Prediction Model and Risk Scores of ICU Admission and Mortality in COVID-19
PLoS ONE(2020)SCI 3区
Abstract
This study aimed to develop risk scores based on clinical characteristics at presentation to predict intensive care unit (ICU) admission and mortality in COVID-19 patients. 641 hospitalized patients with laboratory-confirmed COVID-19 were selected from 4997 persons under investigation. We performed a retrospective review of medical records of demographics, comorbidities and laboratory tests at the initial presentation. Primary outcomes were ICU admission and death. Logistic regression was used to identify independent clinical variables predicting the two outcomes. The model was validated by splitting the data into 70% for training and 30% for testing. Performance accuracy was evaluated using area under the curve (AUC) of the receiver operating characteristic analysis (ROC). Five significant variables predicting ICU admission were lactate dehydrogenase, procalcitonin, pulse oxygen saturation, smoking history, and lymphocyte count. Seven significant variables predicting mortality were heart failure, procalcitonin, lactate dehydrogenase, chronic obstructive pulmonary disease, pulse oxygen saturation, heart rate, and age. The mortality group uniquely contained cardiopulmonary variables. The risk score model yielded good accuracy with an AUC of 0.74 ([95% CI, 0.63-0.85], p = 0.001) for predicting ICU admission and 0.83 ([95% CI, 0.73-0.92], p<0.001) for predicting mortality for the testing dataset. This study identified key independent clinical variables that predicted ICU admission and mortality associated with COVID-19. This risk score system may prove useful for frontline physicians in clinical decision-making under time-sensitive and resource-constrained environment.
MoreTranslated text
PDF
View via Publisher
AI Read Science
AI Summary
AI Summary is the key point extracted automatically understanding the full text of the paper, including the background, methods, results, conclusions, icons and other key content, so that you can get the outline of the paper at a glance.
Example
Background
Key content
Introduction
Methods
Results
Related work
Fund
Key content
- Pretraining has recently greatly promoted the development of natural language processing (NLP)
- We show that M6 outperforms the baselines in multimodal downstream tasks, and the large M6 with 10 parameters can reach a better performance
- We propose a method called M6 that is able to process information of multiple modalities and perform both single-modal and cross-modal understanding and generation
- The model is scaled to large model with 10 billion parameters with sophisticated deployment, and the 10 -parameter M6-large is the largest pretrained model in Chinese
