MUST-Plus: A Machine Learning Classifier That Improves Malnutrition Screening in Acute Care Facilities
JOURNAL OF THE AMERICAN COLLEGE OF NUTRITION(2020)
摘要
Objective Malnutrition among hospital patients, a frequent, yet under-diagnosed problem is associated with adverse impact on patient outcome and health care costs. Development of highly accurate malnutrition screening tools is, therefore, essential for its timely detection, for providing nutritional care, and for addressing the concerns related to the suboptimal predictive value of the conventional screening tools, such as the Malnutrition Universal Screening Tool (MUST). We aimed to develop a machine learning (ML) based classifier (MUST-Plus) for more accurate prediction of malnutrition. Method A retrospective cohort with inpatient data consisting of anthropometric, lab biochemistry, clinical data, and demographics from adult (>= 18 years) admissions at a large tertiary health care system between January 2017 and July 2018 was used. The registered dietitian (RD) nutritional assessments were used as the gold standard outcome label. The cohort was randomly split (70:30) into training and test sets. A random forest model was trained using 10-fold cross-validation on training set, and its predictive performance on test set was compared to MUST. Results In all, 13.3% of admissions were associated with malnutrition in the test cohort. MUST-Plus provided 73.07% (95% confidence interval [CI]: 69.61%-76.33%) sensitivity, 76.89% (95% CI: 75.64%-78.11%) specificity, and 83.5% (95% CI: 82.0%-85.0%) area under the receiver operating curve (AUC). Compared to classic MUST, MUST-Plus demonstrated 30% higher sensitivity, 6% higher specificity, and 17% increased AUC. Conclusions ML-based MUST-Plus provided superior performance in identifying malnutrition compared to the classic MUST. The tool can be used for improving the operational efficiency of RDs by timely referrals of high-risk patients.
更多查看译文
关键词
Malnutrition universal screening tool,machine learning,random forest,hospital medicine
AI 理解论文
溯源树
样例
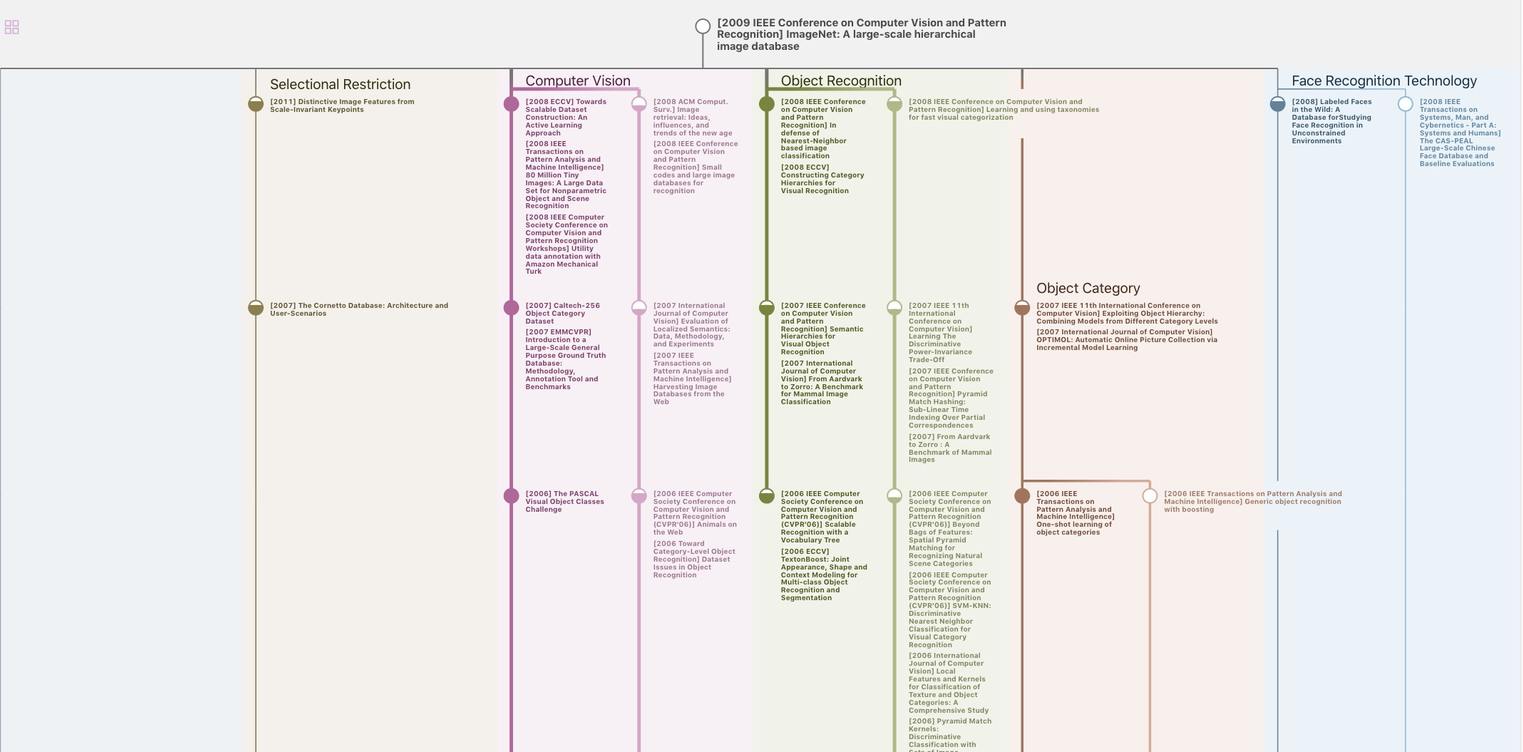
生成溯源树,研究论文发展脉络
Chat Paper
正在生成论文摘要