17Q21.31 Sub-Haplotypes Underlying H1-associated Risk for Parkinson’s Disease and Progressive Supranuclear Palsy Converge on Altered Glial Regulation
semanticscholar(2019)
Ronald M. Loeb Center for Alzheimer's disease | Department of Pathology | University of California Institute for Memory Impairments and Neurological Disorders | Pamela Sklar Division of Psychiatric Genomics Icahn Institute for Data Science and Genomic Technology | National Institute on Aging
Abstract
KR Bowles, DA Pugh, K Farrell, N Han, J TCW, Y Liu, SA Liang, L Qian, J Bendl, JF Fullard, AE Renton, A Casella, MA Iida, S Bandres-Ciga, Z Gan-Or, P Heutink, A Siitonen, S Bertelsen, CM Karch, SJ Frucht, BH Kopell , I Peter, YJ Park, PK Crane, JSK Kauwe, KL Boehme, GU Höglinger, PART working group, International Parkinson’s Disease Genomics Consortium (IPDGC), Progressive Supranuclear Palsy Genetics Consortium, A Charney , P Roussos, JC Wang, WW Poon, T Raj, JF Crary & AM Goate
MoreTranslated text
PDF
View via Publisher
AI Read Science
AI Summary
AI Summary is the key point extracted automatically understanding the full text of the paper, including the background, methods, results, conclusions, icons and other key content, so that you can get the outline of the paper at a glance.
Example
Background
Key content
Introduction
Methods
Results
Related work
Fund
Key content
- Pretraining has recently greatly promoted the development of natural language processing (NLP)
- We show that M6 outperforms the baselines in multimodal downstream tasks, and the large M6 with 10 parameters can reach a better performance
- We propose a method called M6 that is able to process information of multiple modalities and perform both single-modal and cross-modal understanding and generation
- The model is scaled to large model with 10 billion parameters with sophisticated deployment, and the 10 -parameter M6-large is the largest pretrained model in Chinese
- Experimental results show that our proposed M6 outperforms the baseline in a number of downstream tasks concerning both single modality and multiple modalities We will continue the pretraining of extremely large models by increasing data to explore the limit of its performance
Try using models to generate summary,it takes about 60s
Must-Reading Tree
Example
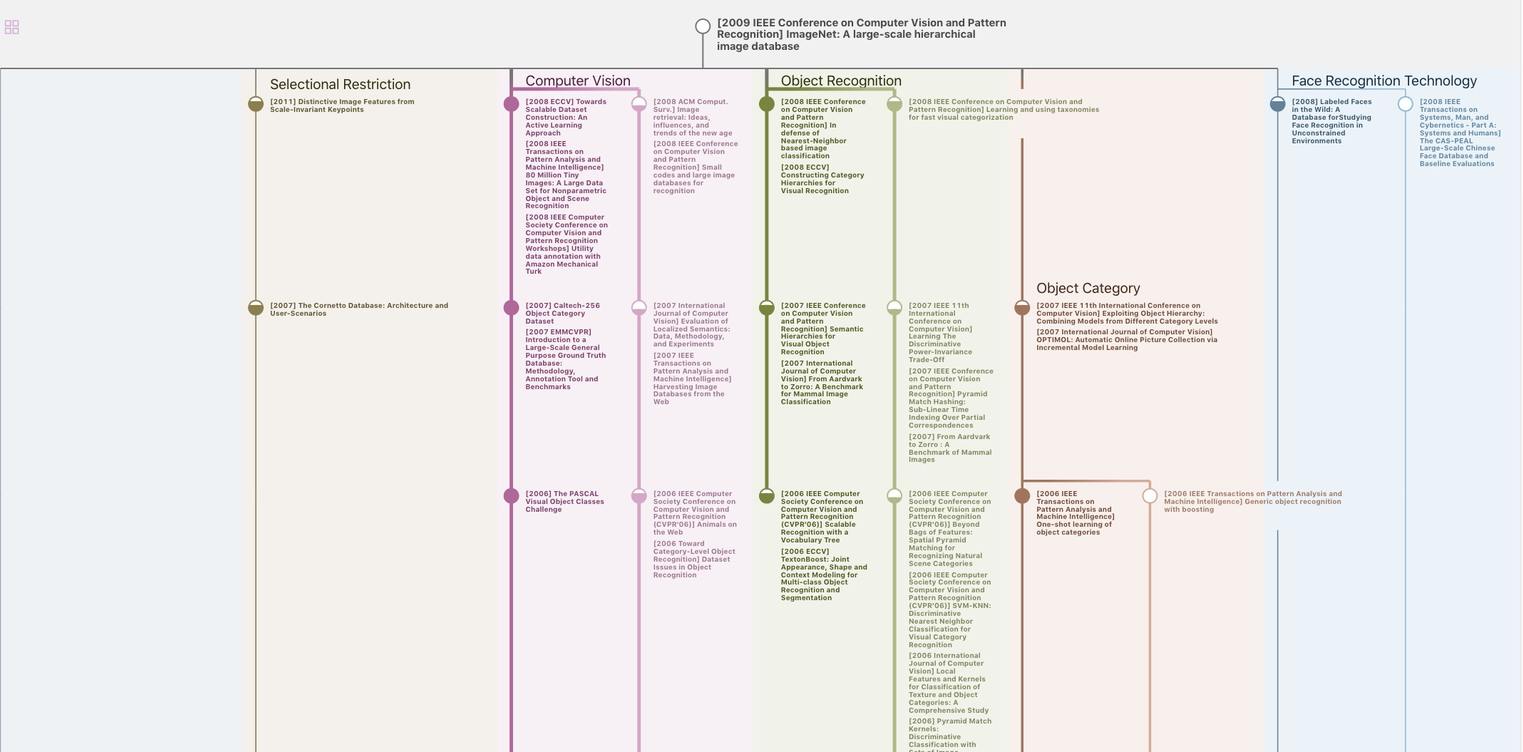
Generate MRT to find the research sequence of this paper
Related Papers
Functional Regulatory Variants Implicate Distinct Transcriptional Networks in Dementia
Science 2022
被引用35
Cross-regional Homeostatic and Reactive Glial Signatures in Multiple Sclerosis
Acta Neuropathologica 2022
被引用17
Data Disclaimer
The page data are from open Internet sources, cooperative publishers and automatic analysis results through AI technology. We do not make any commitments and guarantees for the validity, accuracy, correctness, reliability, completeness and timeliness of the page data. If you have any questions, please contact us by email: report@aminer.cn
Chat Paper
去 AI 文献库 对话