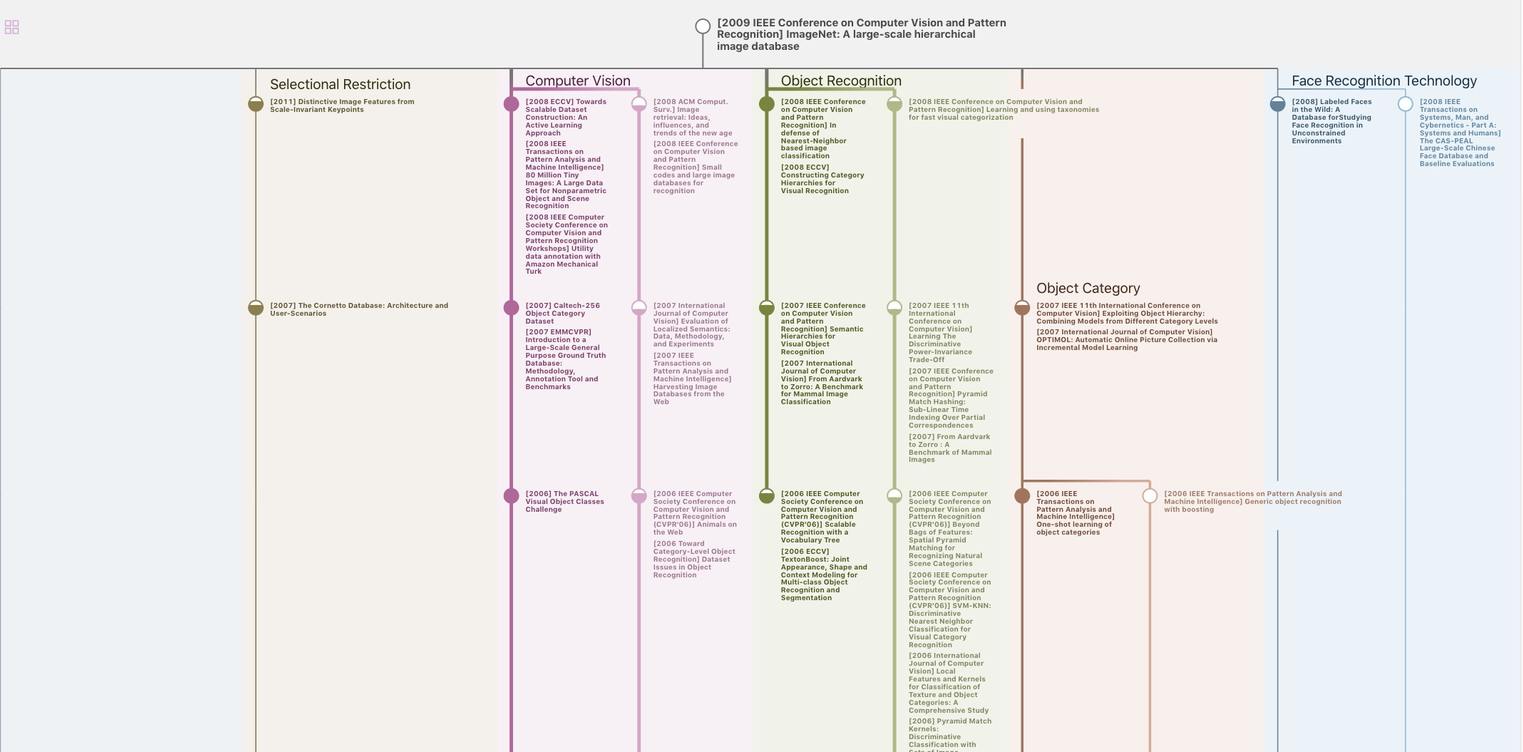
Generate MRT to find the research sequence of this paper
Related Papers
2017
被引用13758 | 浏览
2020
被引用28459 | 浏览
2020
被引用177 | 浏览
2020
被引用79 | 浏览
2020
被引用70 | 浏览
Data Disclaimer
The page data are from open Internet sources, cooperative publishers and automatic analysis results through AI technology. We do not make any commitments and guarantees for the validity, accuracy, correctness, reliability, completeness and timeliness of the page data. If you have any questions, please contact us by email: report@aminer.cn
Chat Paper
去 AI 文献库 对话