- Experimental results show that our proposed M6 outperforms the baseline in a number of downstream tasks concerning both single modality and multiple modalities We will continue the pretraining of extremely large models by increasing data to explore the limit of its performance
Try using models to generate summary,it takes about 60s
Must-Reading Tree
Example
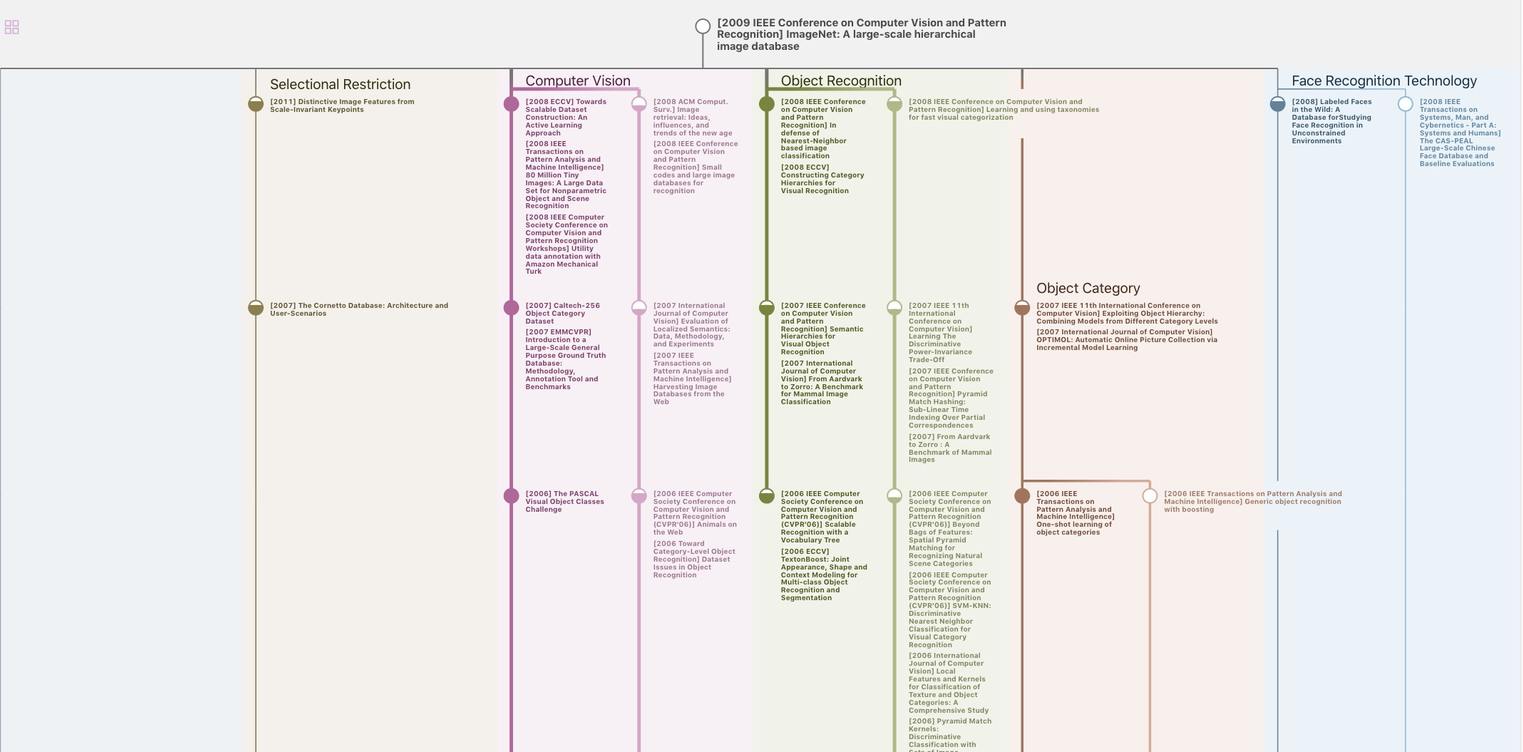
Generate MRT to find the research sequence of this paper
Related Papers
JOURNAL OF THE AMERICAN COLLEGE OF EMERGENCY PHYSICIANS OPEN 2021
被引用6
Japanese Journal of Infectious Diseases 2021
被引用15
Longitudinal Prediction of Hospital-Acquired Acute Kidney Injury in COVID-19: a Two-Center Study
Infection 2021
被引用28
Revista Clínica Española (English Edition) 2021
被引用6
Hepatology International 2021
被引用16
Thiol Level and Total Oxidant/antioxidant Status in Patients with COVID-19 Infection.
Irish Journal of Medical Science 2021
被引用21
Machine Learning Techniques to Predict Different Levels of Hospital Care of CoVid-19
Applied Intelligence 2021
被引用11
BMC Infectious Diseases 2022
被引用7
Journal of Epidemiology and Global Health 2021
被引用48
medRxiv 2021
被引用1
Comparing Machine Learning Algorithms for Predicting COVID-19 Mortality
BMC Medical Informatics and Decision Making 2022
被引用74
Internal and Emergency Medicine 2022
被引用6
Prognosis Patients with COVID-19 Using Deep Learning
BMC Medical Informatics and Decision Making 2022
被引用4
Scientific Reports 2022
被引用8
BioMed Research International 2022
被引用13
Multiple Sclerosis and Related Disorders 2022
被引用4
Journal of Healthcare Engineering 2022
被引用7
BMC Bioinformatics 2022
被引用7
Computational Intelligence and Neuroscience 2022
被引用24
INTERNATIONAL JOURNAL OF INFECTIOUS DISEASES 2022
被引用9
BMC Health Services Research 2022
被引用6
The American Journal of Cardiology 2022
被引用3
Proceedings of the ACM on Interactive, Mobile, Wearable and Ubiquitous Technologies 2022
被引用1
Journal of biomedical physics & engineering 2022
被引用3
JOURNAL OF PERSONALIZED MEDICINE 2023
被引用1
2022 International Conference on Green Energy, Computing and Sustainable Technology (GECOST) 2022
被引用0
Internal and Emergency Medicine 2023
被引用4
Travel Behavior Adjustment Based Epidemic Spreading Model and Prediction for COVID-19
ACTA PHYSICA SINICA 2023
被引用1
Trends in Medical Sciences 2023
被引用0
Data Disclaimer
The page data are from open Internet sources, cooperative publishers and automatic analysis results through AI technology. We do not make any commitments and guarantees for the validity, accuracy, correctness, reliability, completeness and timeliness of the page data. If you have any questions, please contact us by email: report@aminer.cn
Chat Paper
去 AI 文献库 对话