Sample-efficient Cross-Entropy Method for Real-time Planning.
CoRL(2020)
摘要
Trajectory optimizers for model-based reinforcement learning, such as the Cross-Entropy Method (CEM), can yield compelling results even in high-dimensional control tasks and sparse-reward environments. However, their sampling inefficiency prevents them from being used for real-time planning and control. We propose an improved version of the CEM algorithm for fast planning, with novel additions including temporally-correlated actions and memory, requiring 2.7-22x less samples and yielding a performance increase of 1.2-10x in high-dimensional control problems.
更多查看译文
关键词
planning,sample-efficient,cross-entropy,real-time
AI 理解论文
溯源树
样例
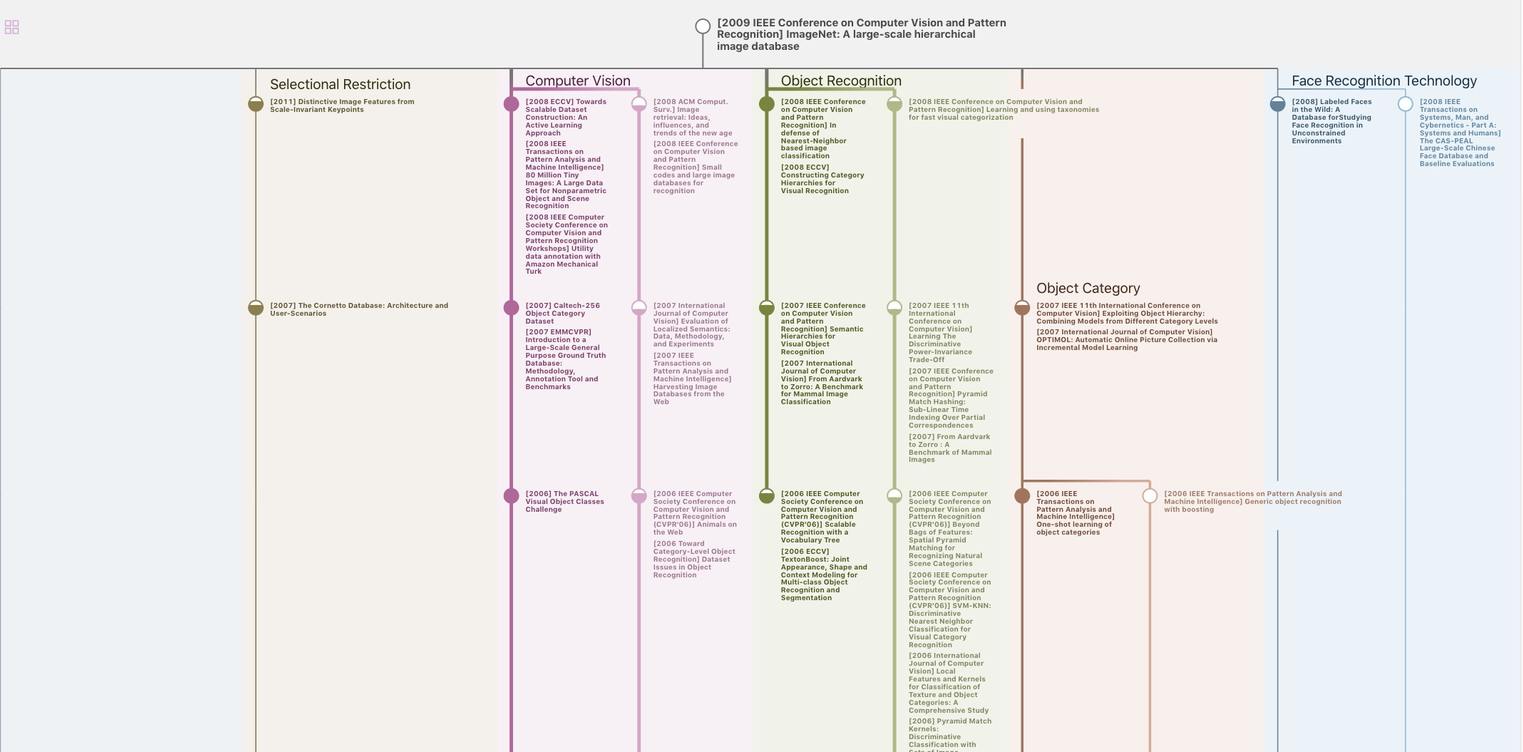
生成溯源树,研究论文发展脉络
Chat Paper
正在生成论文摘要