Imitative Planning using Conditional Normalizing Flow
arXiv (Cornell University)(2022)
摘要
A popular way to plan trajectories in dynamic urban scenarios for Autonomous Vehicles is to rely on explicitly specified and hand crafted cost functions, coupled with random sampling in the trajectory space to find the minimum cost trajectory. Such methods require a high number of samples to find a low-cost trajectory and might end up with a highly suboptimal trajectory given the planning time budget. We explore the application of normalizing flows for improving the performance of trajectory planning for autonomous vehicles (AVs). Our key insight is to learn a sampling policy in a low-dimensional latent space of expert-like trajectories, out of which the best sample is selected for execution. By modeling the trajectory planner's cost manifold as an energy function, we learn a scene conditioned mapping from the prior to a Boltzmann distribution over the AV control space. Finally, we demonstrate the effectiveness of our approach on real-world datasets over IL and hand-constructed trajectory sampling techniques.
更多查看译文
关键词
conditional normalizing flow,planning
AI 理解论文
溯源树
样例
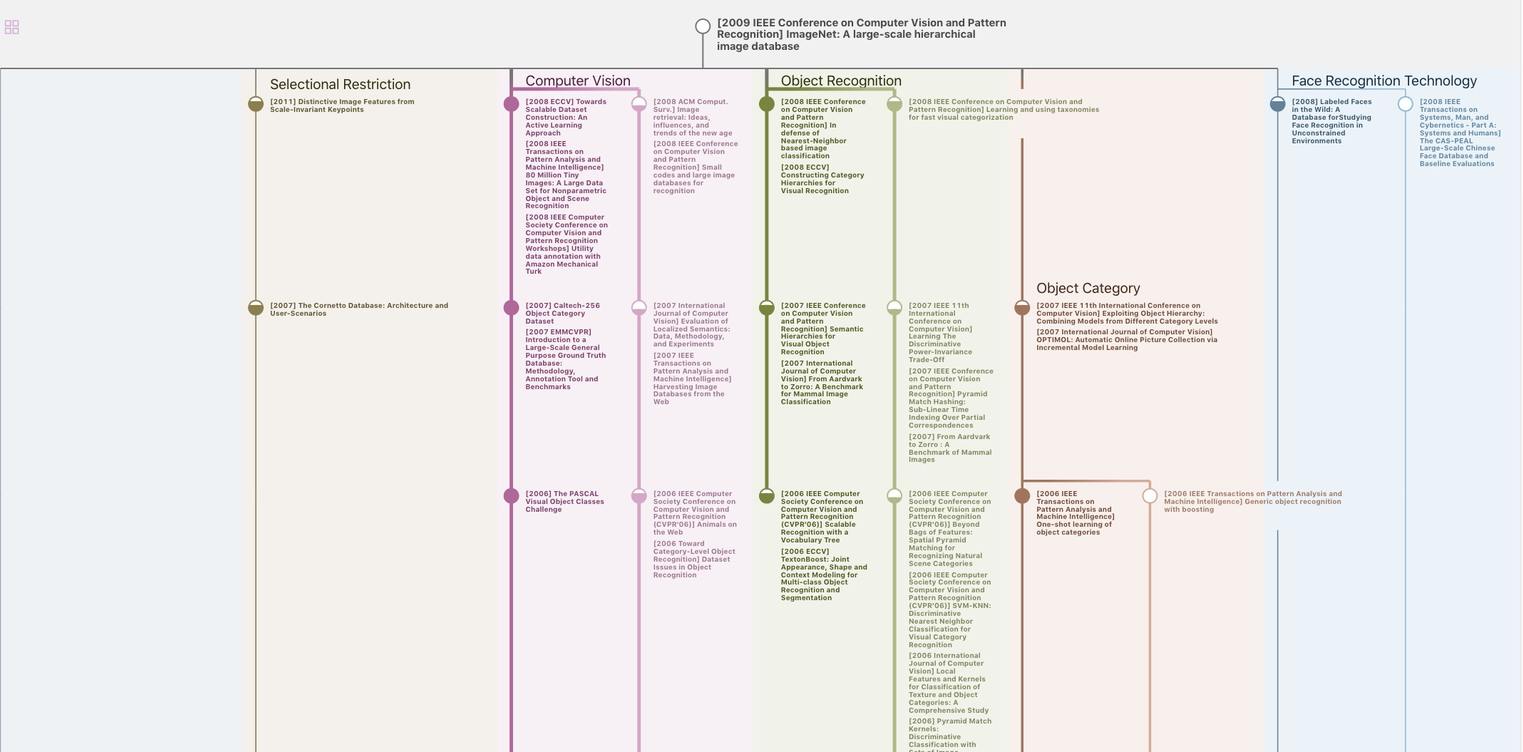
生成溯源树,研究论文发展脉络
Chat Paper
正在生成论文摘要